MSFR: Stance Detection Based on Multi-Aspect Semantic Feature Representation via Hierarchical Contrastive Learning
ICASSP 2024 - 2024 IEEE International Conference on Acoustics, Speech and Signal Processing (ICASSP)(2024)
Abstract
Zero-shot stance detection aims to determine the stance of previously unseen targets during the inference phase. Achieving effective feature alignment from seen targets to unseen targets is crucial for zero-shot stance detection. In this paper, we propose MSFR, a hierarchical contrastive learning framework, which consists of two core components: inter-aspect contrastive learning for distinguishing aspect-level features and intra-aspect contrastive learning for capturing attribute-level features. Specifically, inter-aspect contrastive learning first maps the global features of an utterance to multiple aspects that influence semantic expression (referred to as aspect-level feature differentiation). This process facilitates the alignment of semantic features across different factors of seen and unseen targets. Intra-aspect contrastive learning enhances the distinguishability of features within the same aspect (referred to as attribute-level feature differentiation) and improves the model’s fine-grained generalization capability. Experimental results demonstrate the superior performance of our model compared to competing baseline models.
MoreTranslated text
Key words
Stance Detection,Hierarchical Contrastive Learning,Multi-Aspect Semantic Feature
AI Read Science
Must-Reading Tree
Example
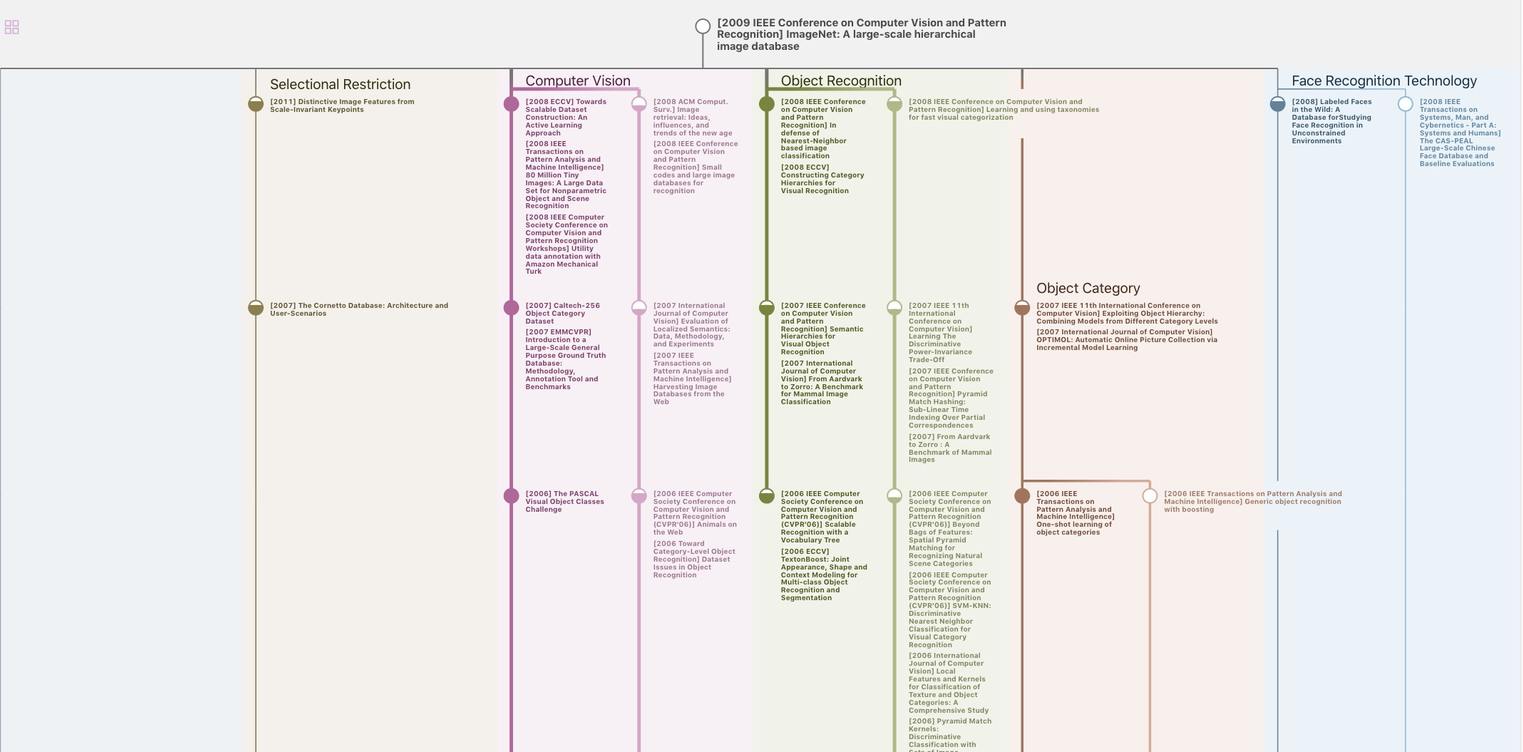
Generate MRT to find the research sequence of this paper
Chat Paper
Summary is being generated by the instructions you defined