Open-Vocabulary Attention Maps with Token Optimization for Semantic Segmentation in Diffusion Models
2024 IEEE/CVF Conference on Computer Vision and Pattern Recognition (CVPR)(2024)
摘要
Diffusion models represent a new paradigm in text-to-image generation. Beyondgenerating high-quality images from text prompts, models such as StableDiffusion have been successfully extended to the joint generation of semanticsegmentation pseudo-masks. However, current extensions primarily rely onextracting attentions linked to prompt words used for image synthesis. Thisapproach limits the generation of segmentation masks derived from word tokensnot contained in the text prompt. In this work, we introduce Open-VocabularyAttention Maps (OVAM)-a training-free method for text-to-image diffusion modelsthat enables the generation of attention maps for any word. In addition, wepropose a lightweight optimization process based on OVAM for finding tokensthat generate accurate attention maps for an object class with a singleannotation. We evaluate these tokens within existing state-of-the-art StableDiffusion extensions. The best-performing model improves its mIoU from 52.1 to86.6 for the synthetic images' pseudo-masks, demonstrating that our optimizedtokens are an efficient way to improve the performance of existing methodswithout architectural changes or retraining.
更多查看译文
关键词
Synthetic Data,Semantic Segmentation,Diffusion Model,Text-to-Image,Token Optimization,Attention
AI 理解论文
溯源树
样例
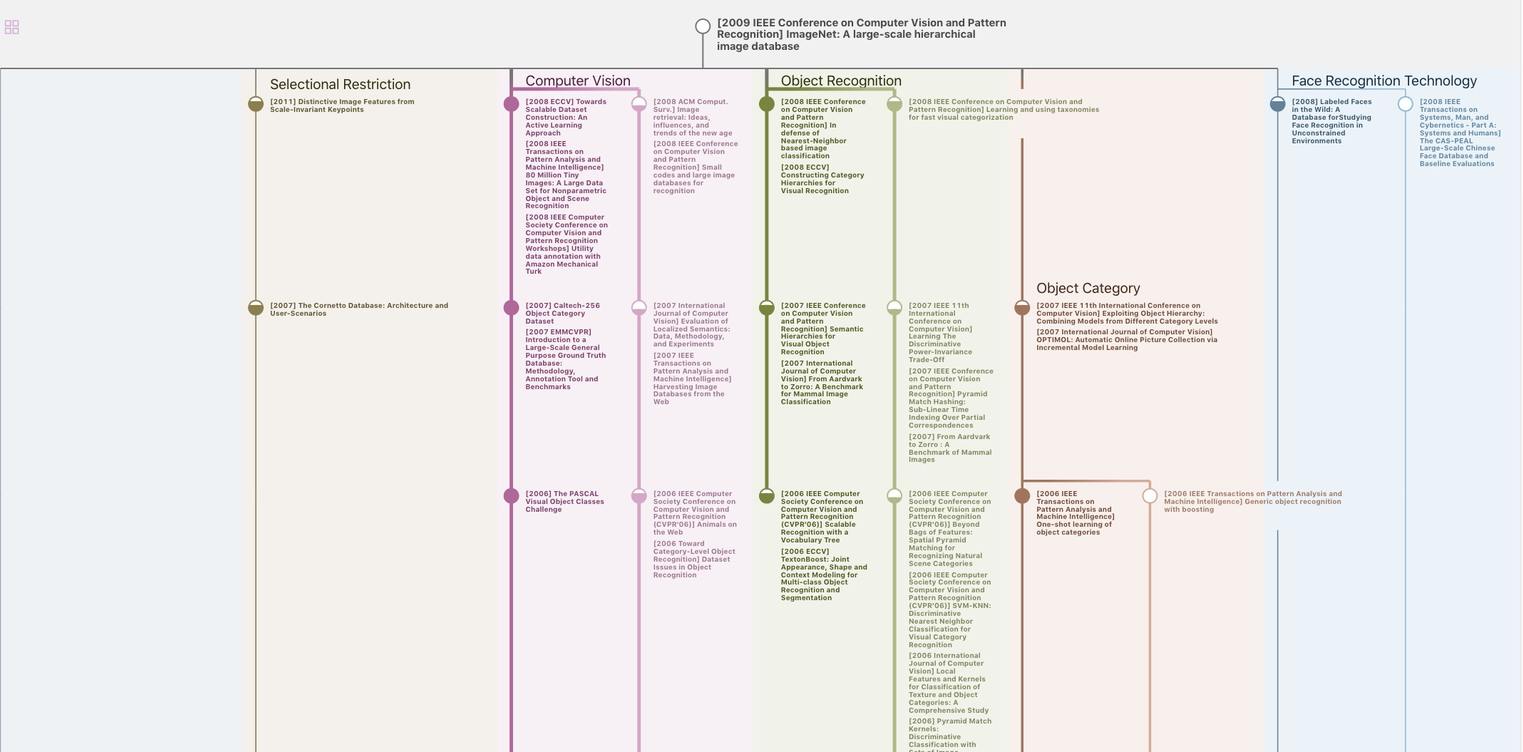
生成溯源树,研究论文发展脉络
Chat Paper
正在生成论文摘要