Revisiting the Equivalence of In-Context Learning and Gradient Descent: The Impact of Data Distribution
ICASSP 2024 - 2024 IEEE International Conference on Acoustics, Speech and Signal Processing (ICASSP)(2024)
摘要
Transformers exhibit in-context learning (ICL), enabling adaptation to various tasks via prompts without the need for computationally intensive fine-tuning. Recent research investigates ICL’s mechanisms under analytically tractable models, with some conjecturing that ICL with linear attention implements one step of gradient descent for simple linear regression tasks. This paper reevaluates this claim, revealing it relies on strong assumptions like feature independence. Relaxing these assumptions, we prove that ICL with linear attention resembles preconditioned gradient descent, with a pre-conditioner that depends on the data covariance. Our experiments support this finding. We also empirically explore softmax-attention and find that increasing the number of attention heads better approximates gradient descent. Our work offers a nuanced perspective on the connection between ICL and gradient descent, emphasizing data assumptions.
更多查看译文
关键词
In-Context Learning,Transformers,Linear Attention,Deep Learning
AI 理解论文
溯源树
样例
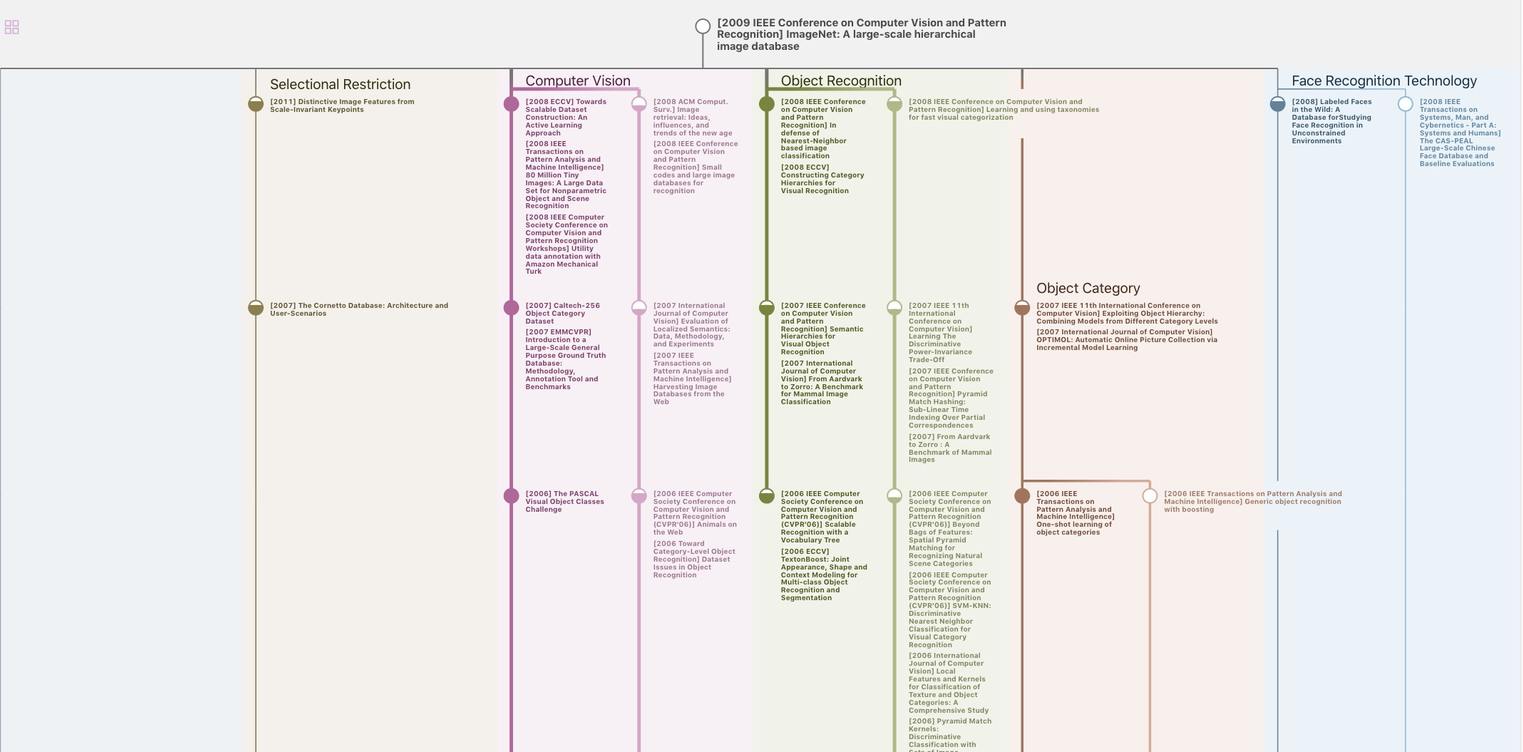
生成溯源树,研究论文发展脉络
Chat Paper
正在生成论文摘要