Enhancing Peak Runoff Forecasting through Feature Engineering Applied to X-Band Radar Data
WATER(2024)
摘要
Floods cause significant damage to human life, infrastructure, agriculture, and the economy. Predicting peak runoffs is crucial for hazard assessment, but it is challenging in remote areas like the Andes due to limited hydrometeorological data. We utilized a 300 km2 catchment over the period 2015-2021 to develop runoff forecasting models exploiting precipitation information retrieved from an X-band weather radar. For the modeling task, we employed the Random Forest (RF) algorithm in combination with a Feature Engineering (FE) strategy applied to the radar data. The FE strategy is based on an object-based approach, which derives precipitation characteristics from radar data. These characteristics served as inputs for the models, distinguishing them as "enhanced models" compared to "referential models" that incorporate precipitation estimates from all available pixels (1210) for each hour. From 29 identified events, enhanced models achieved Nash-Sutcliffe efficiency (NSE) values ranging from 0.94 to 0.50 for lead times between 1 and 6 h. A comparative analysis between the enhanced and referential models revealed a remarkable 23% increase in NSE-values at the 3 h lead time, which marks the peak improvement. The enhanced models integrated new data into the RF models, resulting in a more accurate representation of precipitation and its temporal transformation into runoff.
更多查看译文
关键词
Peak runoff forecast,X-band radar,Random Forest,Andes
AI 理解论文
溯源树
样例
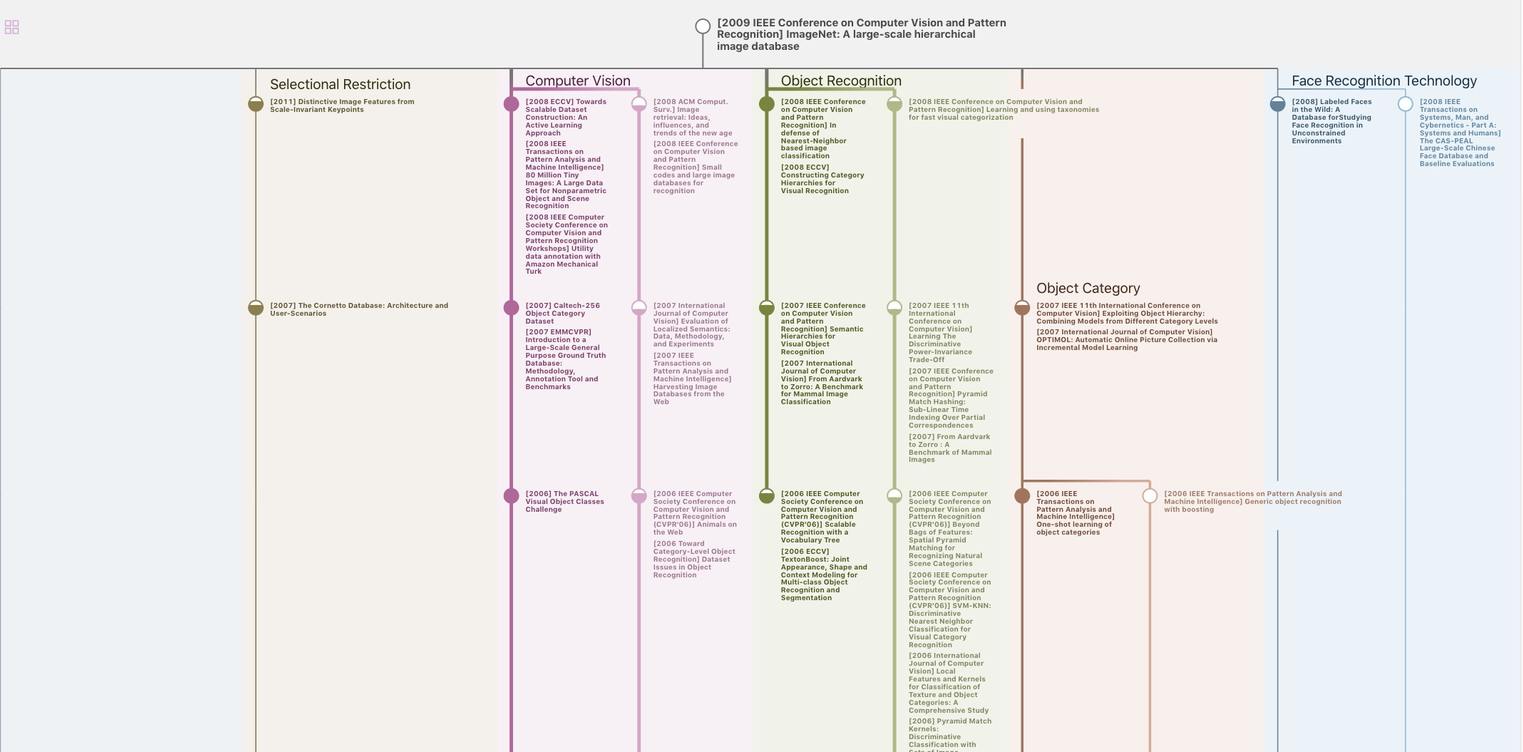
生成溯源树,研究论文发展脉络
Chat Paper
正在生成论文摘要