OmniGlue: Generalizable Feature Matching with Foundation Model Guidance
2024 IEEE/CVF Conference on Computer Vision and Pattern Recognition (CVPR)(2024)
摘要
The image matching field has been witnessing a continuous emergence of novellearnable feature matching techniques, with ever-improving performance onconventional benchmarks. However, our investigation shows that despite thesegains, their potential for real-world applications is restricted by theirlimited generalization capabilities to novel image domains. In this paper, weintroduce OmniGlue, the first learnable image matcher that is designed withgeneralization as a core principle. OmniGlue leverages broad knowledge from avision foundation model to guide the feature matching process, boostinggeneralization to domains not seen at training time. Additionally, we propose anovel keypoint position-guided attention mechanism which disentangles spatialand appearance information, leading to enhanced matching descriptors. Weperform comprehensive experiments on a suite of 7 datasets with varied imagedomains, including scene-level, object-centric and aerial images. OmniGlue'snovel components lead to relative gains on unseen domains of 20.9% withrespect to a directly comparable reference model, while also outperforming therecent LightGlue method by 9.5% relatively.Code and model can be found athttps://hwjiang1510.github.io/OmniGlue
更多查看译文
关键词
Feature Matching,Foundation Model,Training Time,Image Registration,Attention Mechanism,Core Principles,Image Domain,Matching Process,Broad Knowledge,Relative Gain,Local Features,Precision And Recall,Image Pairs,Generalization Capability,Variety Of Domains,Target Domain,Cyanoacrylate,Pose Estimation,Matching Results,Information Propagation,Local Descriptors,Sparse Method,Position Features,Training Domain,Attention Layer,Positional Encoding,Source Domain,Matching Module,Homography,Propagation Characteristics
AI 理解论文
溯源树
样例
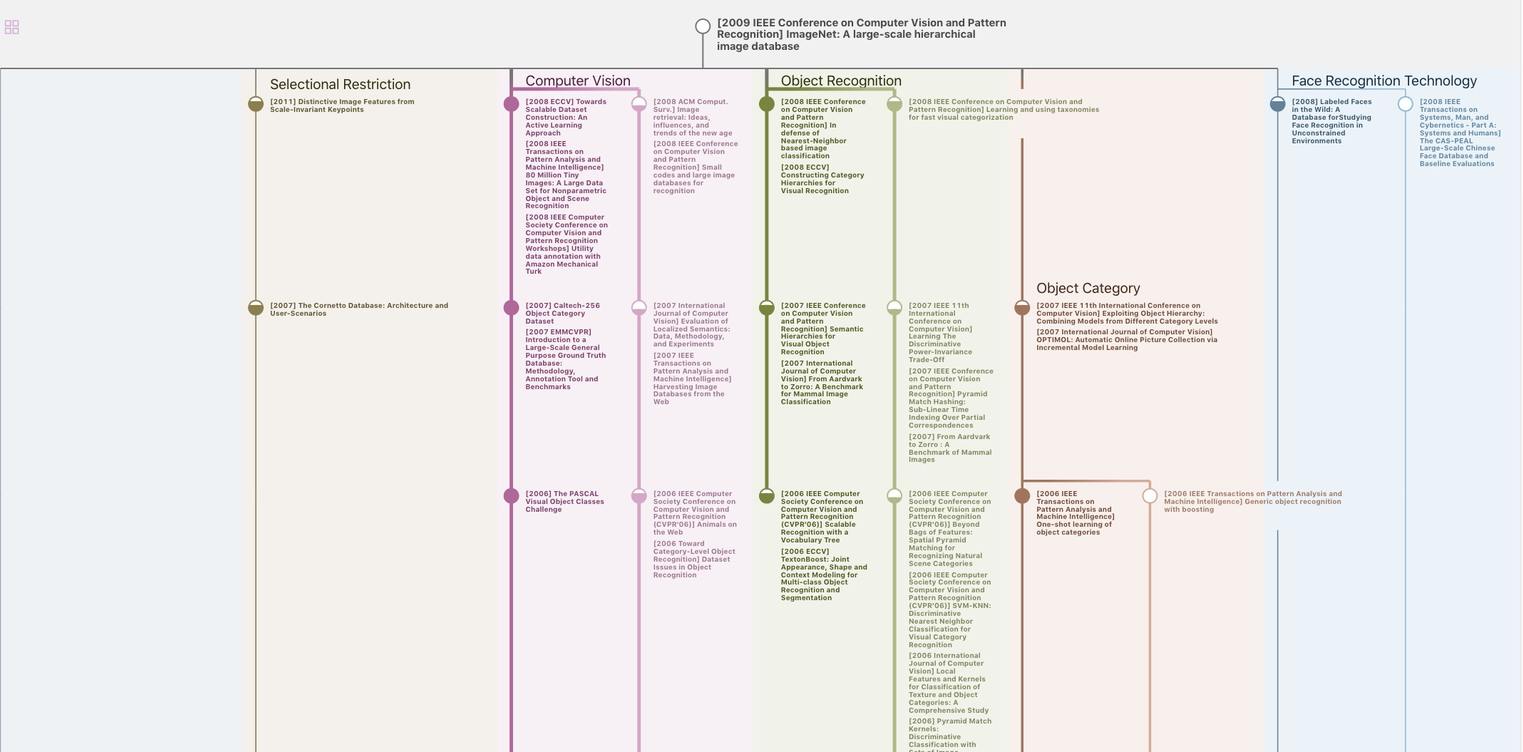
生成溯源树,研究论文发展脉络
Chat Paper
正在生成论文摘要