A Multi-Class Classification Approach for Anemia Level Prediction with Machine Learning Models
2023 8th South-East Europe Design Automation, Computer Engineering, Computer Networks and Social Media Conference (SEEDA-CECNSM)(2023)
Abstract
It is a common belief that Artificial Intelligence (AI) and Machine Learning (ML) provide researchers and medical experts with concepts, tools, and techniques to build intelligent systems able to analyze, process and detect hidden patterns, in order to turn data into actionable knowledge for personalized medicine and decision-making (e.g., disease prevention via associated risk prediction, treatment, etc.). The present study seeks an ML solution that will facilitate the recognition of anemia level by investigating the performance of two well-established strategies for multi-class classification tasks; the One-Vs-All (OVA) and One-Vs-One (OVO). Under the specific strategies, two well-known ML models were assumed, namely, Logistic Regression (LR) and Support Vector Machines (SVM). The validation of both strategies was carried out in a publicly available dataset by measuring the weighted average performance in all involved classes that capture the anemia levels; accuracy, precision, recall and Area Under the ROC curve (AUC) were captured and compared for the identification of the dominant model and strategy. After the experimental evaluation, the OVO strategy with the LR model is the main suggestion of the current study achieving an accuracy of 95.05 %, precision and recall equal to 0.951 and an AUC of 0.990.
MoreTranslated text
AI Read Science
Must-Reading Tree
Example
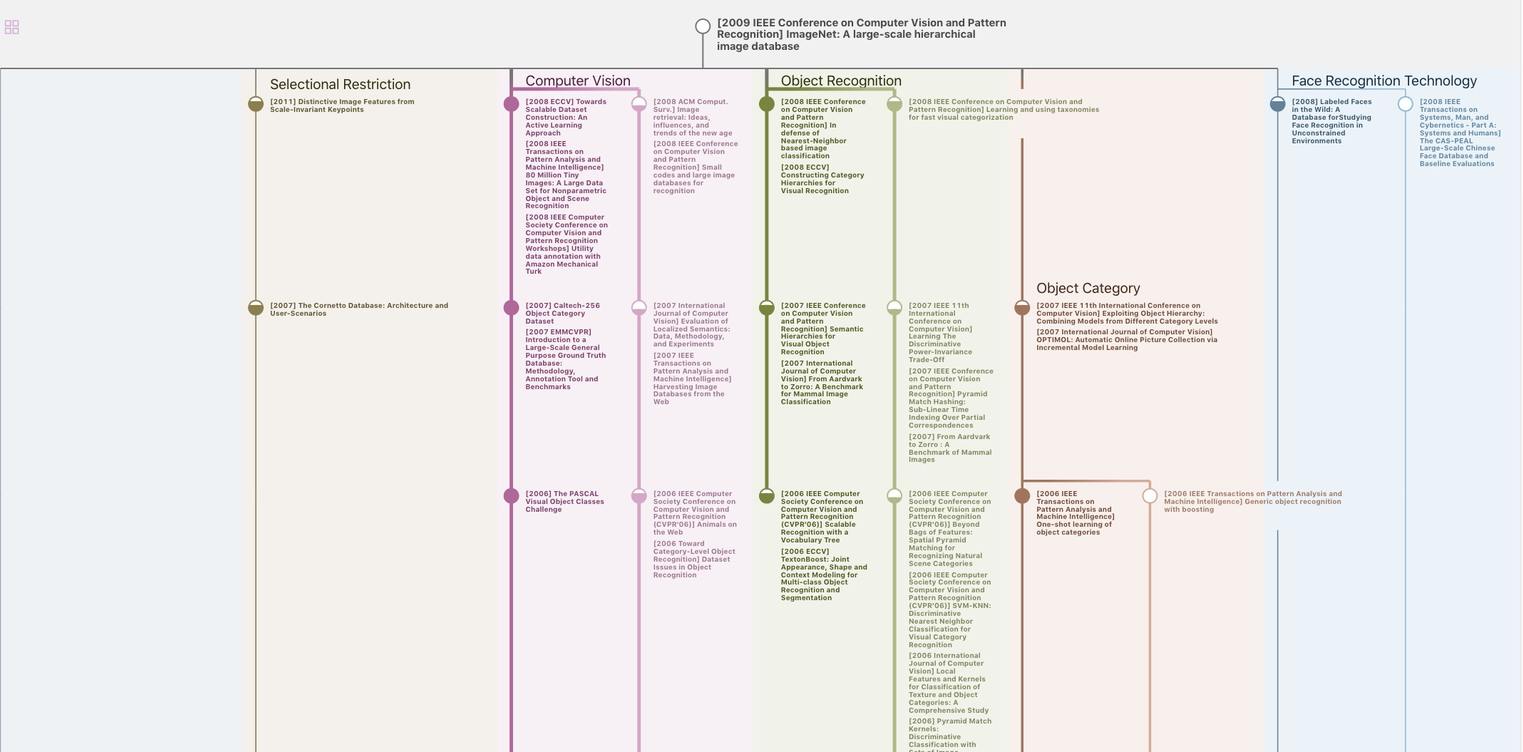
Generate MRT to find the research sequence of this paper
Chat Paper
Summary is being generated by the instructions you defined