Skip-Step Contrastive Predictive Coding for Time Series Anomaly Detection
ICASSP 2024 - 2024 IEEE International Conference on Acoustics, Speech and Signal Processing (ICASSP)(2024)
摘要
Self-supervised learning (SSL) shows impressive performance in many tasks lacking sufficient labels. In this paper, we study SSL in time series anomaly detection (TSAD) by incorporating the characteristics of time series data. Specifically, we build an anomaly detection algorithm consisting of global pattern learning and local association learning. The global pattern learning module builds encoder and decoder to reconstruct the raw time series data to detect global anomalies. To complement the limitation of the global pattern learning that ignores local associations between anomaly points and their adjacent windows, we design a local association learning module, which leverages contrastive predictive coding (CPC) to transform the identification of anomaly points into positive pairs identification. Motivated by the observation that adjusting the distance between the history window and the time point to be detected directly impacts the detection performance in the CPC framework, we further propose a skip-step CPC scheme in the local association learning module which adjusts the distance for better construction of the positive pairs and detection results. The experimental results show that the proposed algorithm achieves superior performance on SMD and PSM datasets in comparison with 12 state-of-the-art algorithms.
更多查看译文
关键词
Time Series,Anomaly Detection,Self-supervised Learning
AI 理解论文
溯源树
样例
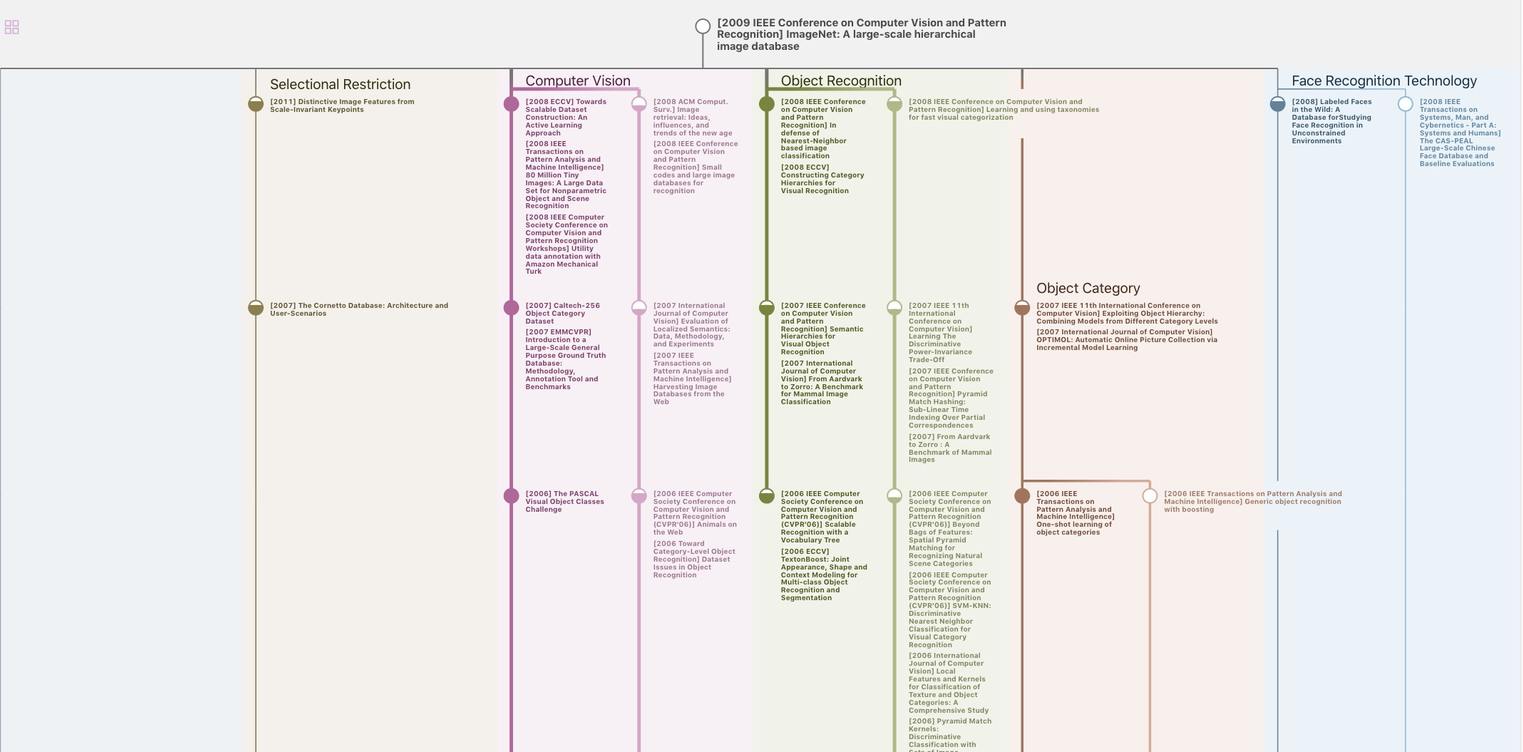
生成溯源树,研究论文发展脉络
Chat Paper
正在生成论文摘要