Can cross-domain term extraction benefit from cross-lingual transfer and nested term labeling?
Machine Learning(2024)
摘要
Automatic term extraction (ATE) is a natural language processing task that eases the effort of manually identifying terms from domain-specific corpora by providing a list of candidate terms. In this paper, we treat ATE as a sequence-labeling task and explore the efficacy of XLMR in evaluating cross-lingual and multilingual learning against monolingual learning in the cross-domain ATE context. Additionally, we introduce NOBI, a novel annotation mechanism enabling the labeling of single-word nested terms. Our experiments are conducted on the ACTER corpus, encompassing four domains and three languages (English, French, and Dutch), as well as the RSDO5 Slovenian corpus, encompassing four additional domains. Results indicate that cross-lingual and multilingual models outperform monolingual settings, showcasing improved F1-scores for all languages within the ACTER dataset. When incorporating an additional Slovenian corpus into the training set, the multilingual model exhibits superior performance compared to state-of-the-art approaches in specific scenarios. Moreover, the newly introduced NOBI labeling mechanism enhances the classifier’s capacity to extract short nested terms significantly, leading to substantial improvements in Recall for the ACTER dataset and consequentially boosting the overall F1-score performance.
更多查看译文
关键词
Term extraction,XLMR,Sequence labeling,Cross-lingual,Cross-domain,Nested terms
AI 理解论文
溯源树
样例
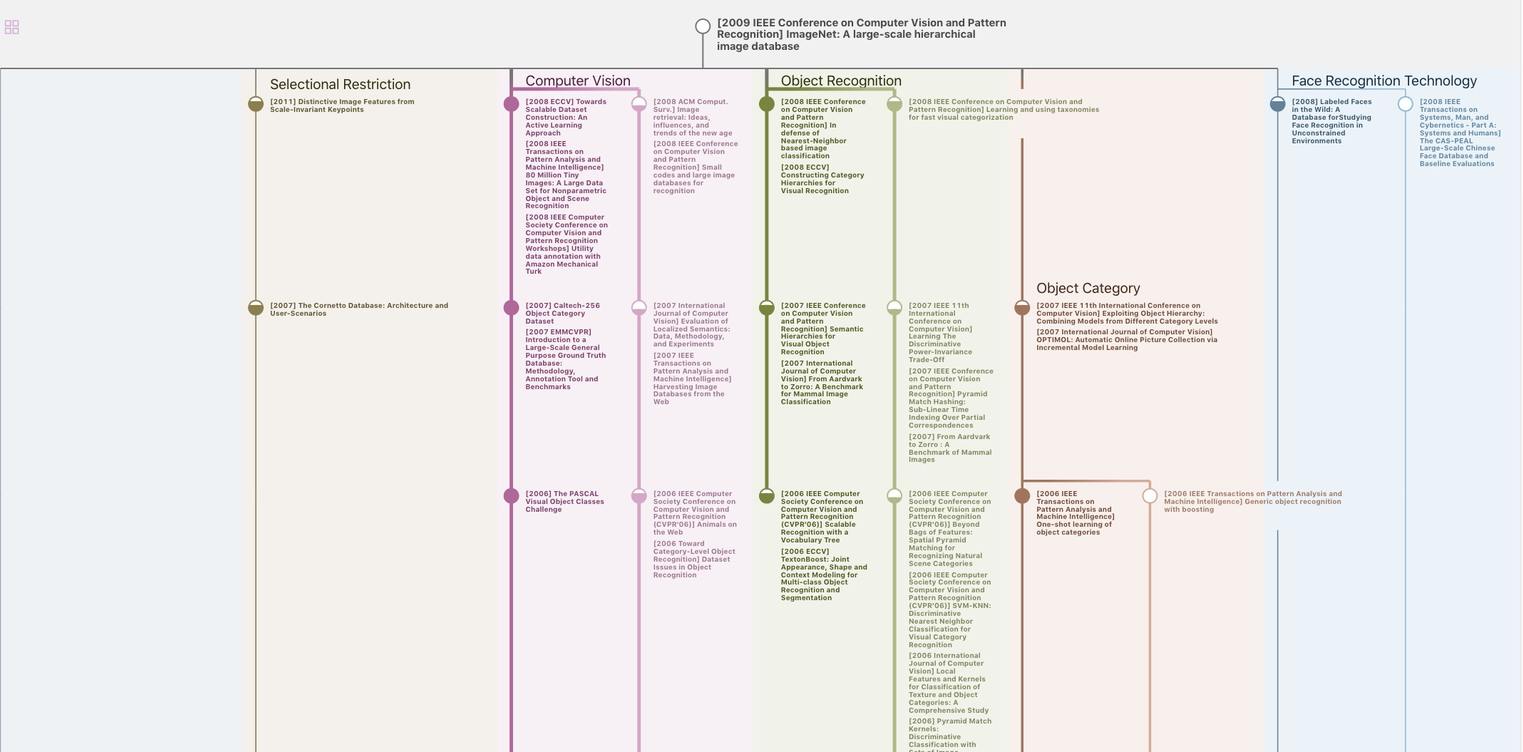
生成溯源树,研究论文发展脉络
Chat Paper
正在生成论文摘要