LSC-2024-Deciphering the Spatial Heterogeneity of Interstitial Lung Disease by Integrative Radiomics and Single-Nucleus Transcriptomics
EUROPEAN RESPIRATORY JOURNAL(2024)
Univ Bern | German Ctr Lung Res DZL | Katholieke Univ Leuven | Univ Zurich | Univ Antwerp
Abstract
Spatial heterogeneity in disease patterns is a key hallmark of interstitial lung diseases (ILDs), such as systemic sclerosis (SSc)-ILD. High-dimensional image analysis (radiomics) of computed tomography (CT) scans offers quantitative insights into organ-scale pathophysiology, creating digital disease fingerprints. Here, we aimed to integrate spatially resolved radiomic profiles extracted from CT scans with matched molecular data to decipher the cellular programs underlying the spatial heterogeneity in ILDs and how they are reflected in radiomic phenotypes. We therefore systematically dissected a whole right lung of a SSc-ILD patient into 65 samples for virtual 3D alignment and association of regional radiomic profiles with CT co-localized histopathology and single-nucleus RNA sequencing (snRNAseq). Radiomic profiles significantly differed with spatial location revealing a typical apical to basal gradient characteristic for the spatial heterogeneity in SSc-ILD. To assess whether those radiomic differences translate to the molecular level, we performed snRNAseq, with 14 distal lung regions from apex to base. Analyzing 124,000 cells from more than 50 cell types we revealed region-specific alterations in cellular composition, gene expression and cell-cell communication networks. Integration of radiomics and transcriptomics data showed spatial radiomic modules with distinct cellular profiles, including an apical T cell exhaustion and a basal macrophage-driven fibrogenic program. In conclusion, we provide an in-depth imaging and molecular phenotyping of the spatial heterogeneity of SSc-ILD, highlighting the potential of radiomics as virtual organ-scale biopsies.
MoreTranslated text
Key words
High-Resolution CT,Medical Imaging,Spectral Imaging,Radiomics,Cancer Imaging
求助PDF
上传PDF
View via Publisher
AI Read Science
AI Summary
AI Summary is the key point extracted automatically understanding the full text of the paper, including the background, methods, results, conclusions, icons and other key content, so that you can get the outline of the paper at a glance.
Example
Background
Key content
Introduction
Methods
Results
Related work
Fund
Key content
- Pretraining has recently greatly promoted the development of natural language processing (NLP)
- We show that M6 outperforms the baselines in multimodal downstream tasks, and the large M6 with 10 parameters can reach a better performance
- We propose a method called M6 that is able to process information of multiple modalities and perform both single-modal and cross-modal understanding and generation
- The model is scaled to large model with 10 billion parameters with sophisticated deployment, and the 10 -parameter M6-large is the largest pretrained model in Chinese
- Experimental results show that our proposed M6 outperforms the baseline in a number of downstream tasks concerning both single modality and multiple modalities We will continue the pretraining of extremely large models by increasing data to explore the limit of its performance
Upload PDF to Generate Summary
Must-Reading Tree
Example
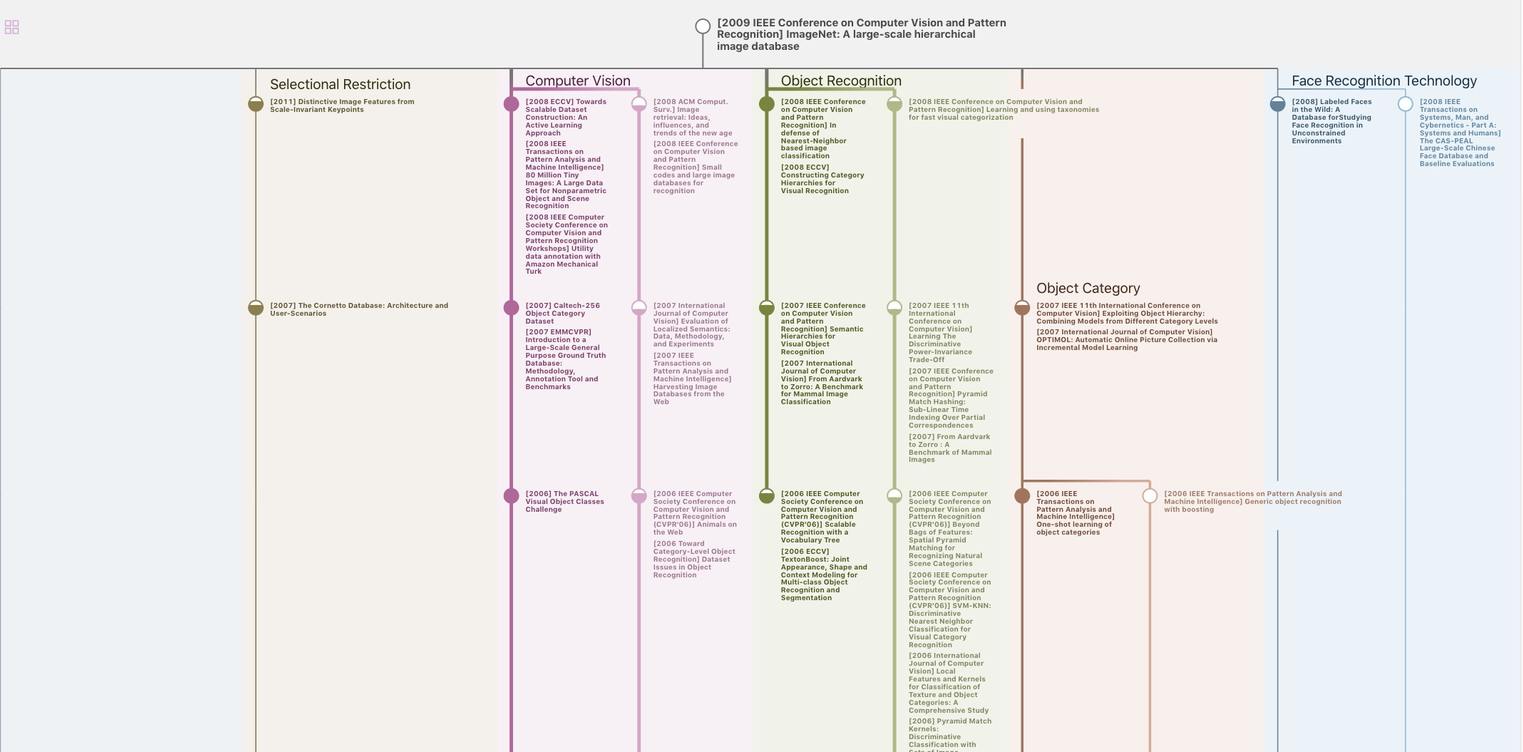
Generate MRT to find the research sequence of this paper
Data Disclaimer
The page data are from open Internet sources, cooperative publishers and automatic analysis results through AI technology. We do not make any commitments and guarantees for the validity, accuracy, correctness, reliability, completeness and timeliness of the page data. If you have any questions, please contact us by email: report@aminer.cn
Chat Paper