DeepGleason: a System for Automated Gleason Grading of Prostate Cancer Using Deep Neural Networks
arXiv (Cornell University)(2024)
University Hospital Augsburg Institute for Digital Medicine | University Hospital Augsburg Institute for Pathology and Molecular Diagnostics | BioM Biotech Cluster Development GmbH Institute for Pathology and Molecular Diagnostics | University Hospital Augsburg Bavarian Cancer Research Center (BZKF)
Abstract
Advances in digital pathology and artificial intelligence (AI) offerpromising opportunities for clinical decision support and enhancing diagnosticworkflows. Previous studies already demonstrated AI's potential for automatedGleason grading, but lack state-of-the-art methodology and model reusability.To address this issue, we propose DeepGleason: an open-source deep neuralnetwork based image classification system for automated Gleason grading usingwhole-slide histopathology images from prostate tissue sections. Implementedwith the standardized AUCMEDI framework, our tool employs a tile-wiseclassification approach utilizing fine-tuned image preprocessing techniques incombination with a ConvNeXt architecture which was compared to variousstate-of-the-art architectures. The neural network model was trained andvalidated on an in-house dataset of 34,264 annotated tiles from 369 prostatecarcinoma slides. We demonstrated that DeepGleason is capable of highlyaccurate and reliable Gleason grading with a macro-averaged F1-score of 0.806,AUC of 0.991, and Accuracy of 0.974. The internal architecture comparisonrevealed that the ConvNeXt model was superior performance-wise on our datasetto established and other modern architectures like transformers. Furthermore,we were able to outperform the current state-of-the-art in tile-wisefine-classification with a sensitivity and specificity of 0.94 and 0.98 forbenign vs malignant detection as well as of 0.91 and 0.75 for Gleason 3 vsGleason 4 5 classification, respectively. Our tool contributes to the wideradoption of AI-based Gleason grading within the research community and pavesthe way for broader clinical application of deep learning models in digitalpathology. DeepGleason is open-source and publicly available for researchapplication in the following Git repository:https://github.com/frankkramer-lab/DeepGleason.
MoreTranslated text
Key words
Deep Learning,Medical Image Analysis,Computer-Aided Detection,Whole Slide Imaging
PDF
View via Publisher
AI Read Science
AI Summary
AI Summary is the key point extracted automatically understanding the full text of the paper, including the background, methods, results, conclusions, icons and other key content, so that you can get the outline of the paper at a glance.
Example
Background
Key content
Introduction
Methods
Results
Related work
Fund
Key content
- Pretraining has recently greatly promoted the development of natural language processing (NLP)
- We show that M6 outperforms the baselines in multimodal downstream tasks, and the large M6 with 10 parameters can reach a better performance
- We propose a method called M6 that is able to process information of multiple modalities and perform both single-modal and cross-modal understanding and generation
- The model is scaled to large model with 10 billion parameters with sophisticated deployment, and the 10 -parameter M6-large is the largest pretrained model in Chinese
- Experimental results show that our proposed M6 outperforms the baseline in a number of downstream tasks concerning both single modality and multiple modalities We will continue the pretraining of extremely large models by increasing data to explore the limit of its performance
Try using models to generate summary,it takes about 60s
Must-Reading Tree
Example
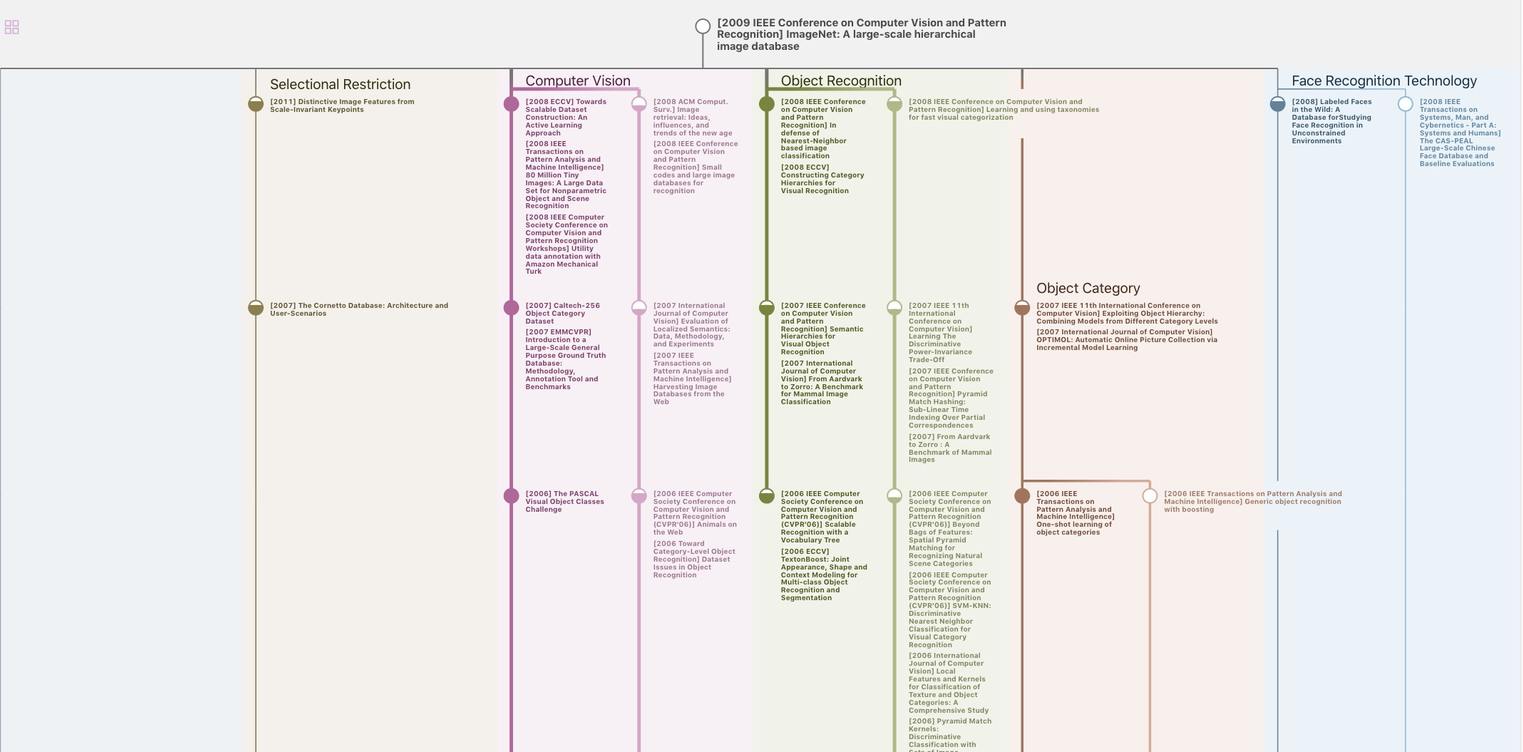
Generate MRT to find the research sequence of this paper
Related Papers
Data Disclaimer
The page data are from open Internet sources, cooperative publishers and automatic analysis results through AI technology. We do not make any commitments and guarantees for the validity, accuracy, correctness, reliability, completeness and timeliness of the page data. If you have any questions, please contact us by email: report@aminer.cn
Chat Paper
去 AI 文献库 对话