Classification of Highly Imbalanced Supervisory Control and Data Acquisition Data for Fault Detection of Wind Turbine Generators
Energies(2024)
摘要
It is common knowledge that wind energy is a crucial, strategic component of the mix needed to create a green economy. In this regard, optimizing the operations and maintenance (O&M) of wind turbines (WTs) is key, as it will serve to reduce the levelized cost of electricity (LCOE) of wind energy. Since most modern WTs are equipped with a Supervisory Control and Data Acquisition (SCADA) system for remote monitoring and control, condition-based maintenance using SCADA data is considered a promising solution, although certain drawbacks still exist. Typically, large amounts of normal-operating SCADA data are generated against small amounts of fault-related data. In this study, we use high-frequency SCADA data from an operating WT with a significant imbalance between normal and fault classes. We implement several resampling techniques to address this challenge and generate synthetic generator fault data. In addition, several machine learning (ML) algorithms are proposed for processing the resampled data and WT generator fault classification. Experimental results show that ADASYN + Random Forest obtained the best performance, providing promising results toward wind farm O&M optimization.
更多查看译文
关键词
class imbalance,fault prediction,oversampling technique,SCADA data,wind turbine
AI 理解论文
溯源树
样例
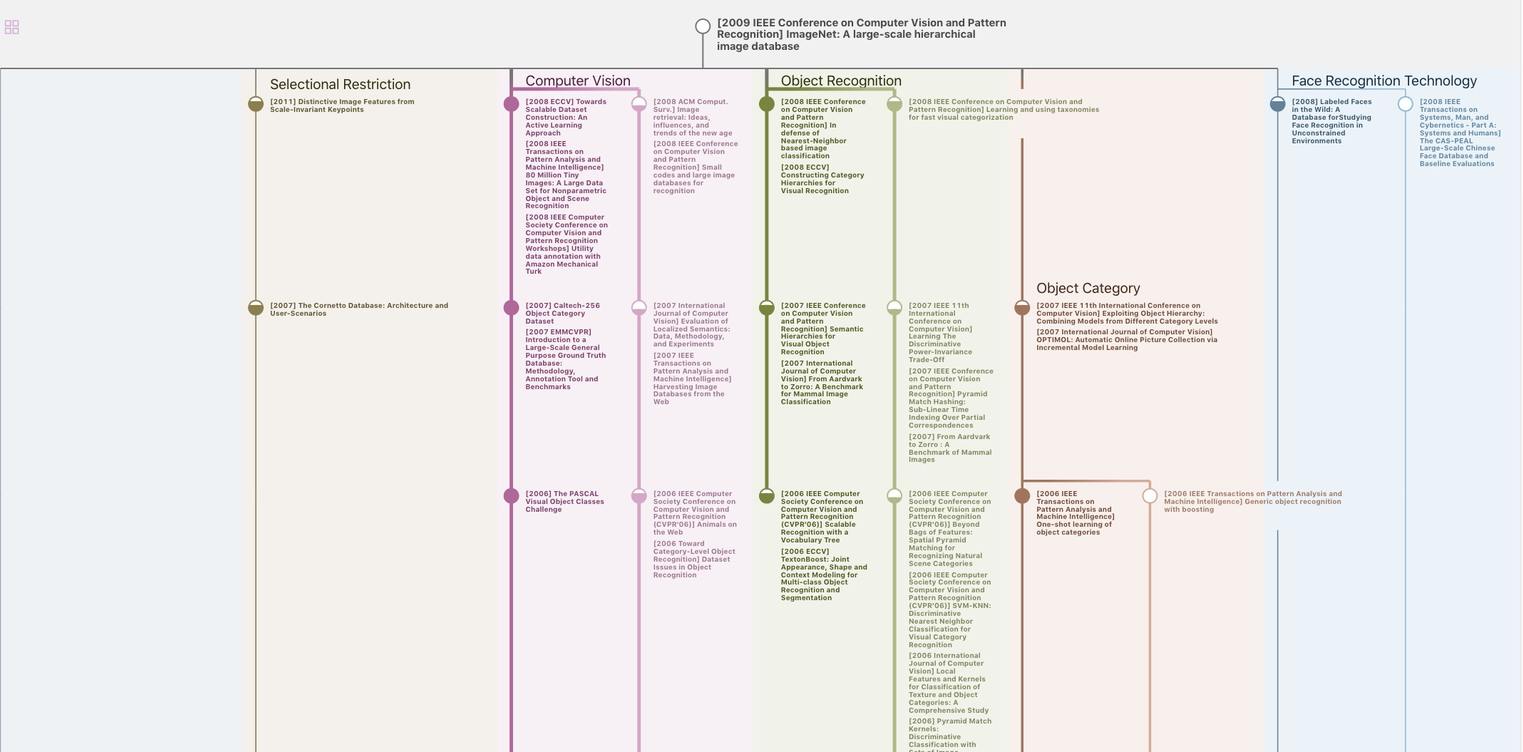
生成溯源树,研究论文发展脉络
Chat Paper
正在生成论文摘要