Stable Neighbor Denoising for Source-free Domain Adaptive Segmentation.
CVPR 2024(2024)
Abstract
We study source-free unsupervised domain adaptation (SFUDA) for semanticsegmentation, which aims to adapt a source-trained model to the target domainwithout accessing the source data. Many works have been proposed to addressthis challenging problem, among which uncertainty-based self-training is apredominant approach. However, without comprehensive denoising mechanisms, theystill largely fall into biased estimates when dealing with different domainsand confirmation bias. In this paper, we observe that pseudo-label noise ismainly contained in unstable samples in which the predictions of most pixelsundergo significant variations during self-training. Inspired by this, wepropose a novel mechanism to denoise unstable samples with stable ones.Specifically, we introduce the Stable Neighbor Denoising (SND) approach, whicheffectively discovers highly correlated stable and unstable samples by nearestneighbor retrieval and guides the reliable optimization of unstable samples bybi-level learning. Moreover, we compensate for the stable set by object-levelobject paste, which can further eliminate the bias caused by less learnedclasses. Our SND enjoys two advantages. First, SND does not require a specificsegmentor structure, endowing its universality. Second, SND simultaneouslyaddresses the issues of class, domain, and confirmation biases duringadaptation, ensuring its effectiveness. Extensive experiments show that SNDconsistently outperforms state-of-the-art methods in various SFUDA semanticsegmentation settings. In addition, SND can be easily integrated with otherapproaches, obtaining further improvements.
MoreTranslated text
Key words
Domain Adaptation,Domain Adaptive Segmentation,Semantic Segmentation,Target Domain,Confirmation Bias,Stable Set,Stable Ones,Learning Rate,Weight Decay,Domain Shift,Segmentation Model,Version Of Task,Segmentation Task,Iterative Optimization,Probability Threshold,Outer Loop,Source Domain,Spatial Layout,Unbiased Way,Bilevel Optimization,Style Image,Debiasing,Stability Metrics,Uncertainty Map,Missing Category,Source Domain Data
PDF
PPT
Code
Data
View via Publisher
AI Read Science
Must-Reading Tree
Example
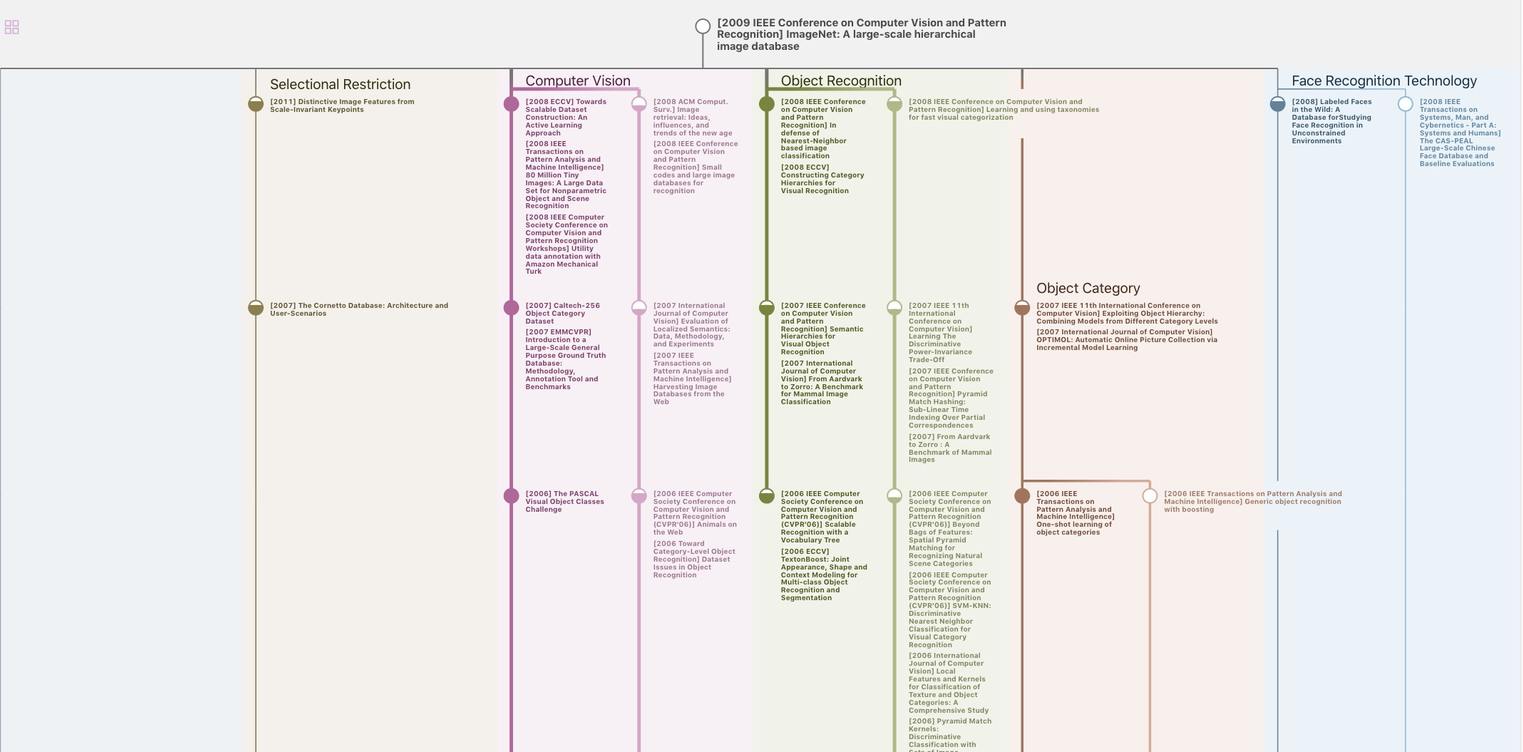
Generate MRT to find the research sequence of this paper
Data Disclaimer
The page data are from open Internet sources, cooperative publishers and automatic analysis results through AI technology. We do not make any commitments and guarantees for the validity, accuracy, correctness, reliability, completeness and timeliness of the page data. If you have any questions, please contact us by email: report@aminer.cn
Chat Paper
Summary is being generated by the instructions you defined