Explanation-Guided Adversarial Example Attacks
Big data research(2024)
摘要
Neural network-based classifiers are vulnerable to adversarial example attacks even in a black-box setting. Existing adversarial example generation technologies mainly rely on optimization-based attacks, which optimize the objective function by iterative input perturbation. While being able to craft adversarial examples, these techniques require big budgets. Latest transfer-based attacks, though being limited queries, also have a disadvantage of low attack success rate. In this paper, we propose an adversarial example attack method called MEAttack using the model-agnostic explanation technology, which can more efficiently generate adversarial examples in the black-box setting with limited queries. The core idea is to design a novel model-agnostic explanation method for target models, and generate adversarial examples based on model explanations. We experimentally demonstrate that MEAttack outperforms the state-of-the-art attack technology, i.e., AutoZOOM. The success rate of MEAttack is 4.54%-47.42% higher than AutoZOOM, and its query efficiency is reduced by 2.6-4.2 times. Experimental results show that MEAttack is efficient in terms of both attack success rate and query efficiency.
更多查看译文
关键词
Deep neural network,Model explanation,Adversarial examples,Black-box,Label-only
AI 理解论文
溯源树
样例
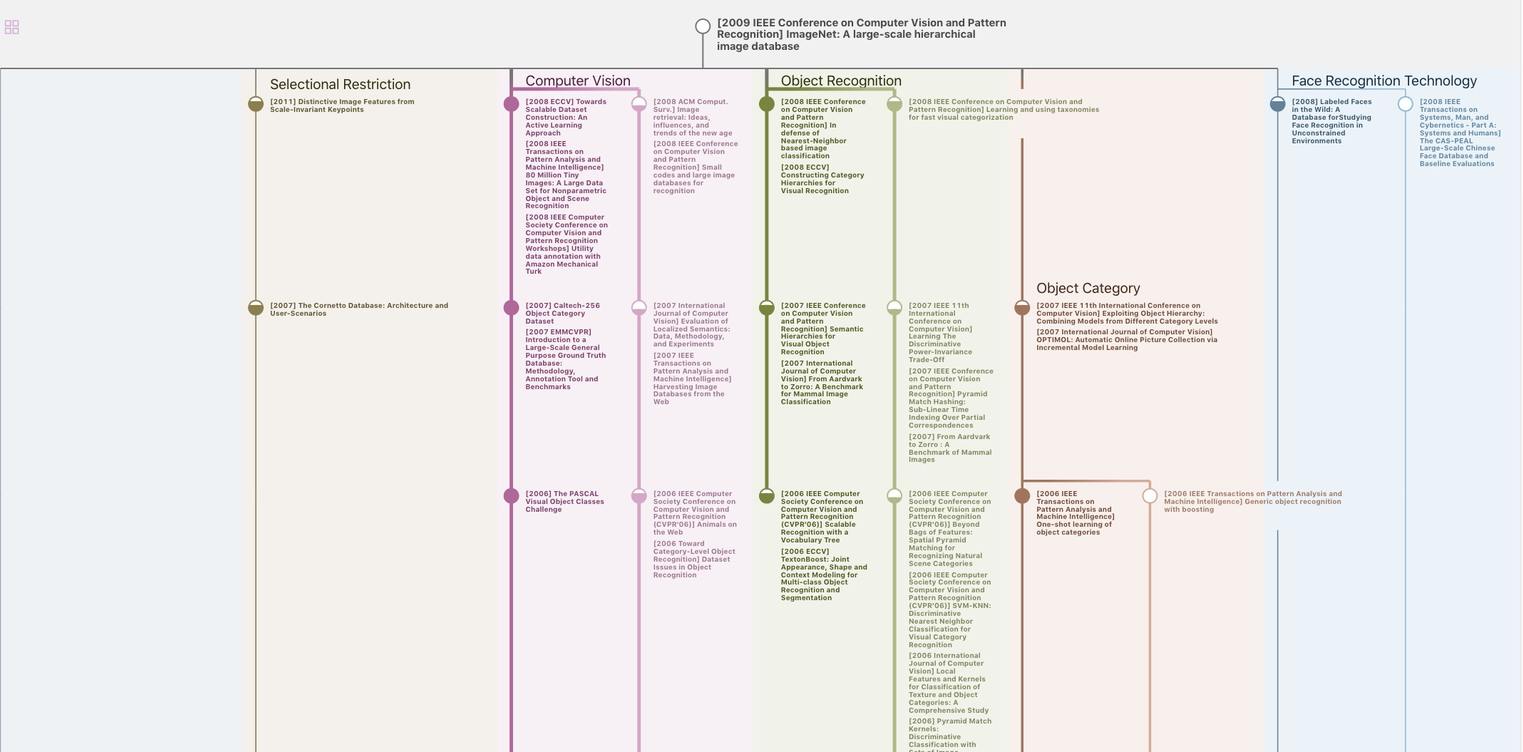
生成溯源树,研究论文发展脉络
Chat Paper
正在生成论文摘要