QDAP: Downsizing adaptive policy for cooperative multi-agent reinforcement learning
Knowledge-Based Systems(2024)
摘要
Existing multi-agent reinforcement learning methods employ a paradigm of centralized training with decentralized execution (CTDE) to learn cooperative policy among agents via coordination. However, within continuous destruction conditions, the inclusion of information from dead agents significantly undermines the ability to effectively learn cooperative policies in multi-agent systems. In this paper, we first analyze the bias introduced by dead agents under the CTDE paradigm and how it affects cooperation among agents. Following this, we propose q-learning based downsizing adaptive policy (QDAP) framework for cooperative multi-agent reinforcement learning. QDAP actively discerns relevant values from dead agents and utilizes an innovative approach to convert historical trajectories into weighting factors, thereby aiding remaining active agents in learning more appropriate cooperative policies. Moreover, we extend our proposed framework into the CTDE paradigm, facilitating seamless adaptation with the methods of value decomposition. Experimental results demonstrate that QDAP significantly improves learning speed and achieves superior cooperation performance on challenging Starcraft II micromanagement benchmark tasks.
更多查看译文
关键词
Reinforcement learning,Multi-agent reinforcement learning,Centralized Training with Decentralized Execution,Multi-agent credit assignment
AI 理解论文
溯源树
样例
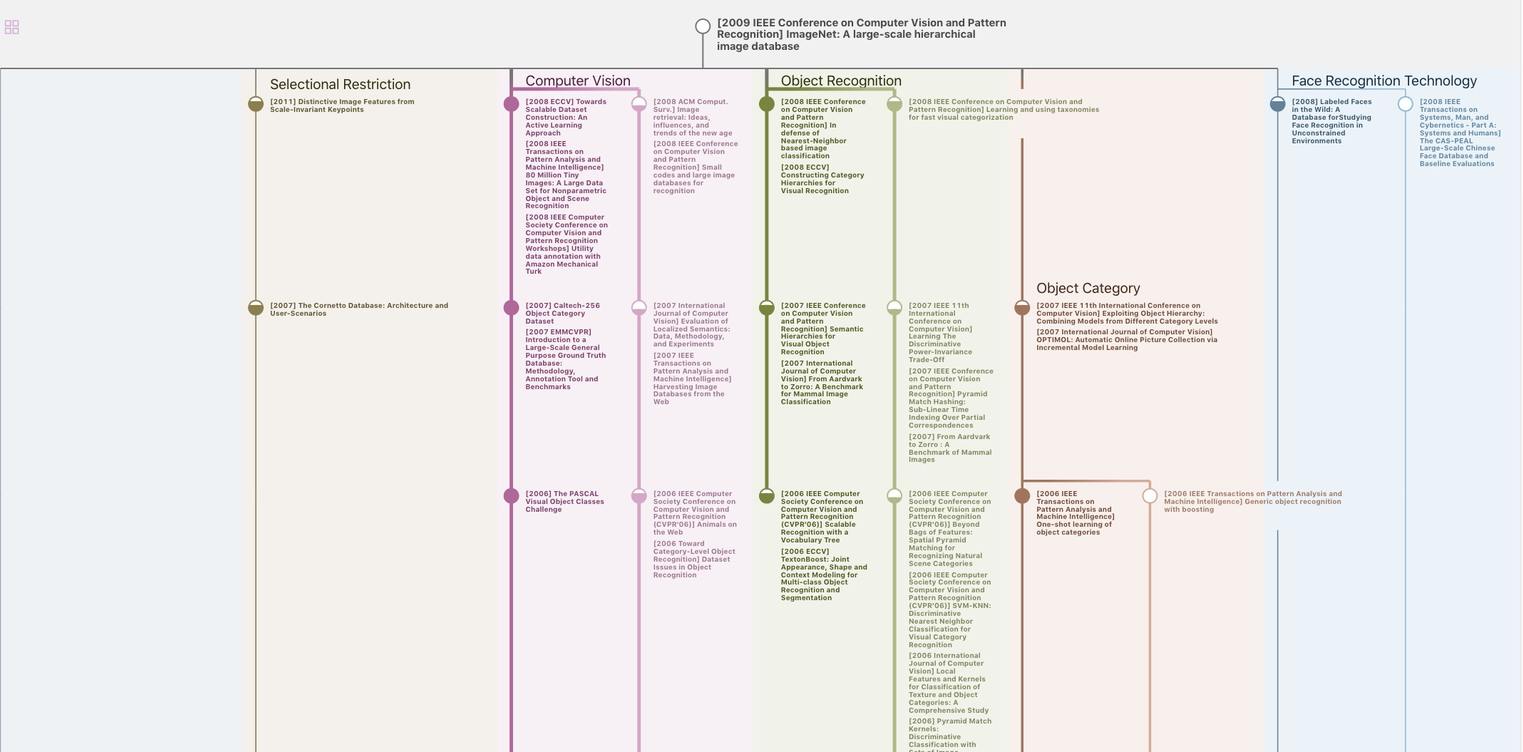
生成溯源树,研究论文发展脉络
Chat Paper
正在生成论文摘要