Multi-Agent Exploration Via Self-Learning and Social Learning
IEEE International Conference on Acoustics, Speech, and Signal Processing(2024)
摘要
Self-learning and social learning stand as two pivotal constituents in multi-agent exploration. Inspired by the fact that animals and humans explore unfamiliar environments to learn survival skills by training themselves using unlabeled data and replicating others' successful experiences, we propose a multi-agent reinforcement learning method, named Self-Learning and Social Learning (S 2 L), which aims to address the complex tasks caused by sparse rewards and intricate sequential structures. Specifically, in Self-Learning, we incorporate both task-specific and task-agnostic intrinsic rewards. These incentives steer individual agents towards exploration and comprehension of the environment. Furthermore, in Social Learning, different independent agents can implicitly share the successful experience by observing others in view and without additional communication or parameter-sharing overhead. Finally, experimental evaluation of S 2 L on the complex task characterized by sparse rewards and intricate sequential structures demonstrates its superior performance against other competing exploration baselines.
更多查看译文
AI 理解论文
溯源树
样例
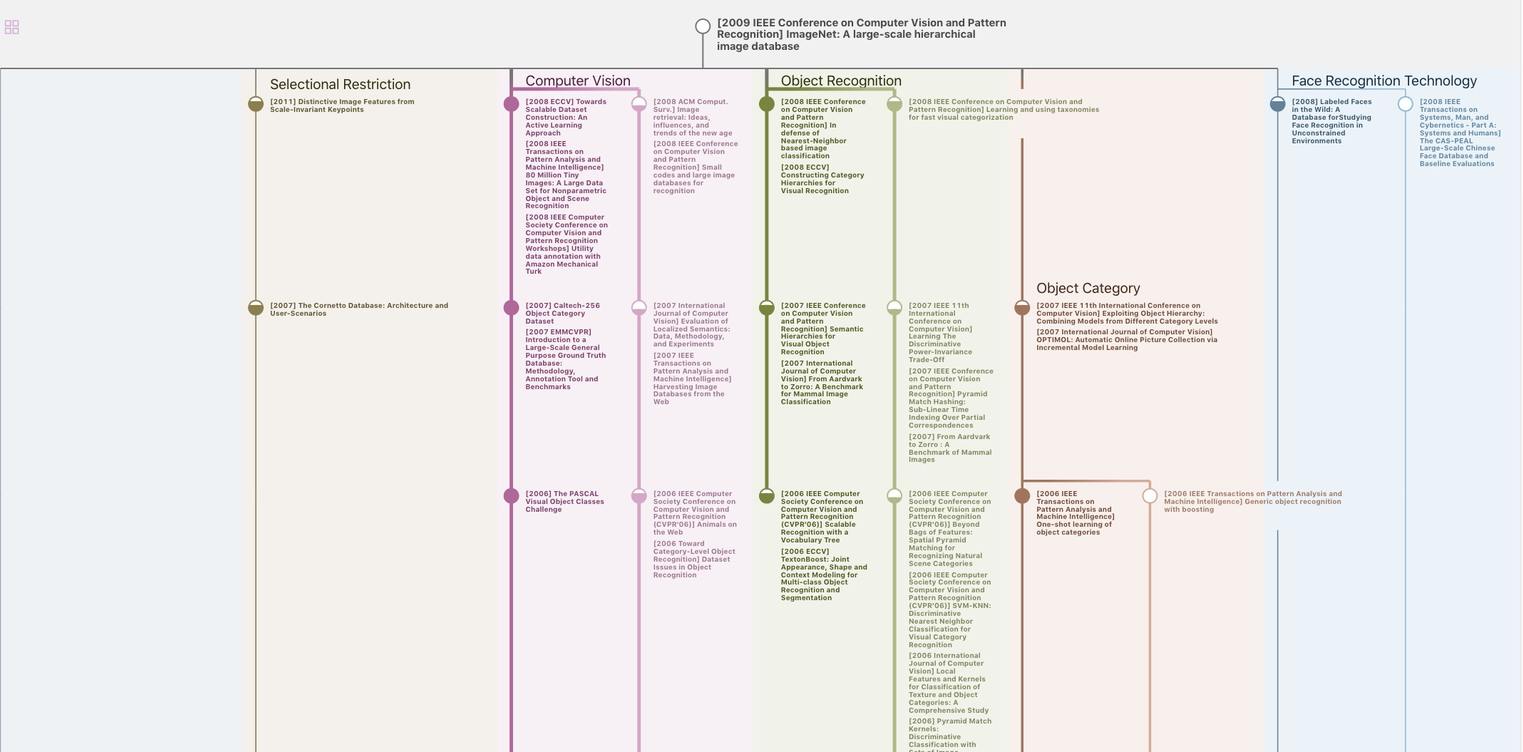
生成溯源树,研究论文发展脉络
Chat Paper
正在生成论文摘要