Downscaled XCO2 Estimation Using Data Fusion and AI-based Spatio-Temporal Models
IEEE GEOSCIENCE AND REMOTE SENSING LETTERS(2024)
关键词
Data resampling,downscaling,gap-filling,interpolation,kriging,land cover,orbiting carbon observatory-2 (OCO-2),open-source data inventory for anthropogenic CO2 (ODIAC),regressors,SIFoco2_005,XCO2
AI 理解论文
溯源树
样例
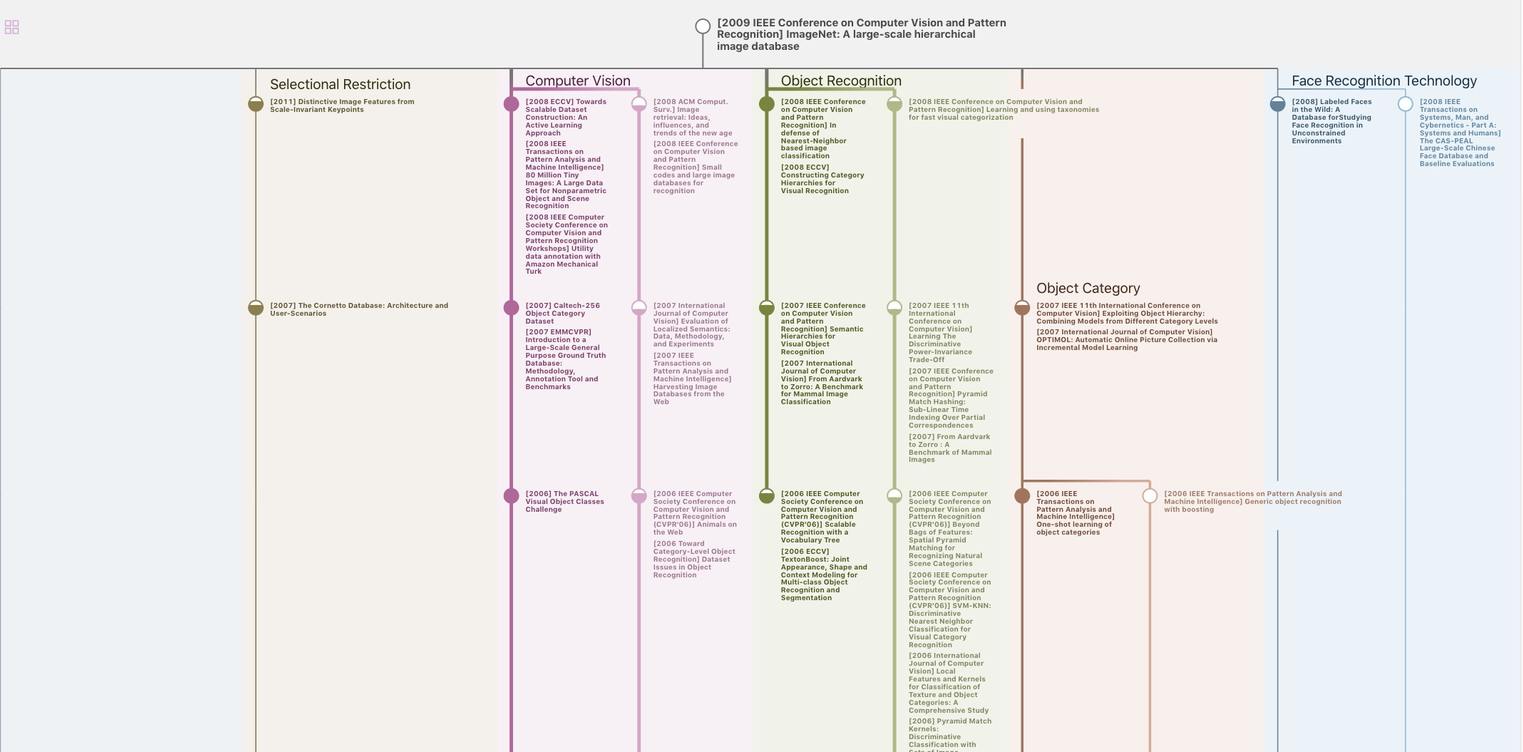
生成溯源树,研究论文发展脉络
Chat Paper
正在生成论文摘要