A cost-effective, machine learning-driven approach for screening arterial functional aging in a large-scale Chinese population
FRONTIERS IN PUBLIC HEALTH(2024)
摘要
Introduction An easily accessible and cost-free machine learning model based on prior probabilities of vascular aging enables an application to pinpoint high-risk populations before physical checks and optimize healthcare investment.Methods A dataset containing questionnaire responses and physical measurement parameters from 77,134 adults was extracted from the electronic records of the Health Management Center at the Third Xiangya Hospital. The least absolute shrinkage and selection operator and recursive feature elimination-Lightweight Gradient Elevator were employed to select features from a pool of potential covariates. The participants were randomly divided into training (70%) and test cohorts (30%). Four machine learning algorithms were applied to build the screening models for elevated arterial stiffness (EAS), and the performance of models was evaluated by calculating the area under the receiver operating characteristic curve (AUC), sensitivity, specificity, and accuracy.Results Fourteen easily accessible features were selected to construct the model, including "systolic blood pressure" (SBP), "age," "waist circumference," "history of hypertension," "sex," "exercise," "awareness of normal blood pressure," "eat fruit," "work intensity," "drink milk," "eat bean products," "smoking," "alcohol consumption," and "Irritableness." The extreme gradient boosting (XGBoost) model outperformed the other three models, achieving AUC values of 0.8722 and 0.8710 in the training and test sets, respectively. The most important five features are SBP, age, waist, history of hypertension, and sex.Conclusion The XGBoost model ideally assesses the prior probability of the current EAS in the general population. The integration of the model into primary care facilities has the potential to lower medical expenses and enhance the management of arterial aging.
更多查看译文
关键词
machine learning,XGBoost,arterial stiffness,physical examination,questionnaire,feature
AI 理解论文
溯源树
样例
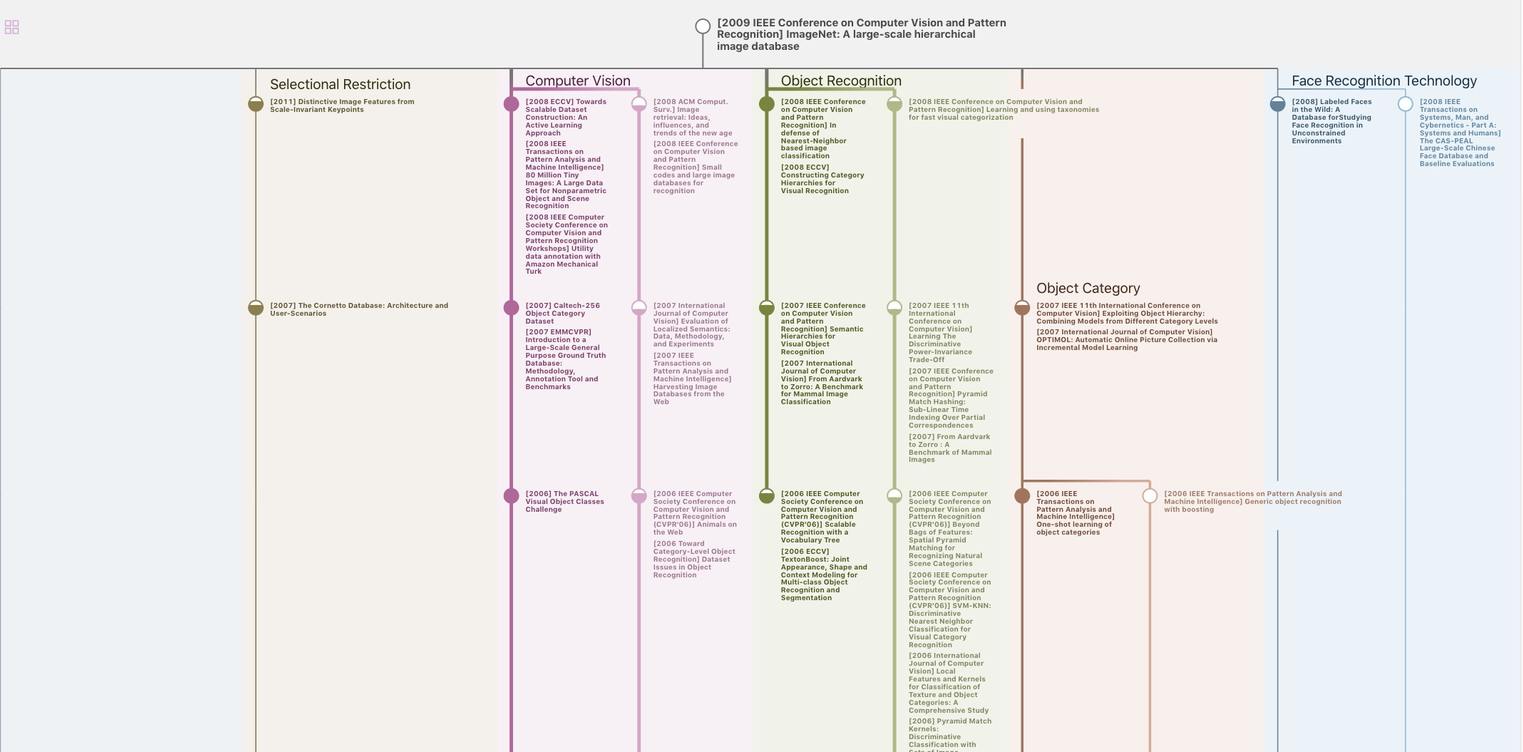
生成溯源树,研究论文发展脉络
Chat Paper
正在生成论文摘要