Time Series Clustering via Inter-network Heterogeneity
crossref(2024)
摘要
Time series clustering is a prominent research area with broad applications. In light of the complexity and diversity of contemporary time series data, we introduce a novel method for time series clustering based on inter-network heterogeneity. Firstly, we convert each time series into a network by using two types of time series segmentation methods. Secondly, we introduce an inter-network clustering technique based on the spectral theory of graphs. By measuring the total variation (TV) distance between the empirical spectral distribution of each network, and identify distinct clusters by using a hierarchical clustering method. Our approach effectively preserves a greater number of distinctive features inherent in the original time series. To evaluate the performance of our method, simulation studies including the recognition of both stationary and non-stationary sequences are performed. Our approach also works well for real-world datasets, such as stock closing prices and sleep electroencephalogram (EEG) data. These results demonstrate that our approach has the capability to handle non-stationary sequence and identifying the intrinsic correlations in time series.
更多查看译文
AI 理解论文
溯源树
样例
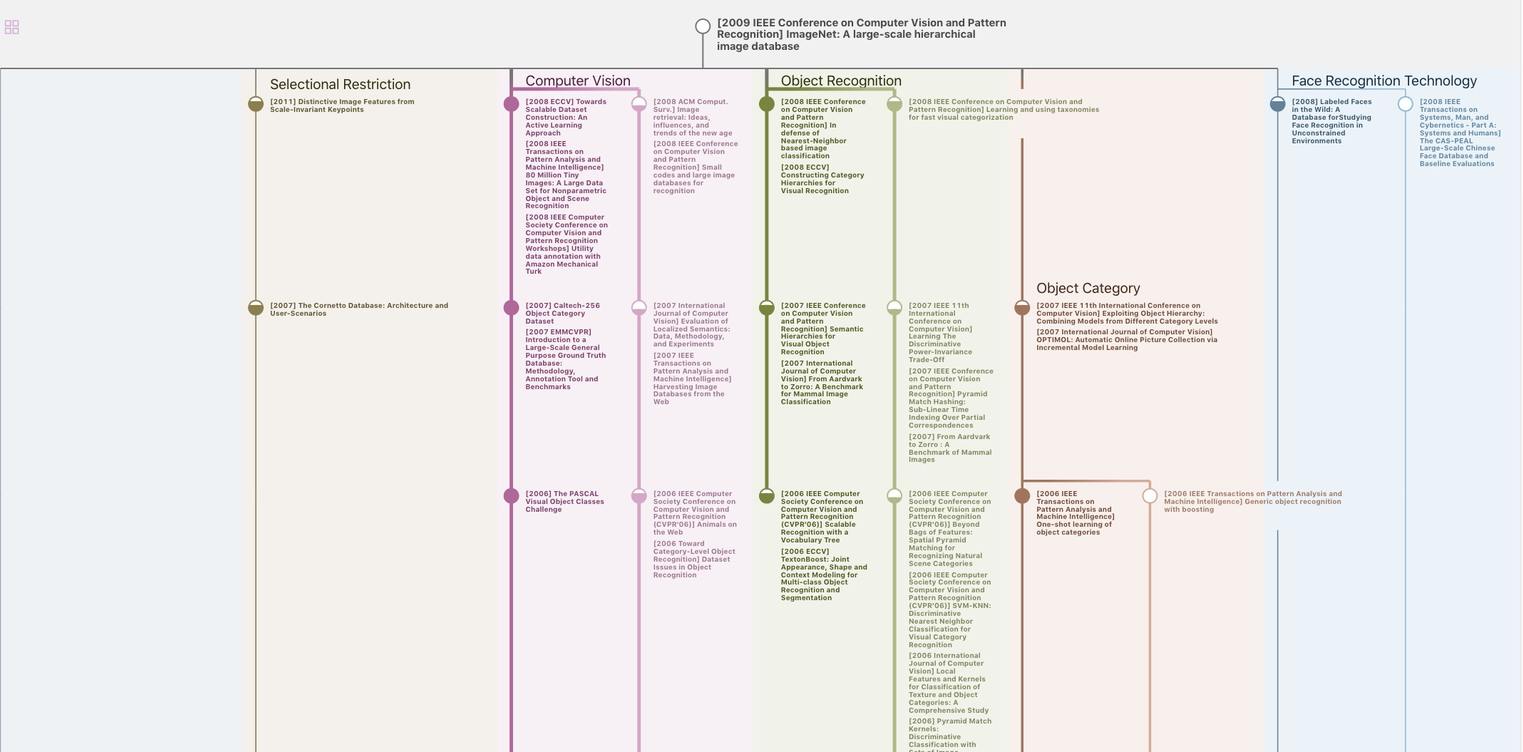
生成溯源树,研究论文发展脉络
Chat Paper
正在生成论文摘要