XPose: Explainable Human Pose Estimation
arXiv (Cornell University)(2024)
摘要
Current approaches in pose estimation primarily concentrate on enhancingmodel architectures, often overlooking the importance of comprehensivelyunderstanding the rationale behind model decisions. In this paper, we proposeXPose, a novel framework that incorporates Explainable AI (XAI) principles intopose estimation. This integration aims to elucidate the individual contributionof each keypoint to final prediction, thereby elevating the model'stransparency and interpretability. Conventional XAI techniques havepredominantly addressed tasks with single-target tasks like classification.Additionally, the application of Shapley value, a common measure in XAI, topose estimation has been hindered by prohibitive computational demands. To address these challenges, this work introduces an innovative conceptcalled Group Shapley Value (GSV). This approach strategically organizeskeypoints into clusters based on their interdependencies. Within theseclusters, GSV meticulously calculates Shapley value for keypoints, while forinter-cluster keypoints, it opts for a more holistic group-level valuation.This dual-level computation framework meticulously assesses keypointcontributions to the final outcome, optimizing computational efficiency.Building on the insights into keypoint interactions, we devise a novel dataaugmentation technique known as Group-based Keypoint Removal (GKR). This methodingeniously removes individual keypoints during training phases, deliberatelypreserving those with strong mutual connections, thereby refining the model'spredictive prowess for non-visible keypoints. The empirical validation of GKRacross a spectrum of standard approaches attests to its efficacy. GKR's successdemonstrates how using Explainable AI (XAI) can directly enhance poseestimation models.
更多查看译文
关键词
Pose Estimation,3D Human Pose
AI 理解论文
溯源树
样例
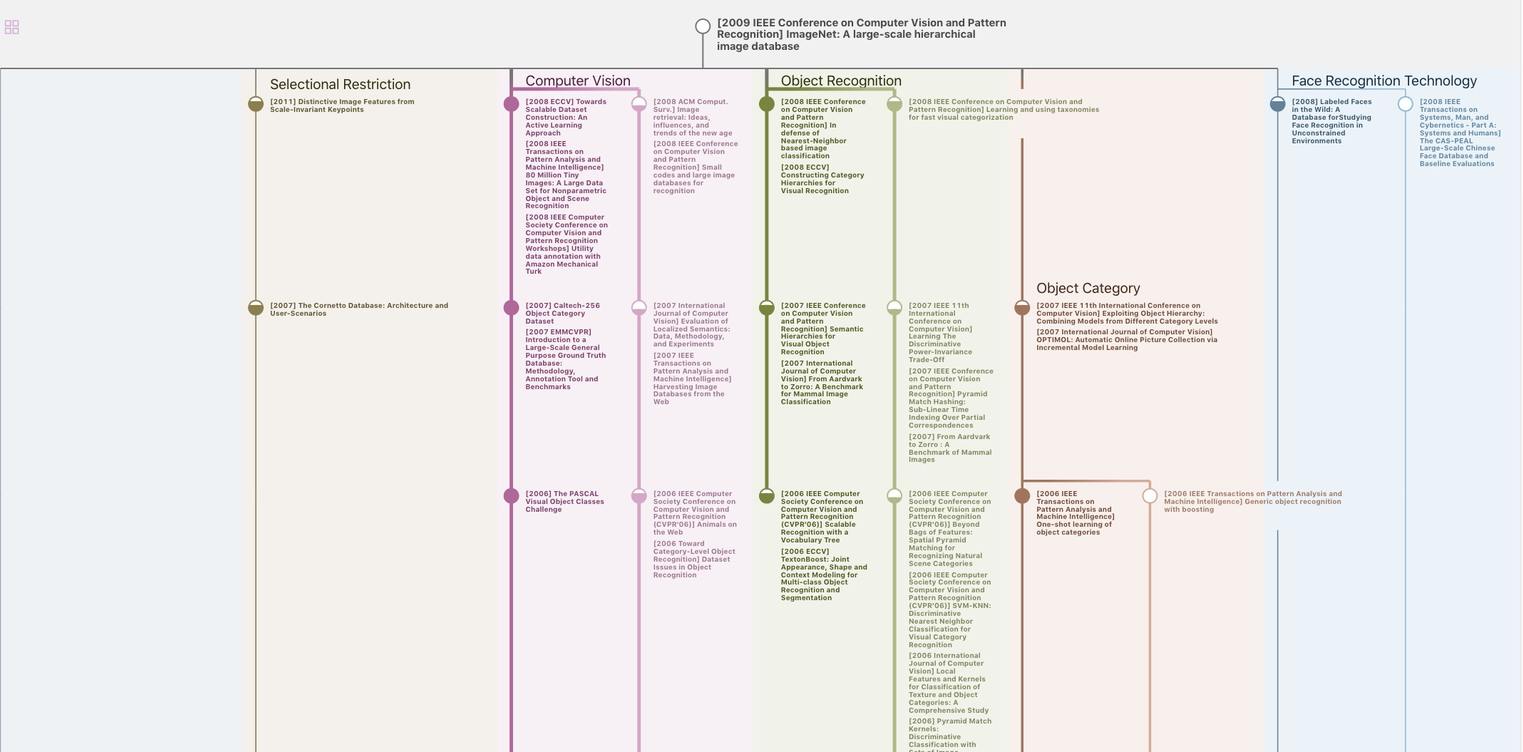
生成溯源树,研究论文发展脉络
Chat Paper
正在生成论文摘要