Improving Reliability of Heterogeneous Change Detection by Sample Synthesis and Knowledge Transfer
IEEE TRANSACTIONS ON GEOSCIENCE AND REMOTE SENSING(2024)
摘要
Detecting changes in heterogeneous images without the supervision of changed label is a challenging yet critical task for quick responding natural disaster relief. Nevertheless, most of available unsupervised heterogeneous change detection (CD) methods strong rely on the quality of pseudo-labels, and they suffer from performance degradation, even irreversible model collapse, when encounter the low-quality pseudo-labels, leading to unreliable detection results. In order to improve the reliability of unsupervised heterogeneous CD, in this article, we propose a novel CD paradigm based on sample synthesis and knowledge transfer. We address the issue of label reliability by artificially creating a changed region and assigning labels rather than constructing pseudo-labels. These constructed labels guide the network in automatically learning the correspondence between heterogeneous images, confirming the reliability of changed regions. Moreover, an augmentation with synthetic samples on real samples makes it possible to generate more transferable samples while reducing the domain gap coarsely. A dual-branch joint training with feature contrastive learning is further developed to transfer the knowledge of changes from the synthetic sample domain to real sample domain. Experimental results on five public datasets demonstrate that our proposed method has superior performance when compared with available state-of-the-art (SOTA) methods. Our code is available at https://github.com/zhangqiiii/SS-KT.
更多查看译文
关键词
Training,Feature extraction,Reliability,Task analysis,Remote sensing,Knowledge transfer,Buildings,Change detection (CD),heterogeneous,knowledge transfer,sample synthesis
AI 理解论文
溯源树
样例
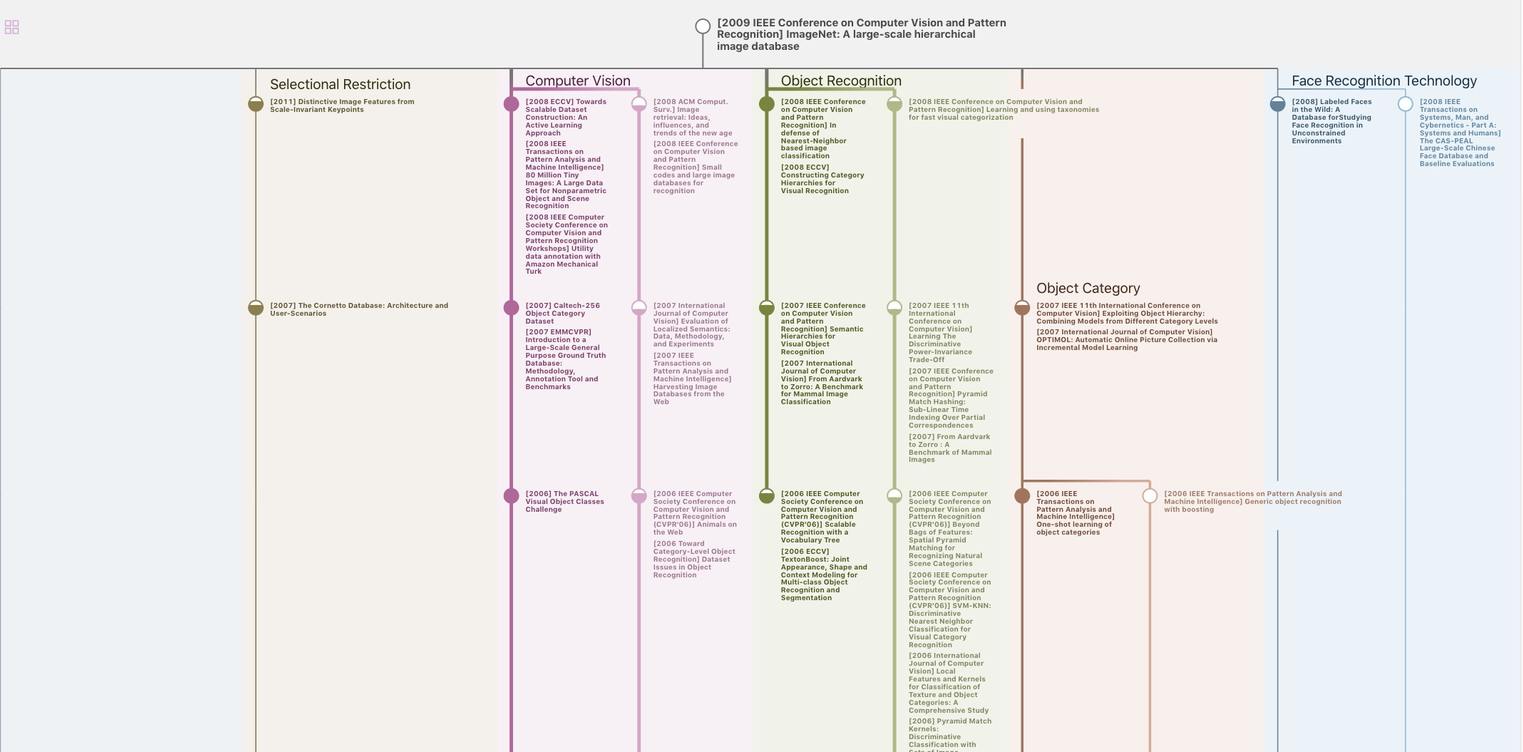
生成溯源树,研究论文发展脉络
Chat Paper
正在生成论文摘要