Dual Contrastive Learning for Multi-Behavior Recommendation.
International Conference on Parallel and Distributed Systems(2023)
摘要
Most existing collaborative filtering-based recommendation models only use a single type of interactive data. However, in real-world scenarios, there is more than one type of user interaction behavior. Although numerous recommendation models already use multi-behavior to make recommendation, there are still two limitations for them: i) the scarcity of supervised interaction data under the target behavior; ii) unable to obtain high-order collaborative relation due to over-smoothing. In order to solve the above problems, we propose a multi-behavior recommendation model, D ual C ontrastive L earning (DCL), based on contrastive learning. In DCL, a graph neural network is used to learn the local representations of users and items under multiple behaviors, while a hypergraph neural network is used to capture the global item dependency. In particular, we design two contrastive learning strategies to reinforce the quality of representations. Behavior-wise contrastive learning captures the knowledge that can be transferred between different behaviors. As well as item-wise contrastive learning captures the dependencies between item representations from different perspectives. The effectiveness of our method is verified on three real-world datasets, and the recommendation performance of DCL is superior to the various state-of-the-art recommendation models. At the same time, we also verify the robustness of our model for users with sparse interactions.
更多查看译文
AI 理解论文
溯源树
样例
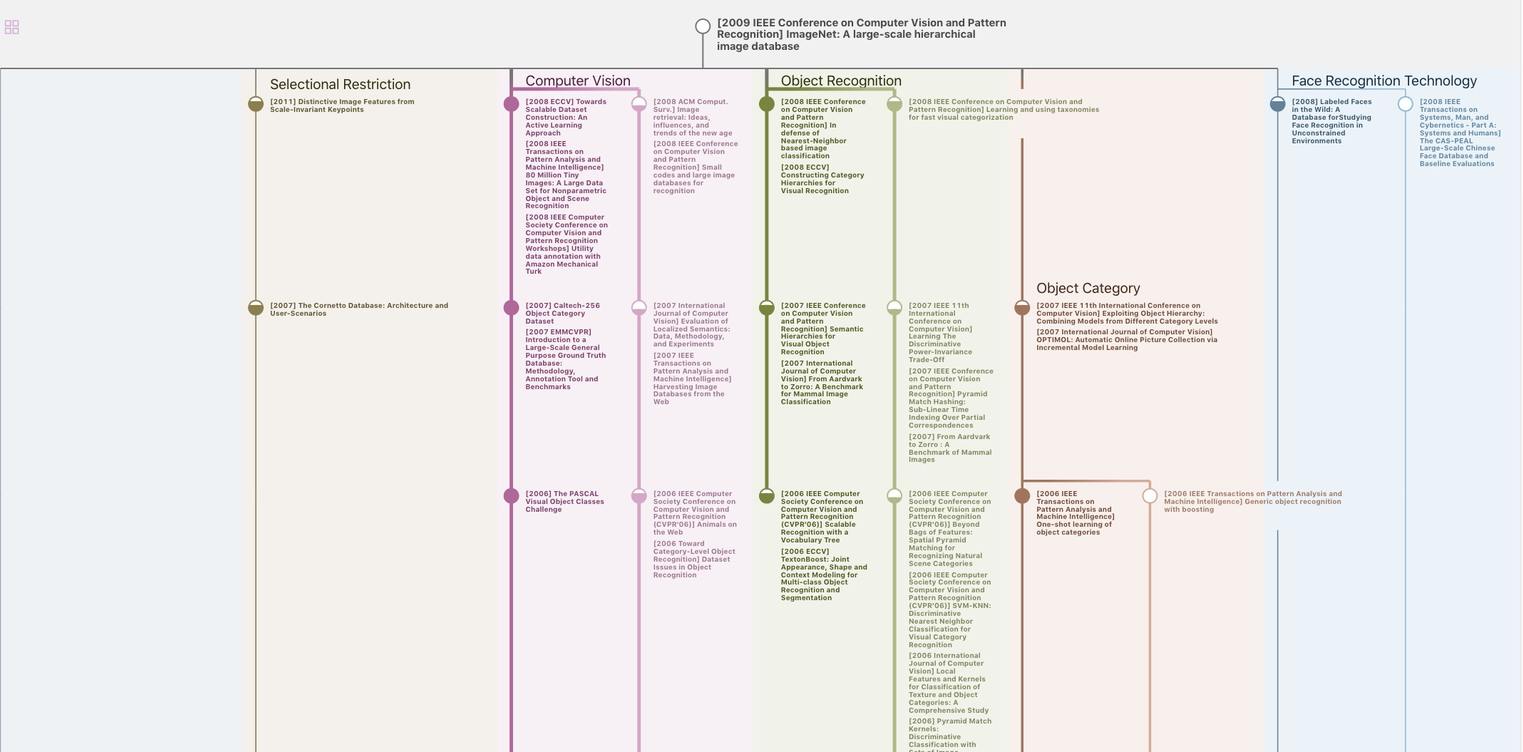
生成溯源树,研究论文发展脉络
Chat Paper
正在生成论文摘要