SPD Manifold-Based Graph Neural Network for Fault Diagnosis of Harmonic Drive
2023 3rd International Conference on Electronic Information Engineering and Computer Communication (EIECC)(2023)
摘要
Harmonic drive (HD), as an essential component of industrial robots, is susceptible to damage due to product manufacturing and working conditions. Therefore, it is imperative to conduct a comprehensive test to accurately diagnose potential HD faults. In this paper, a SPD Manifold-based Graph Neural Network (SPD-GNN) is proposed to accurately identify different faults of HD. In the time-frequency domain, the high-definition vibration signals obtained from multiple sensors are segmented. The resulting multi-channel spatial covariance matrices (MSCM) act as vertices in a time-frequency graph. A SPD-GNN is then utilized to extract classification details while preserving discriminative capabilities. Subsequently, the SPD matrices are projected to the tangent space using a logarithmic mapping (LOG) layer. Ultimately, the data is input into a cross-entropy loss function for subsequent computation. The SPD-GNN uses graph convolutional techniques tailored for SPD matrices to capture HD features in the time-frequency domain.
更多查看译文
AI 理解论文
溯源树
样例
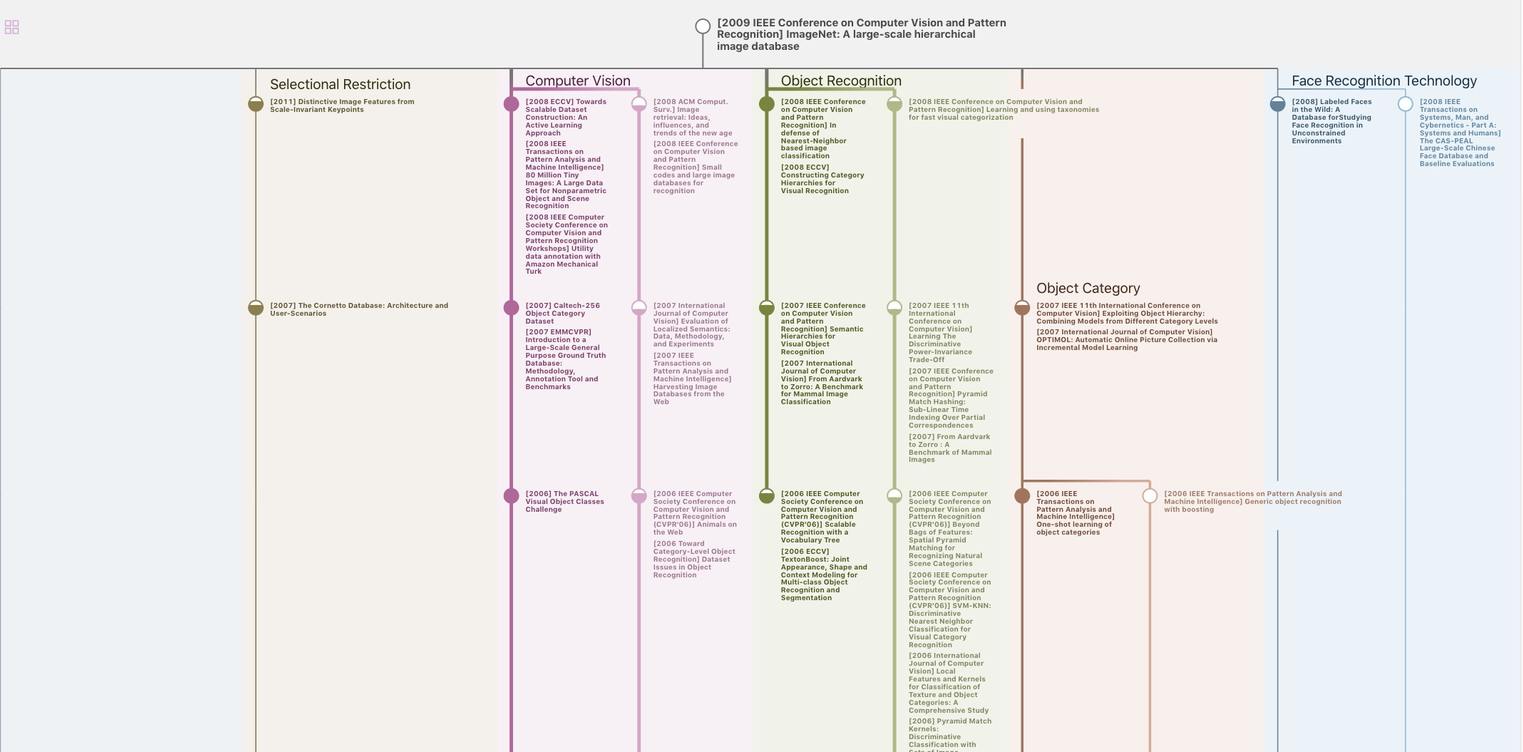
生成溯源树,研究论文发展脉络
Chat Paper
正在生成论文摘要