The Sample Complexity of Simple Binary Hypothesis Testing
CoRR(2024)
摘要
The sample complexity of simple binary hypothesis testing is the smallest
number of i.i.d. samples required to distinguish between two distributions p
and q in either: (i) the prior-free setting, with type-I error at most
α and type-II error at most β; or (ii) the Bayesian setting, with
Bayes error at most δ and prior distribution (α, 1-α). This
problem has only been studied when α = β (prior-free) or α =
1/2 (Bayesian), and the sample complexity is known to be characterized by the
Hellinger divergence between p and q, up to multiplicative constants. In
this paper, we derive a formula that characterizes the sample complexity (up to
multiplicative constants that are independent of p, q, and all error
parameters) for: (i) all 0 ≤α, β≤ 1/8 in the prior-free
setting; and (ii) all δ≤α/4 in the Bayesian setting. In
particular, the formula admits equivalent expressions in terms of certain
divergences from the Jensen–Shannon and Hellinger families. The main technical
result concerns an f-divergence inequality between members of the
Jensen–Shannon and Hellinger families, which is proved by a combination of
information-theoretic tools and case-by-case analyses. We explore applications
of our results to robust and distributed (locally-private and
communication-constrained) hypothesis testing.
更多查看译文
AI 理解论文
溯源树
样例
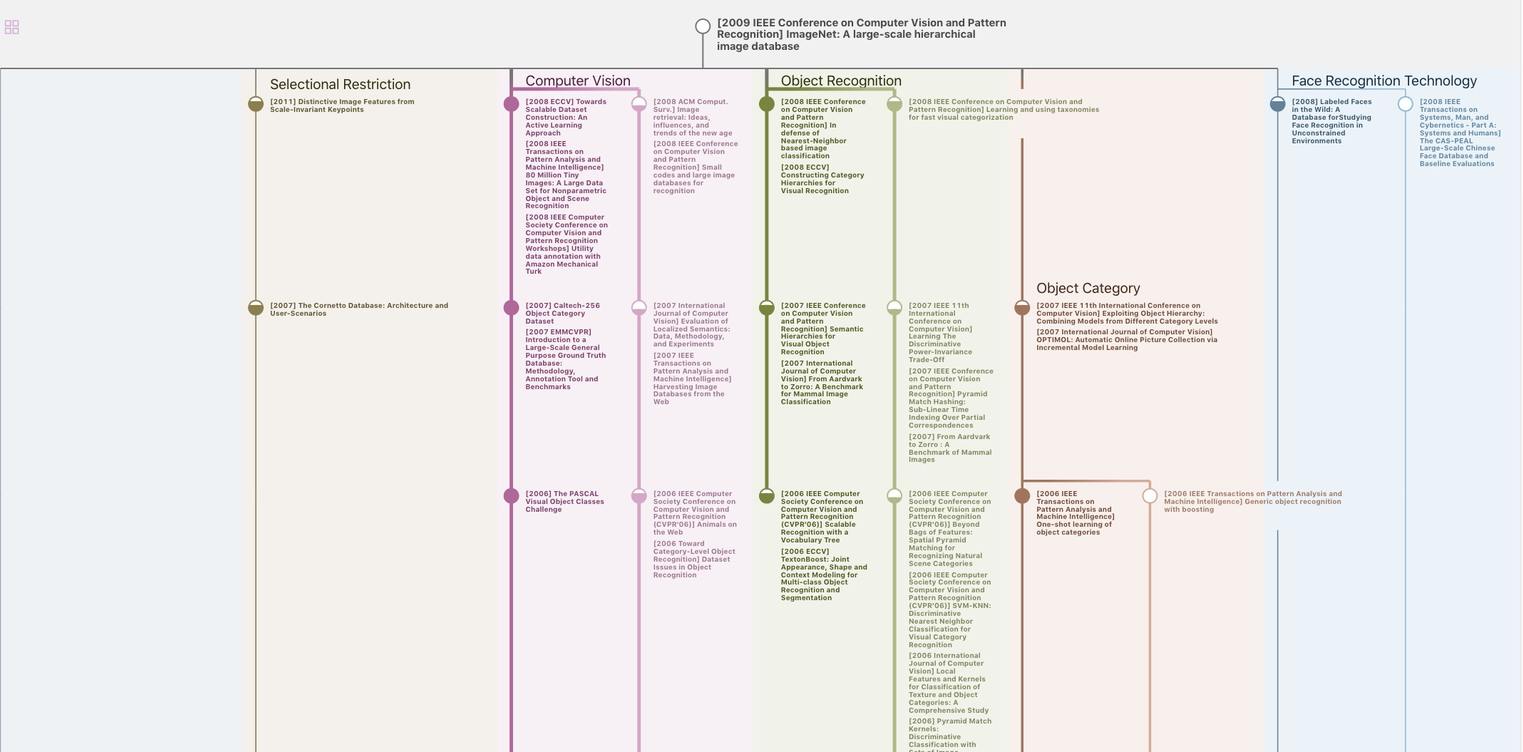
生成溯源树,研究论文发展脉络
Chat Paper
正在生成论文摘要