Delving Deeper Into Vulnerable Samples in Adversarial Training.
IEEE International Conference on Acoustics, Speech, and Signal Processing(2024)
摘要
Recently, vulnerable samples have been shown to be crucial for improving adversarial training performance. Our analysis on existing vulnerable samples mining methods indicate that existing methods have two problems: 1) valuable connections among different pairs of natural samples and their adversarial counterparts are ignored; 2) parts of vulnerable samples are unconsidered. To better leverage vulnerable samples, we propose INter PAir ConstrainT (INPACT) and Vulnerable Aware adveRsarial Training (VART) to address these drawbacks respectively. INPACT assesses adversarial risk with more comprehensive regularization on sample relationships, which takes both inter and intra connections of natural/adversarial sample pairs into consideration. Meanwhile VART makes full use of all vulnerable samples, including notable proportion neglected by existing instance re-weighting strategies. Extensive experiments on different datasets and backbones demonstrate the effectiveness of the proposed method.
更多查看译文
关键词
Adversarial training,Vulunerable samples
AI 理解论文
溯源树
样例
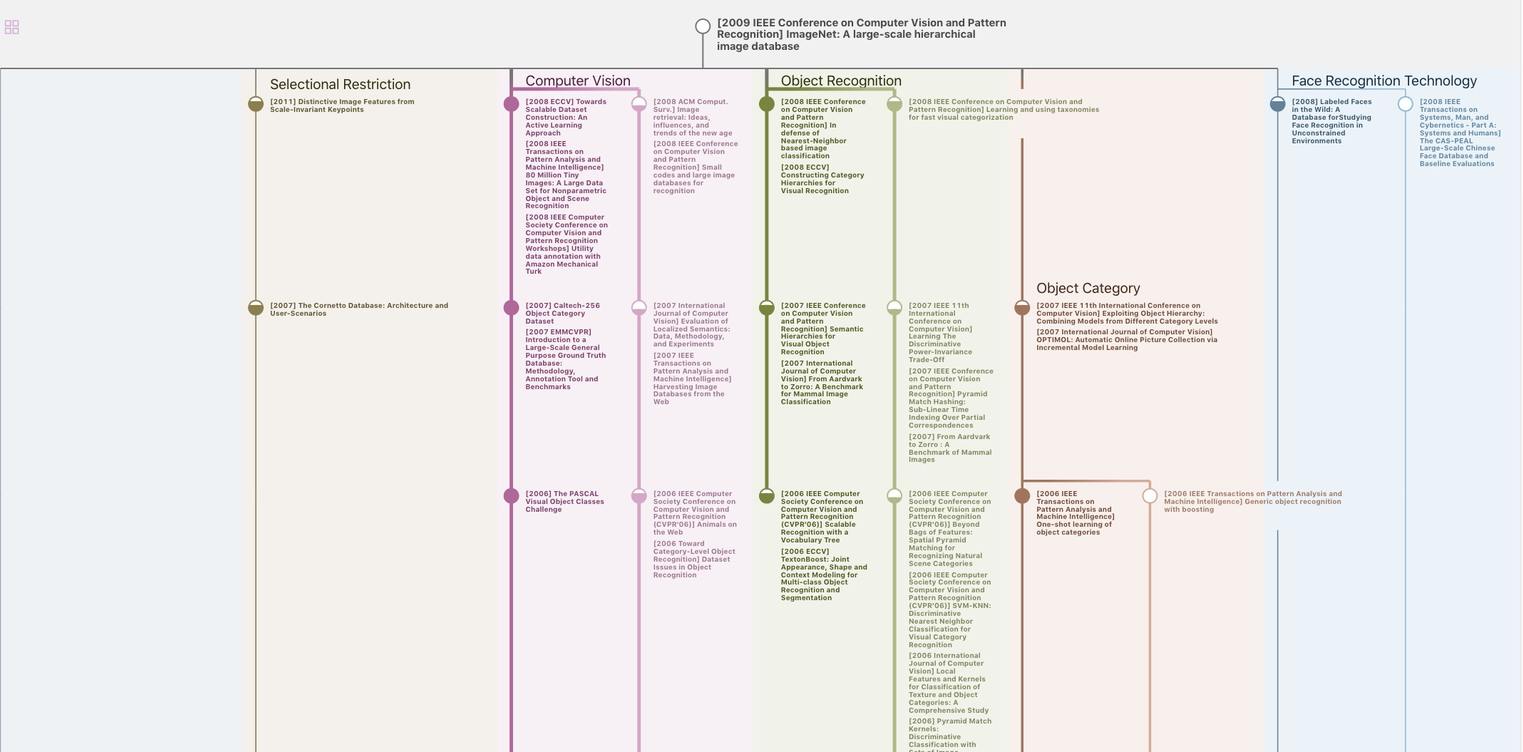
生成溯源树,研究论文发展脉络
Chat Paper
正在生成论文摘要