Induction Motor Inter-turn Short Circuit Fault Classification by Extracting Auto Features Using LSTM Neural Network
2024 IEEE 3rd International Conference on Control, Instrumentation, Energy & Communication (CIEC)(2024)
摘要
Inter Turn Short Circuit (ITSC) fault in stator winding of three phase induction motor (IM) is one of the most recurrent type of failures, which counts more than 30% of total electrical faults in machine. Thus, the investigation of ITSC fault detection in early stage is essential, since IMs are widely used in industries and household applications. The proposed work exploits the facility of deep learning-based approaches for ITSC fault detection in IMs using auto feature extraction enabled Long Short-Term Memory (LSTM) Neural network. Initially, three phase stator current envelop is extracted by Hilbert Transform and the envelop samples are used further for auto feature extraction. Along with, modified weighted batch normalization (BN) is used to improve the training capability of proposed method. MATLAB/ SIMULINK model with three different IM parameters along with three different loading conditions are used to train the proposed diagnostic system for eight different stator fault conditions. The method is also experimentally tested with 1 hp IM to check the accuracy of the diagnostic system. The performance of proposed method is compared with statistical feature extraction with ANN and from the result, it is observed that auto feature extraction using LSTM neural network is more accurate (up to 98%) than previous one.
更多查看译文
关键词
Hilbert Transform (HT),Auto features,Long Short Term memory (LSTM),Weighted Batch Normalization
AI 理解论文
溯源树
样例
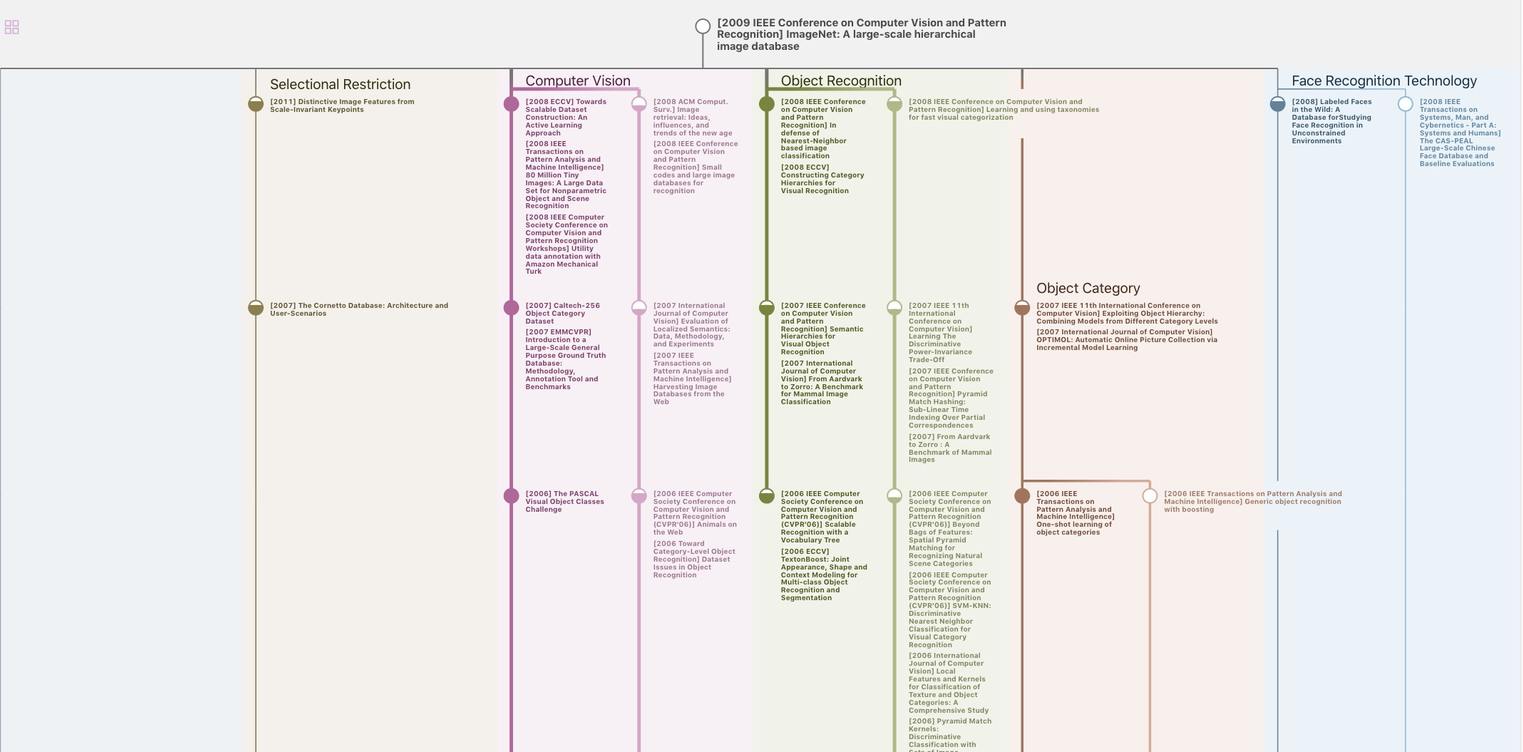
生成溯源树,研究论文发展脉络
Chat Paper
正在生成论文摘要