rpcPRF: Generalizable MPI Neural Radiance Field for Satellite Camera With Single and Sparse Views.
IEEE Trans. Geosci. Remote. Sens.(2024)
摘要
Most advances in neural radiance fields (NeRF) assume sufficient input views from pinhole cameras. This paper proposes rpcPRF, a Multiplane Images (MPI) based Planar neural Radiance Field for Rational Polynomial Camera (RPC), exhibits robust performance even with single or sparse inputs. Unlike coordinate-based NeRFs that require sufficient views of one scene, our model can be applied to new scenes and has shown positive results for single or sparse test images. This allows for generalization across various scenes. To achieve generalization across scenes, we use reprojection supervision to ensure that the predicted MPI (multiplane image) accurately captures the geometry between the 3D coordinates and the images. Additionally, we have obviated the requisite for dense depth supervision in multiview-stereo-based methods by introducing rendering techniques of radiance fields. rpcPRF combines the superiority of implicit representations and the advantages of the RPC model, to capture the continuous altitude space while learning the 3D structure. On the DFC2019 dataset with sparse input of the same scene, rpcPRF achieves the best results. On the TLC dataset and the SatMVS3D dataset with changing scenes in every batch, rpcPRF outperforms state-of-the-art NeRF-based methods by a significant margin in terms of image fidelity, reconstruction accuracy, and efficiency, for both single-view and multiview task.
更多查看译文
关键词
novel view synthesis,neural radiance field,multi-plane image,rational polynomial camera
AI 理解论文
溯源树
样例
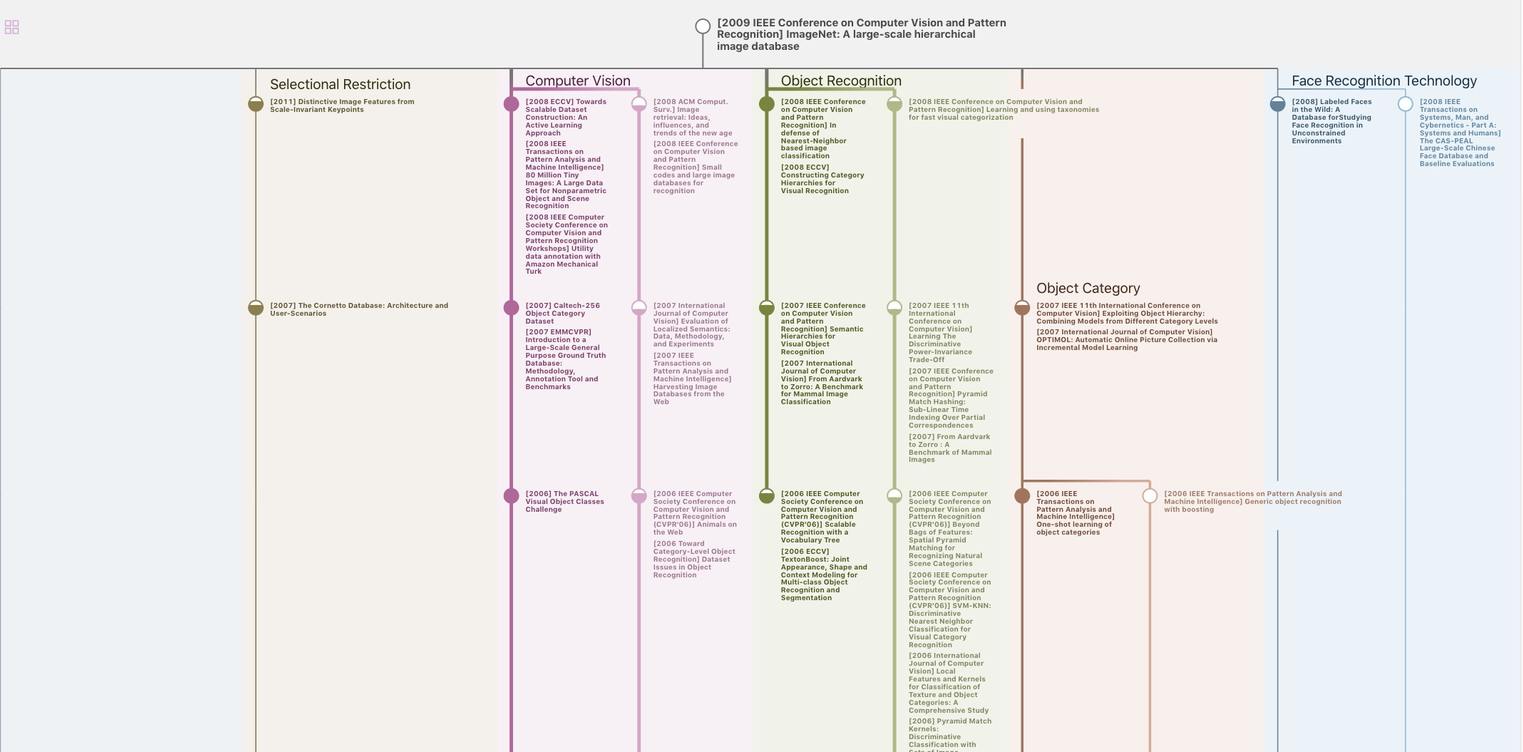
生成溯源树,研究论文发展脉络
Chat Paper
正在生成论文摘要