Image Mixing and Gradient Smoothing to Enhance the SAR Image Attack Transferability.
IEEE International Conference on Acoustics, Speech, and Signal Processing(2024)
摘要
Deep Neural Networks (DNNs) are known to be vulnerable to adversarial examples, which are crafted by adding imperceptible perturbations to clean examples. With the wide applications of DNNs to Synthetic Aperture Radar (SAR) Automatic Target Recognition (ATR), the vulnerability of SAR deep recognition models has attracted increasing attention. Existing works show that input transformation can effectively improve the black-box attack performance of adversarial examples, but there is little work in the field of SAR-ATR. In this paper, we propose a novel input transformation attack called Image Mixing and Gradient Smoothing (IMGS), which is dedicated to attacking SAR images. IMGS mixes a small portion of another image into the input samples in amplitude and phase with different rates and uses the Local Mean Square Error (LMSE) filter to smooth the gradient. Extensive experiments conducted on the MSTAR dataset demonstrate that IMGS significantly outperforms other input transformation methods, originally designed for attacking visual images, in both white-box and black-box settings. The code is available at https://github.com/JHL-HUST/IMGS.
更多查看译文
关键词
SAR image classification,black-box attack,input transformation,adversarial transferability
AI 理解论文
溯源树
样例
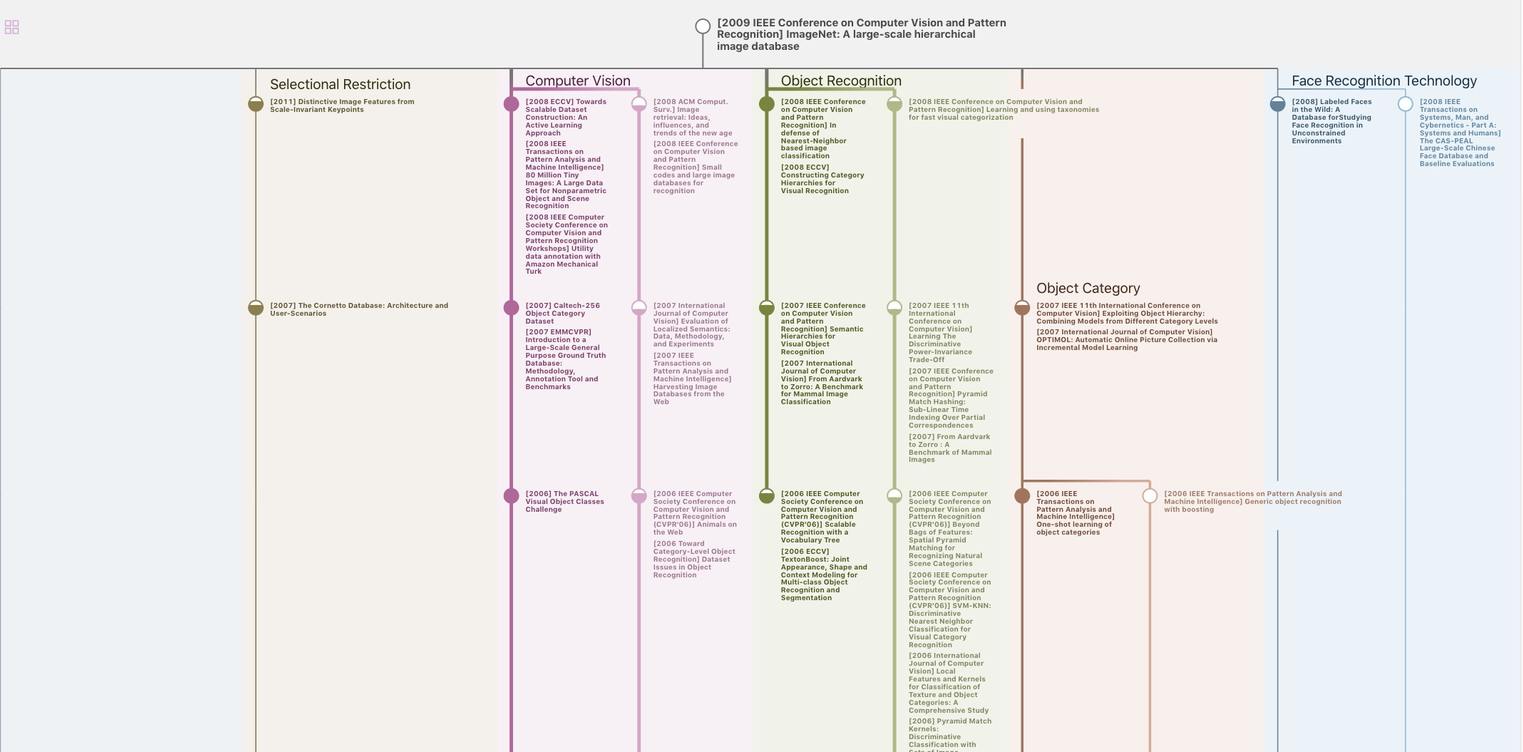
生成溯源树,研究论文发展脉络
Chat Paper
正在生成论文摘要