GraphPath: a Graph Attention Model for Molecular Stratification with Interpretability Based on the Pathway-Pathway Interaction Network
Bioinformatics (Oxford, England)(2024)
摘要
Motivation Studying the molecular heterogeneity of cancer is essential for achieving personalized therapy. At the same time, understanding the biological processes that drive cancer development can lead to the identification of valuable therapeutic targets. Therefore, achieving accurate and interpretable clinical predictions requires paramount attention to thoroughly characterizing patients at both the molecular and biological pathway levels.Results Here, we present GraphPath, a biological knowledge-driven graph neural network with multi-head self-attention mechanism that implements the pathway-pathway interaction network. We train GraphPath to classify the cancer status of patients with prostate cancer based on their multi-omics profiling. Experiment results show that our method outperforms P-NET and other baseline methods. Besides, two external cohorts are used to validate that the model can be generalized to unseen samples with adequate predictive performance. We reduce the dimensionality of latent pathway embeddings and visualize corresponding classes to further demonstrate the optimal performance of the model. Additionally, since GraphPath's predictions are interpretable, we identify target cancer-associated pathways that significantly contribute to the model's predictions. Such a robust and interpretable model has the potential to greatly enhance our understanding of cancer's biological mechanisms and accelerate the development of targeted therapies.Availability and implementation https://github.com/amazingma/GraphPath.
更多查看译文
AI 理解论文
溯源树
样例
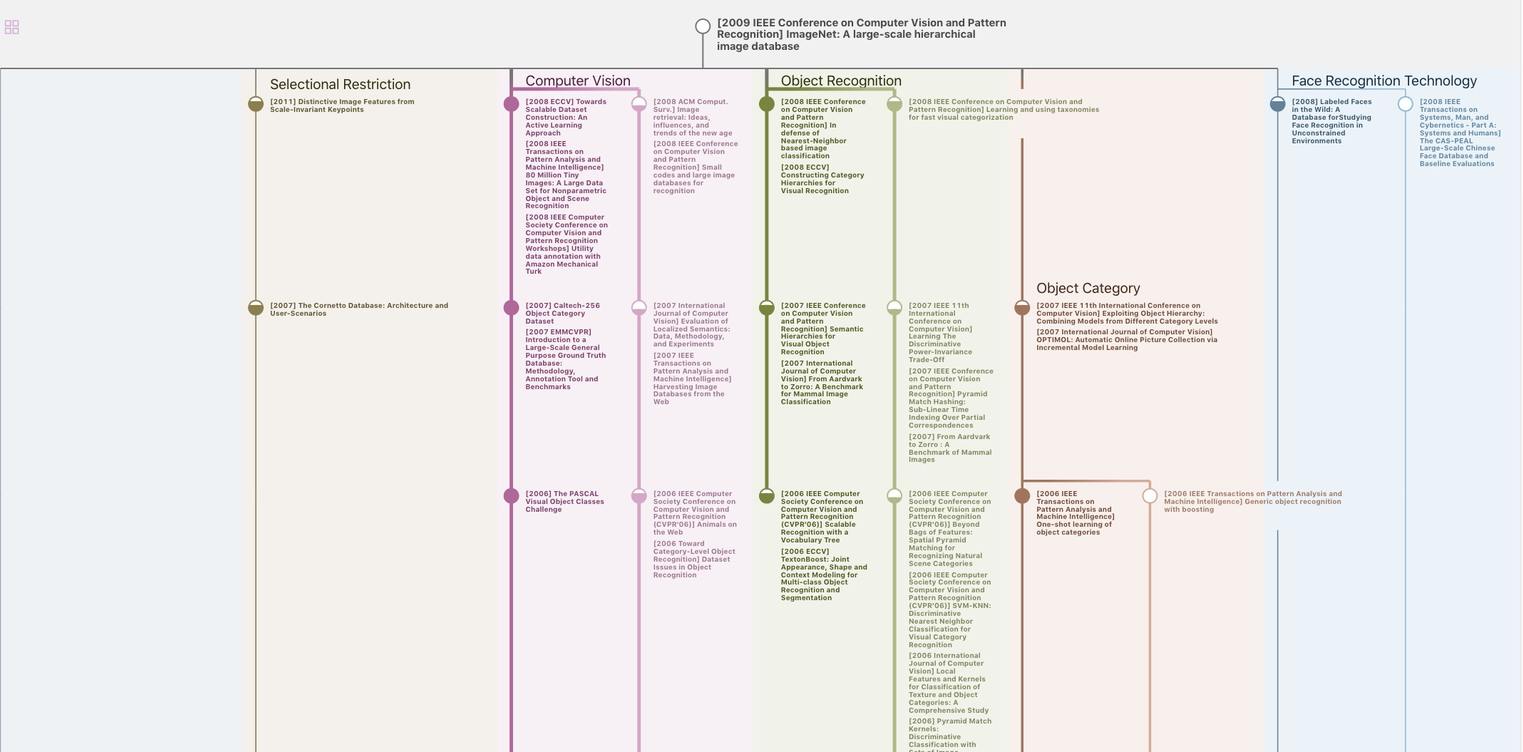
生成溯源树,研究论文发展脉络
Chat Paper
正在生成论文摘要