Ensuring Solution Uniqueness in Fixed-Point-Based Harmonic Power Flow Analysis with Converter-Interfaced Resources: Ex-post Conditions
IEEE Trans Smart Grid(2025)
University of Naples Federico II Department of Electrical Engineering and Information Technology | PSI NEPLAN AG
Abstract
Recently, the authors of this paper proposed a method for the HarmonicPower-Flow (HPF) calculus in polyphase grids with widespread deployment ofConverter-Interfaced Distributed Energy Resources (CIDERs). The HPF problem wasformulated by integrating the hybrid nodal equations of the grid with adetailed representation of the CIDERs hardware, sensing, and controls as LinearTime-Periodic (LTP) systems, and solving the resulting mismatch equations usingthe Newton-Raphson (NR) method. This work introduces a novel problemformulation based on the fixed-point algorithm that, combined with thecontraction property of the HPF problem, provides insights into the uniquenessof its solution. Notably, the effectiveness of the fixed-point formulation andthe uniqueness of the solution are evaluated through numerical analysesconducted on a modified version of the CIGRE low-voltage benchmark microgrid.
MoreTranslated text
Key words
converter-interfaced resources,distributed energy resources,harmonic power-flow study,fixed point method,solution uniqueness
PDF
View via Publisher
AI Read Science
AI Summary
AI Summary is the key point extracted automatically understanding the full text of the paper, including the background, methods, results, conclusions, icons and other key content, so that you can get the outline of the paper at a glance.
Example
Background
Key content
Introduction
Methods
Results
Related work
Fund
Key content
- Pretraining has recently greatly promoted the development of natural language processing (NLP)
- We show that M6 outperforms the baselines in multimodal downstream tasks, and the large M6 with 10 parameters can reach a better performance
- We propose a method called M6 that is able to process information of multiple modalities and perform both single-modal and cross-modal understanding and generation
- The model is scaled to large model with 10 billion parameters with sophisticated deployment, and the 10 -parameter M6-large is the largest pretrained model in Chinese
- Experimental results show that our proposed M6 outperforms the baseline in a number of downstream tasks concerning both single modality and multiple modalities We will continue the pretraining of extremely large models by increasing data to explore the limit of its performance
Try using models to generate summary,it takes about 60s
Must-Reading Tree
Example
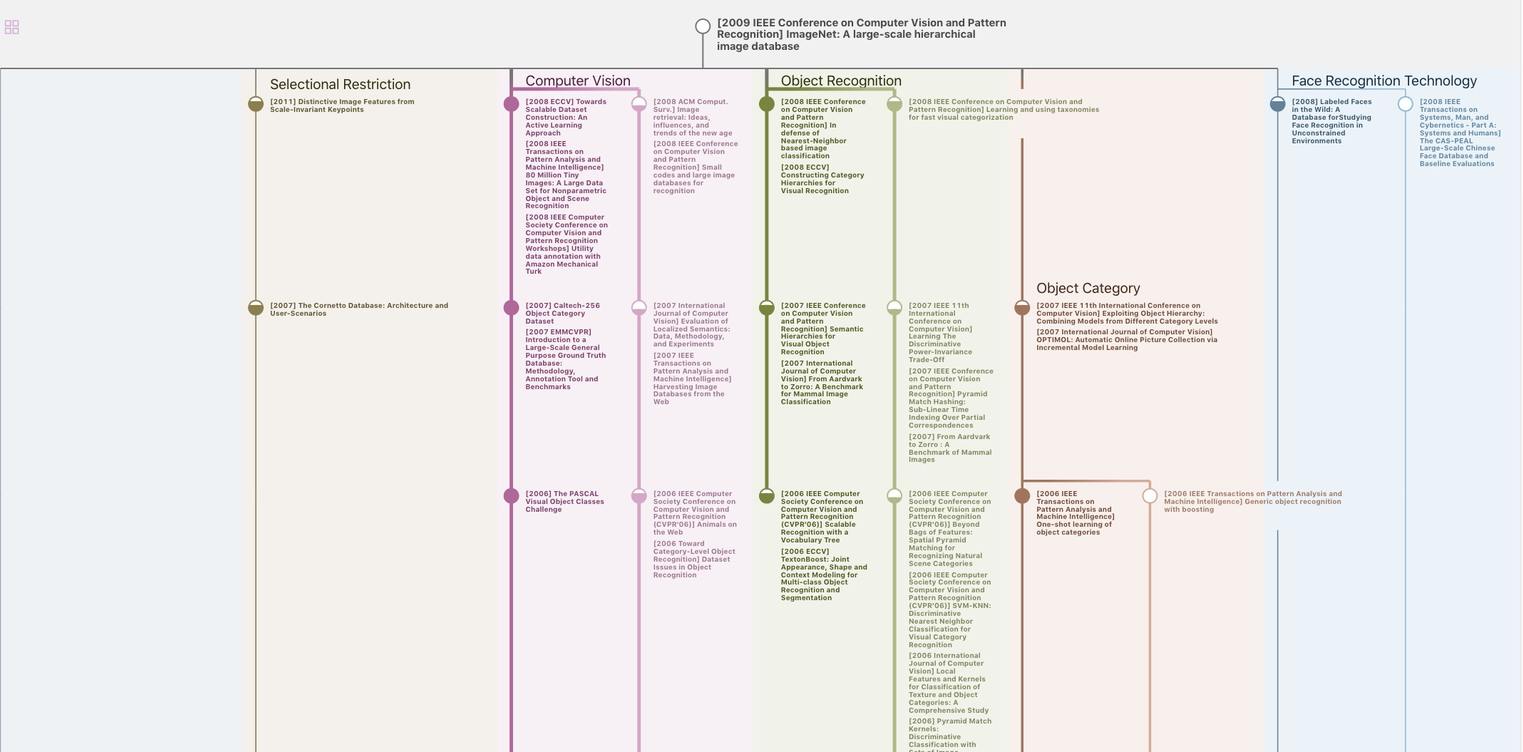
Generate MRT to find the research sequence of this paper
Related Papers
2016
被引用116 | 浏览
Data Disclaimer
The page data are from open Internet sources, cooperative publishers and automatic analysis results through AI technology. We do not make any commitments and guarantees for the validity, accuracy, correctness, reliability, completeness and timeliness of the page data. If you have any questions, please contact us by email: report@aminer.cn
Chat Paper
去 AI 文献库 对话