Engineering the Neural Collapse Geometry of Supervised-Contrastive Loss (Student Abstract)
AAAI 2024(2024)
摘要
Supervised-contrastive loss (SCL) is an alternative to cross-entropy (CE) for classification tasks that makes use of similarities in the embedding space to allow for richer representations. Previous works have used trainable prototypes to help
improve test accuracy of SCL when training under imbalance. In this work, we propose the use of fixed prototypes to help engineering the feature geometry when training with SCL. We gain further insights by considering a limiting scenario
where the number of prototypes far outnumber the original batch size. Through this, we establish a connection to CE loss with a fixed classifier and normalized embeddings. We validate our findings by conducting a series of experiments with deep neural networks on benchmark vision datasets.
更多查看译文
关键词
Neural Collapse,Feature Geometry,Supervised Contrastive
AI 理解论文
溯源树
样例
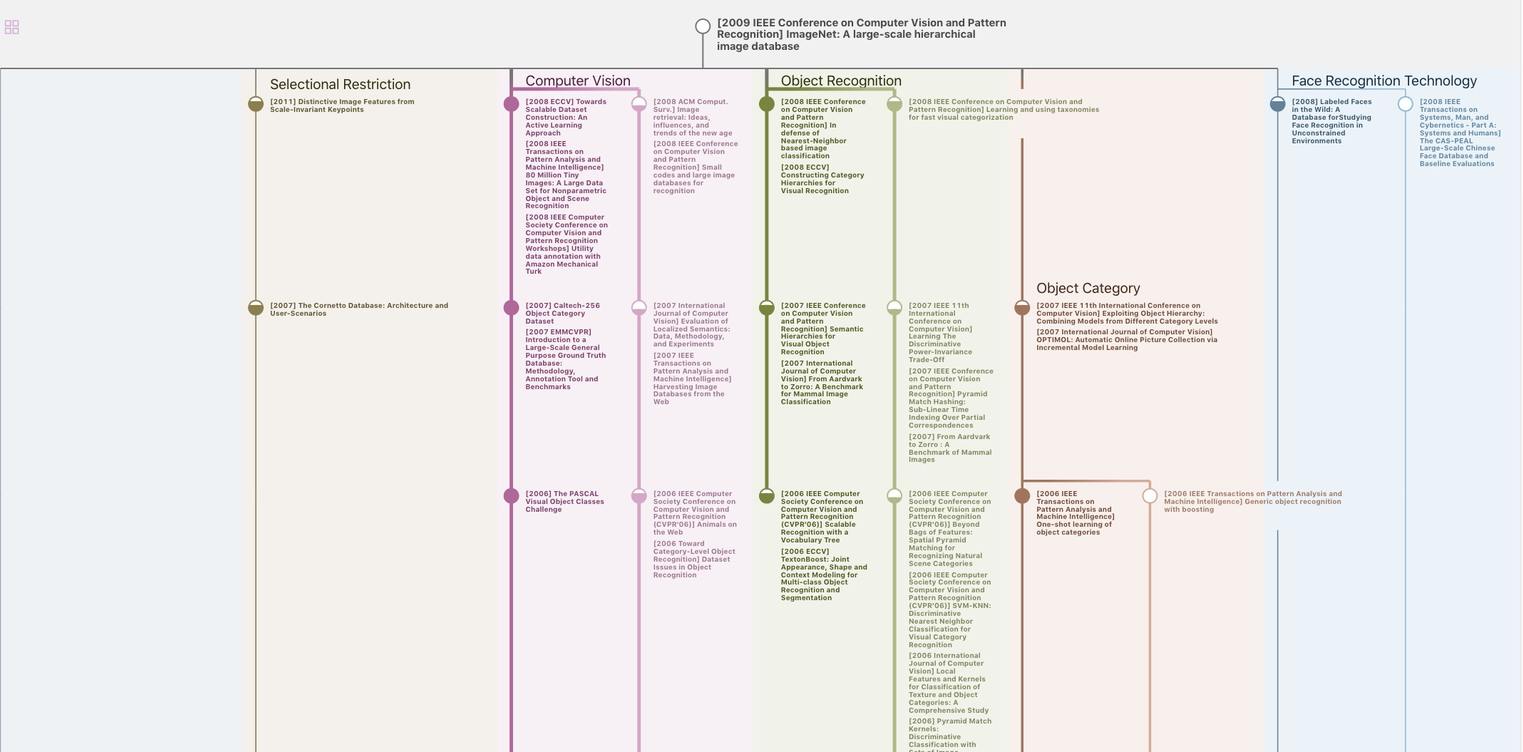
生成溯源树,研究论文发展脉络
Chat Paper
正在生成论文摘要