A Novel Stacking-Based Predictor for Accurate Prediction of Antimicrobial Peptides.
Journal of biomolecular structure and dynamics/Journal of biomolecular structure & dynamics(2024)
摘要
Antimicrobial peptides (AMPs) are gaining acceptance and support as a chief antibiotic substitute since they boost human immunity. They retain a wide range of actions and have a low risk of developing resistance, which are critical properties to the pharmaceutical industry for drug discovery. Antibiotic sensitivity, however, is an issue that affects people all around the world and has the potential to one day lead to an epidemic. As cutting-edge therapeutic agents, AMPs are also expected to cure microbial infections. In order to produce tolerable drugs, it is crucial to understand the significance of the basic architecture of AMPs. Traditional laboratory methods are expensive and time-consuming for AMPs testing and detection. Currently, bioinformatics techniques are being successfully applied to the detection of AMPs. In this study, we have developed a novel STacking-based ensemble learning framework for AntiMicrobial Peptide (STAMP) prediction. First, we constructed 84 different baseline models by using 12 different feature encoding schemes and 7 popular machine learning algorithms. Second, these baseline models were trained and employed to create a new probabilistic feature vector. Finally, based on the feature selection strategy, we determined the optimal probabilistic feature vector, which was further utilized for the construction of our stacked model. Resultantly, the STAMP predictor achieved excellent performance during cross-validation with an accuracy and Matthew's correlation coefficient of 0.930 and 0.860, respectively. The corresponding metrics during the independent test were 0.710 and 0.464, respectively. Overall, STAMP achieved a more accurate and stable performance than the baseline models and significantly outperformed the existing predictors, demonstrating the effectiveness of our proposed hybrid framework. Furthermore, STAMP is expected to assist community-wide efforts in identifying AMPs and will contribute to the development of novel therapeutic methods and drug-design for immunity.Communicated by Ramaswamy H. Sarma.
更多查看译文
关键词
Antimicrobial peptides,bioinformatics,feature representation,learning algorithms,classification,sequence-based models
AI 理解论文
溯源树
样例
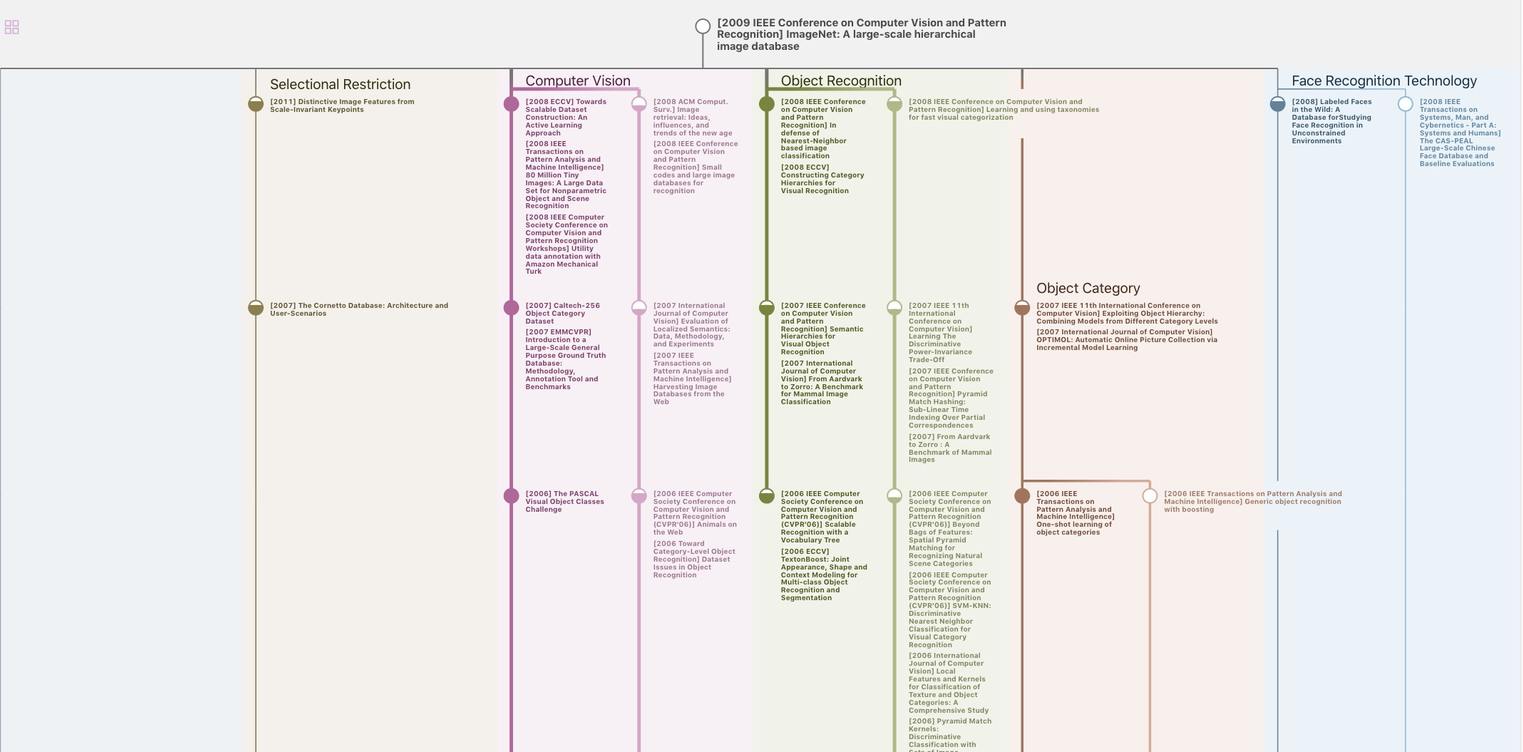
生成溯源树,研究论文发展脉络
Chat Paper
正在生成论文摘要