Uncertainty Quantification in Autoencoders Predictions: Applications in Aerodynamics
Journal of Computational Physics(2024)
摘要
A data-driven model is compared to classical equation-driven approaches to investigate its ability to predict quantity of interest and their uncertainty when studying airfoil aerodynamics. The focus is on autoencoders and the effect of uncertainties due to the architecture, the hyperparamaters and the choice of the training data (internal or model-form uncertainties). Comparisons with a Gaussian Process regression approach clearly illustrate the autoencoder advantage in extracting useful information on the prediction confidence even in the absence of ground truth data. Simulations accounting for internal uncertainties are also compared to the impact of the variability induced by uncertain operating conditions (external uncertainties) showing the importance of accounting for the total uncertainty when establishing prediction confidence.
更多查看译文
关键词
Uncertainty Quantification,Machine Learning,Autoencoder,Airfoil Aerodynamics
AI 理解论文
溯源树
样例
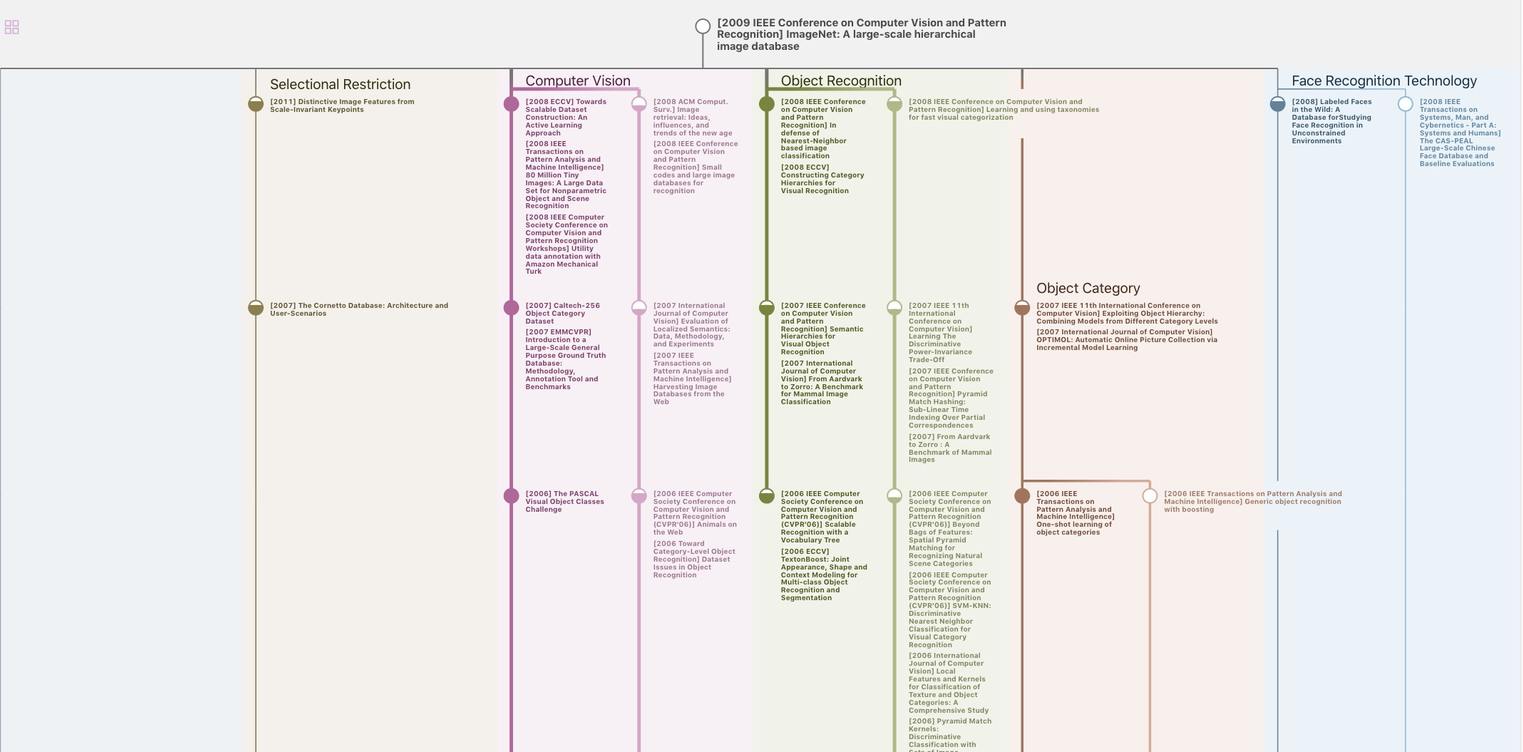
生成溯源树,研究论文发展脉络
Chat Paper
正在生成论文摘要