Recognizing Semantics-Consistent Subsequences for Sequential Recommendation.
International Conference on Parallel and Distributed Systems(2023)
摘要
Predicting user behavior based on their interactions poses a great challenge in the realm of sequential recommendation. This challenge primarily stems from the erratic and periodic nature of user behavior. Recent approaches leverage attention mechanisms to capture users’ long- and short-term interests by analyzing their past interactions, and have achieved notable improvements of recommendation performance. However, none of these methods take into account semantic subsequences, which are indicative of a user’s focused activity over a specific period within the sequence. Incorporating semantic subsequences can significantly enhance the attention mechanism’s ability to capture users’ short-term interests.In this paper, we introduce the Semantic Subsequences Recognizer for Sequential Recommendation (SSR4Rec), comprising two distinct modules tailored for modeling long-term and short-term interests, respectively. For short-term interest modeling, we present a novel LSTM network that intelligently identifies semantic subsequences within sequences. It then extracts semantically coherent subsequences to create a representation of short-term interests. Meanwhile, for long-term interest modeling, we employ a modified BERT model to generate long-term interest representations. Our extensive experiments on three benchmark datasets demonstrates that SSR4Rec outperforms other state-of-the-art sequential models such as Locker and STOSA.
更多查看译文
AI 理解论文
溯源树
样例
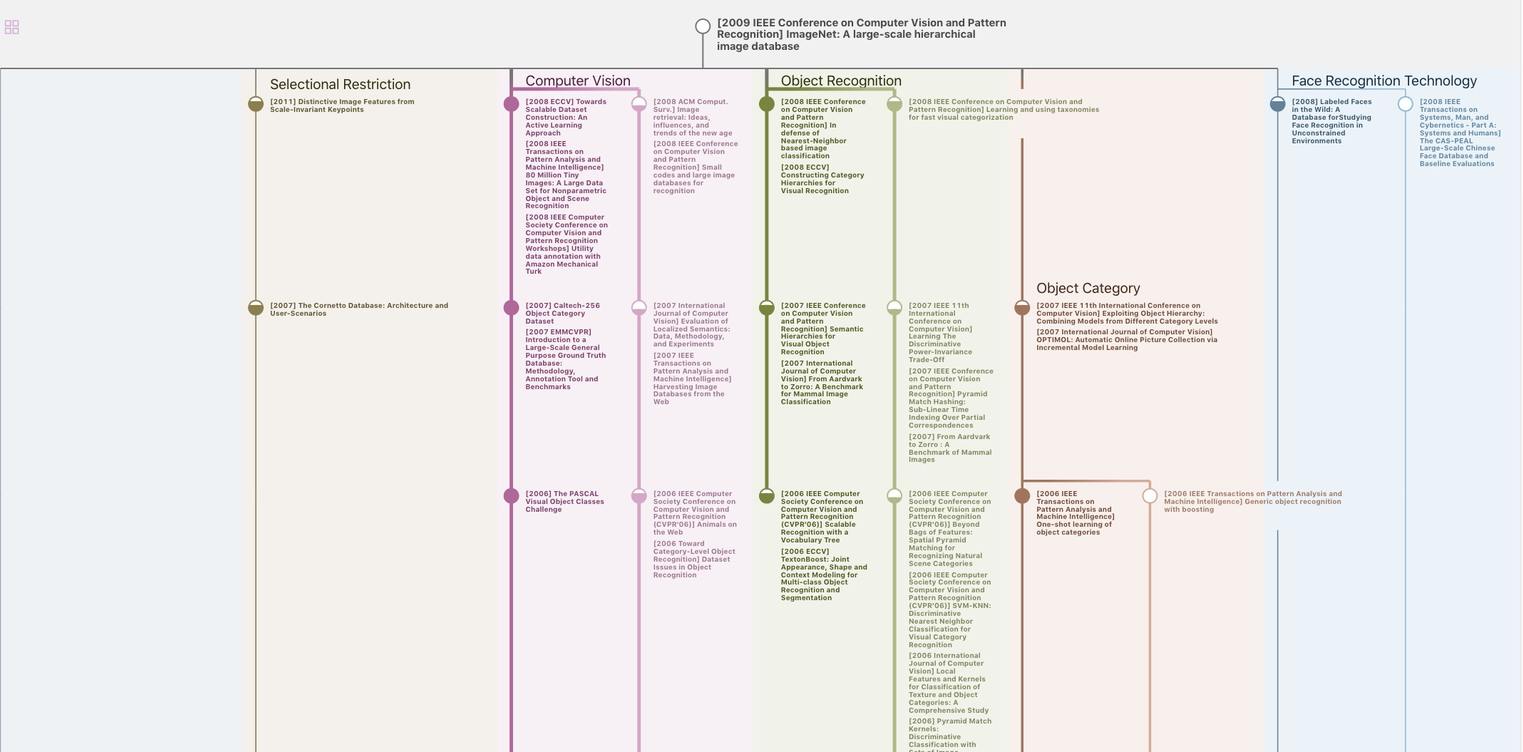
生成溯源树,研究论文发展脉络
Chat Paper
正在生成论文摘要