Evaluating deep learning methods applied to Landsat time series subsequences to detect and classify boreal forest disturbances events: The challenge of partial and progressive disturbances
Remote Sensing of Environment(2024)
摘要
The monitoring of forest ecosystems is significantly affected by the lack of consistent historical data of low-severity (forest partially disturbed) or gradual disturbance (e.g. eastern spruce budworm epidemic). The goal of this paper is to explore the use of a subset of Landsat time series and deep learning models to identify both the type and the year of disturbances, including low-severity and gradual disturbances, in the boreal forest of eastern Canada at the pixel level. Remote sensing data such as the spectral information from Landsat time series are the best available option for large scale observations of disturbances that go back decades. Traditional modeling approaches, like LandTrendr, require substantial handcrafted pre-processing to remove noise and to extract temporal features from the image sequences before using them as input to a classical machine-learning model. Deep-learning models can autonomously discern which features are relevant within the coarse temporal and spectral information from the Landsat annual dense time series.We evaluated the performance of TempCNN and Transformer model in detecting and classifying the type and the year of the forest disturbance using Landsat time series subsequences. Our findings resulted in the generation of four disturbance maps outlining the forest history from 1986 to 2021 within the eastern Canadian boreal forest. Our experimental outcomes demonstrate several significant benefits of employing deep learning models. Firstly, using noisy Landsat time series they achieve comparable accuracy for classifying fire and total harvesting than existing publicly available disturbance maps. Secondly, the use of shorter time series subsequence with deep learning models enables to map adequately different overlapping disturbances occurring in the complete time series. Finally, they increase the number of distinguishable disturbance classes by adding partial harvesting, gradual disturbances, and forest recovery from older events, making them useful approaches for obtaining the first remote sensing-based map for areas affected by the eastern spruce budworm.
更多查看译文
关键词
Remote sensing,Forest disturbances,Boreal forest,Deep learning,Landsat time series,Transformer,Time series subsequence
AI 理解论文
溯源树
样例
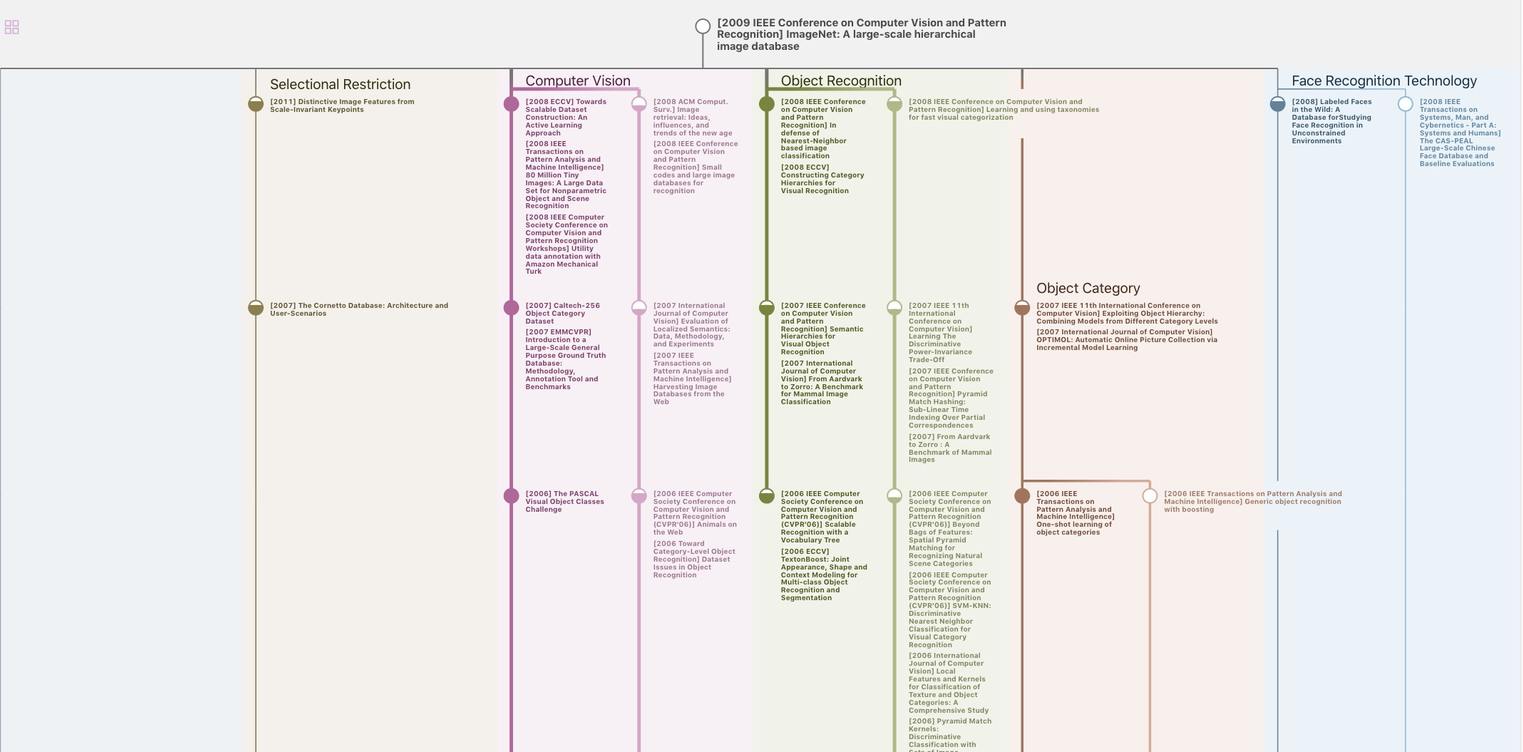
生成溯源树,研究论文发展脉络
Chat Paper
正在生成论文摘要