Rating-Review Graph Contrastive Learning for Review-based Recommendation.
International Conference on Parallel and Distributed Systems(2023)
摘要
Textual reviews have been widely utilized in recommender systems because text reviews contain rich user preference information. Recent studies have increasingly incorporated textual reviews into user-item graphs as auxiliary information to learn node representations, notably enhancing recommendation performance. However, most existing review-based recommendations could not effectively exploit correlations between ratings and reviews, and suffer from noisy interactions which are further amplified during neighborhood aggregation. To address such limitations, we propose a new graph contrastive learning model for review-based recommendations in this paper. We construct a user-item graph view using both ratings and reviews. In addition, we design two graph views with ratings and reviews, respectively. Through contrastive learning based on these three views, our model manages to generate rich supervision signals for both user and item nodes. Our approach effectively explores intrinsic correlations between heterogeneous rating and review data, which enhance the robustness against interaction noises. A comprehensive experimental study on five benchmark datasets demonstrates that our model outperforms state-of-the-art methods.
更多查看译文
关键词
recommender systems,graph neural networks,review-based recommendation,contrastive learning
AI 理解论文
溯源树
样例
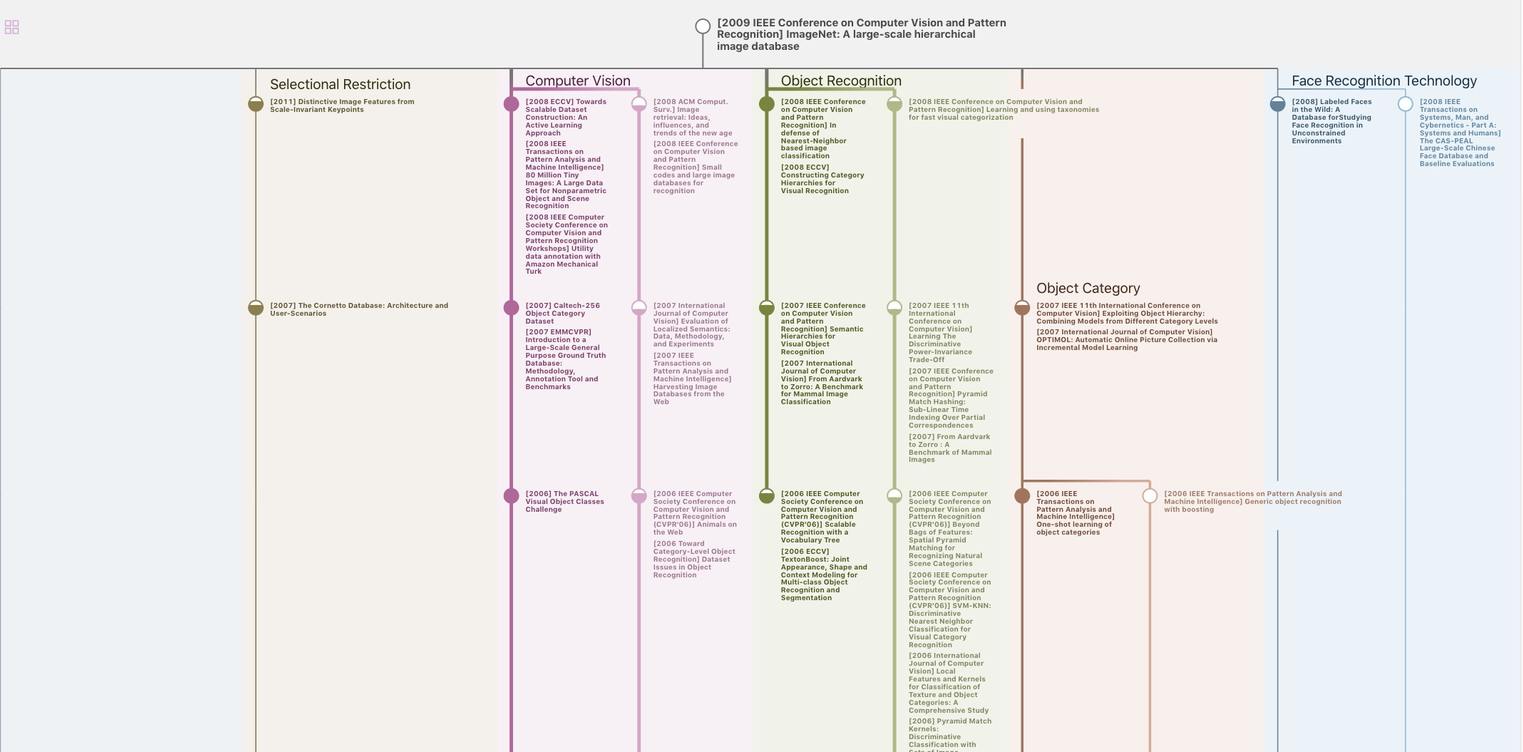
生成溯源树,研究论文发展脉络
Chat Paper
正在生成论文摘要