Hierarchical Medical Image Report Adversarial Generation with Hybrid Discriminator
Artificial intelligence in medicine(2024)
摘要
Background and objectivesGenerating coherent reports from medical images is an important task for reducing doctors' workload. Unlike traditional image captioning tasks, the task of medical image report generation faces more challenges. Current models for generating reports from medical images often fail to characterize some abnormal findings, and some models generate reports with low quality. In this study, we propose a model to generate high-quality reports from medical images.MethodsIn this paper, we propose a model called Hybrid Discriminator Generative Adversarial Network (HDGAN), which combines Generative Adversarial Network (GAN) with Reinforcement Learning (RL). The HDGAN model consists of a generator, a one-sentence discriminator, and a one-word discriminator. Specifically, the RL reward signals are judged on the one-sentence discriminator and one-word discriminator separately. The one-sentence discriminator can better learn sentence-level structural information, while the one-word discriminator can learn word diversity information effectively.ResultsOur approach performs better on the IU-X-ray and COV-CTR datasets than the baseline models. For the ROUGE metric, our method outperforms the state-of-the-art model by 0.36 on the IU-X-ray, 0.06 on the MIMIC-CXR and 0.156 on the COV-CTR.ConclusionsThe compositional framework we proposed can generate more accurate medical image reports at different levels.
更多查看译文
关键词
Generative adversarial network,Medical image report generation,Reinforcement learning
AI 理解论文
溯源树
样例
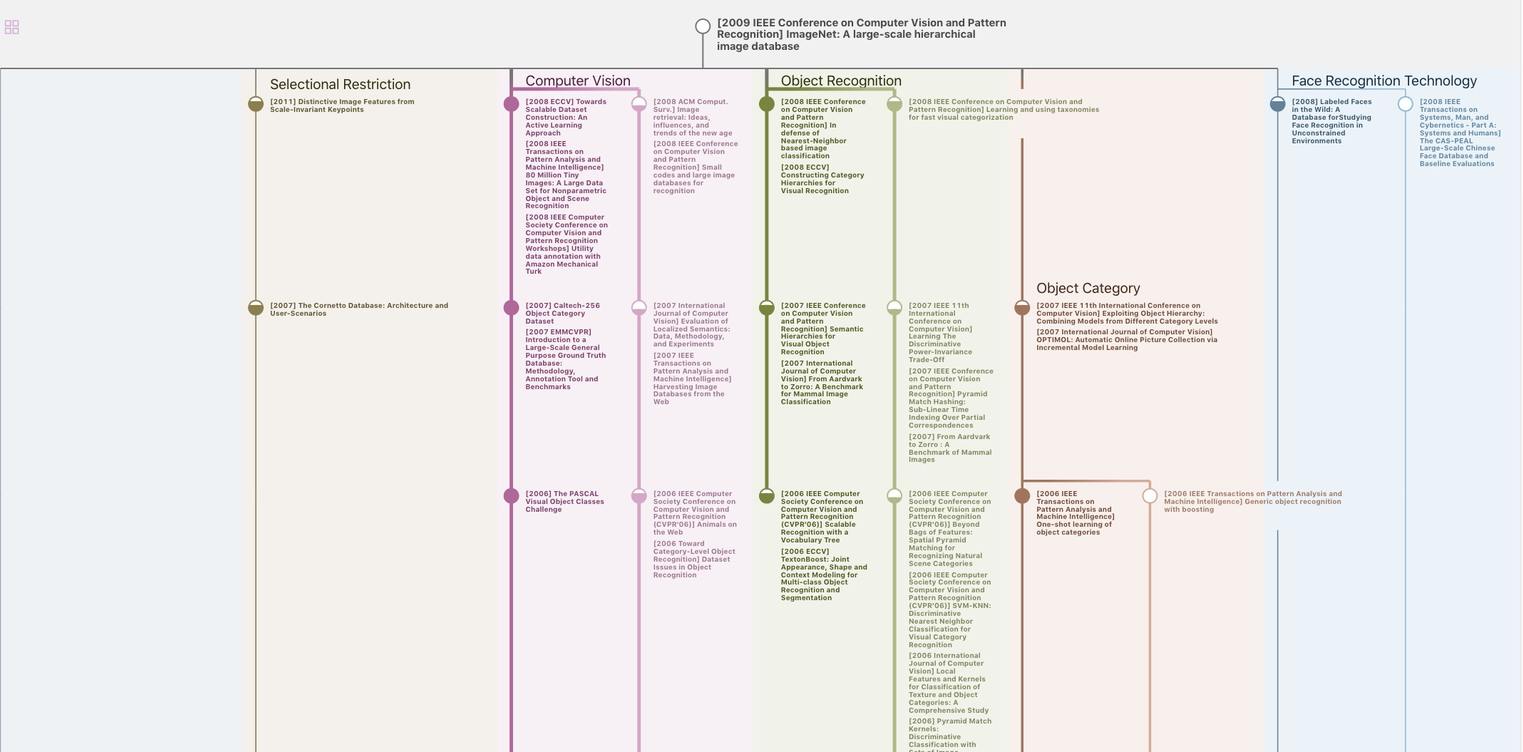
生成溯源树,研究论文发展脉络
Chat Paper
正在生成论文摘要