Novel Adversarial Unsupervised Subdomain Adaption Multi-Channel Deep Convolutional Network for Cross-Operating Fault Diagnosis of Rolling Bearings
IEEE ACCESS(2024)
摘要
Rolling bearings in production practice usually serve in a healthy state. Some fault state labels are scarce or even no labels, resulting in unbalanced data categories. Meanwhile, frequent working condition switching results in significant differences in data distribution among working conditions, and labeled data in some working states cannot be fully utilized. To deal with the challenge of low fault identification accuracy caused by these practical factors, this paper proposed a novel adversarial unsupervised subdomain adaption multi-channel deep convolutional network (ASMDCN). Firstly, a parallel three-channel depth feature extraction module is built, and a multi-scale convolution kernel is used to fully extract the rich features of vibration signals under various working conditions. Secondly, a novel loss function is designed to adequately consider the classification difficulty of samples and the degree of class imbalance. Finally, the adversarial training strategy is used to force the feature extractor to extract the domain invariant features, and the Local Maximum Mean discrepancy (LMMD) is used to align the global and related subdomains of the source and target domains. The experimental results show that the designed feature extraction can fully extract the domain-invariant features of the rolling bearings under different working conditions. Under the proposed objective function optimization, the network model can fully align the features of multi-source and single-target domain under unbalanced data and has strong generalization performance.
更多查看译文
关键词
Intelligent cross-domain fault diagnosis,unbalanced data,adversarial domain adaptation,subdomain adaptation
AI 理解论文
溯源树
样例
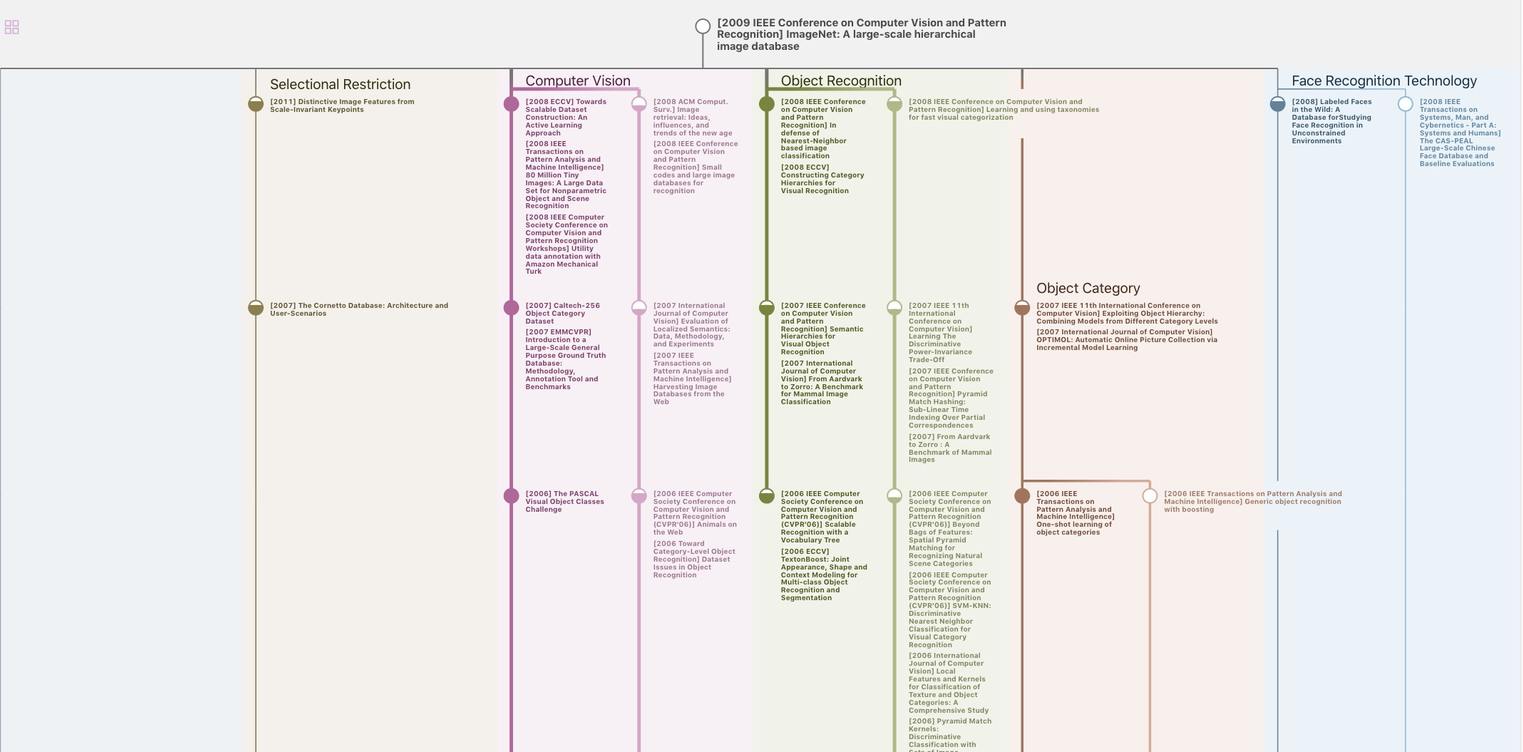
生成溯源树,研究论文发展脉络
Chat Paper
正在生成论文摘要