An Efficient Transformer For Demosaicing Via Compressed Multi-Branch Attention Mechanism.
IEEE International Conference on Acoustics, Speech, and Signal Processing(2024)
摘要
Recent demosaicing approaches are not effective and efficient enough as they do not make full use of these two factors: (1) Capturing long-range spatial dependencies effiently. (2) Reducing the computational costs when utilizing channel attention. To take them into consideration, we propose an Efficient Compressed Multibranch Transformer (ECMT) to handle demosaicing problem on various color filter arrays (CFA). There are two key specifically designed components: First, a Local-Global Spatial-wise Multi-head Self-Attention (LGSMSA) captures both spatial long-range dependencies and local context details by an efficient manner. Second, an Inner-Compressed Channel-wise Multi-head Self-Attention (ICCMSA) clusters closely related channels into the same group and then calculates channel-wise MSA within each group to reduce the computational cost. Experimental results on both real and synthetic datasets show that our ECMT achieves promising reconstruction quality in Bayer and QuadBayer CFA while requiring cheaper computational, memory costs and less inference time compared to state-of-the-art methods.
更多查看译文
关键词
Demosaicing,Efficient Transformer,Image Signal Processing
AI 理解论文
溯源树
样例
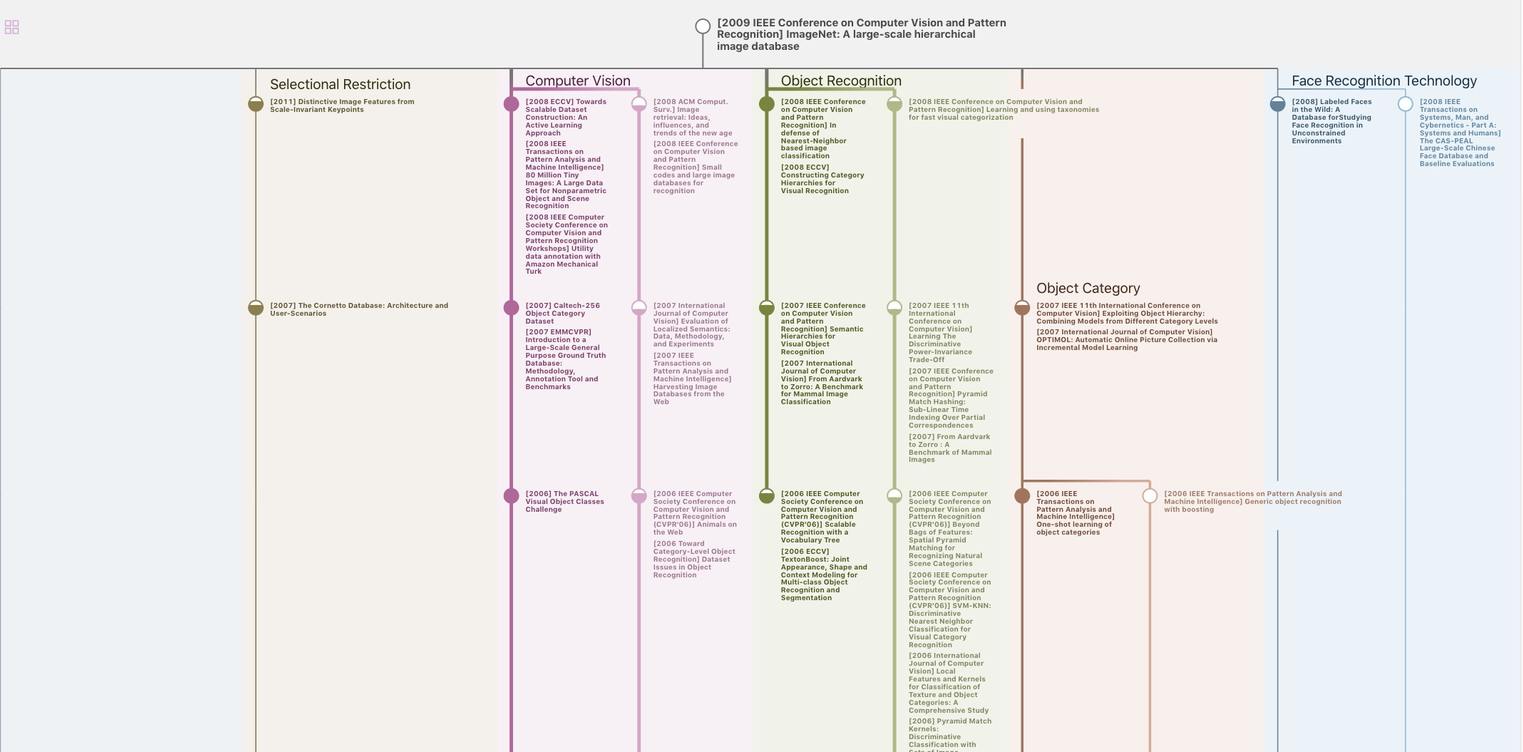
生成溯源树,研究论文发展脉络
Chat Paper
正在生成论文摘要