Axis Order Invariance Learned from Point Clouds
ICASSP 2024 - 2024 IEEE International Conference on Acoustics, Speech and Signal Processing (ICASSP)(2024)
摘要
Point-based models have achieved impressive success in point cloud processing, but current methods mainly address issues such as disorder, sparsity, and rotation of point clouds. In addition to these characteristics, we also found that for the point set P (X
i
, Y
i
, Z
i
) of a point cloud, any order of exchanging coordinate axes, such as P (Z
i
, Y
i
, X
i
), still yields a reasonable point cloud. At present, point-based models only handle point clouds in the X-Y-Z coordinate order, while the classification accuracy of point clouds in the Z-Y-X coordinate order is extremely low, even dropping to 10%, greatly reducing the recognition ability of the model. To learn the invariance of the coordinate axis order of the base model, we first designed an axis-order augmentation algorithm, which increases data diversity by shuffling the coordinate axis order of the point cloud; Secondly, we designed an axis-order-invariant module that can learn the invariance of coordinate axis order from the data. Numerous experiments have shown that our proposed method can greatly improve the classification accuracy of the base model for point clouds with different coordinate axis orders.
更多查看译文
关键词
Point Cloud,Axis Order Invariance
AI 理解论文
溯源树
样例
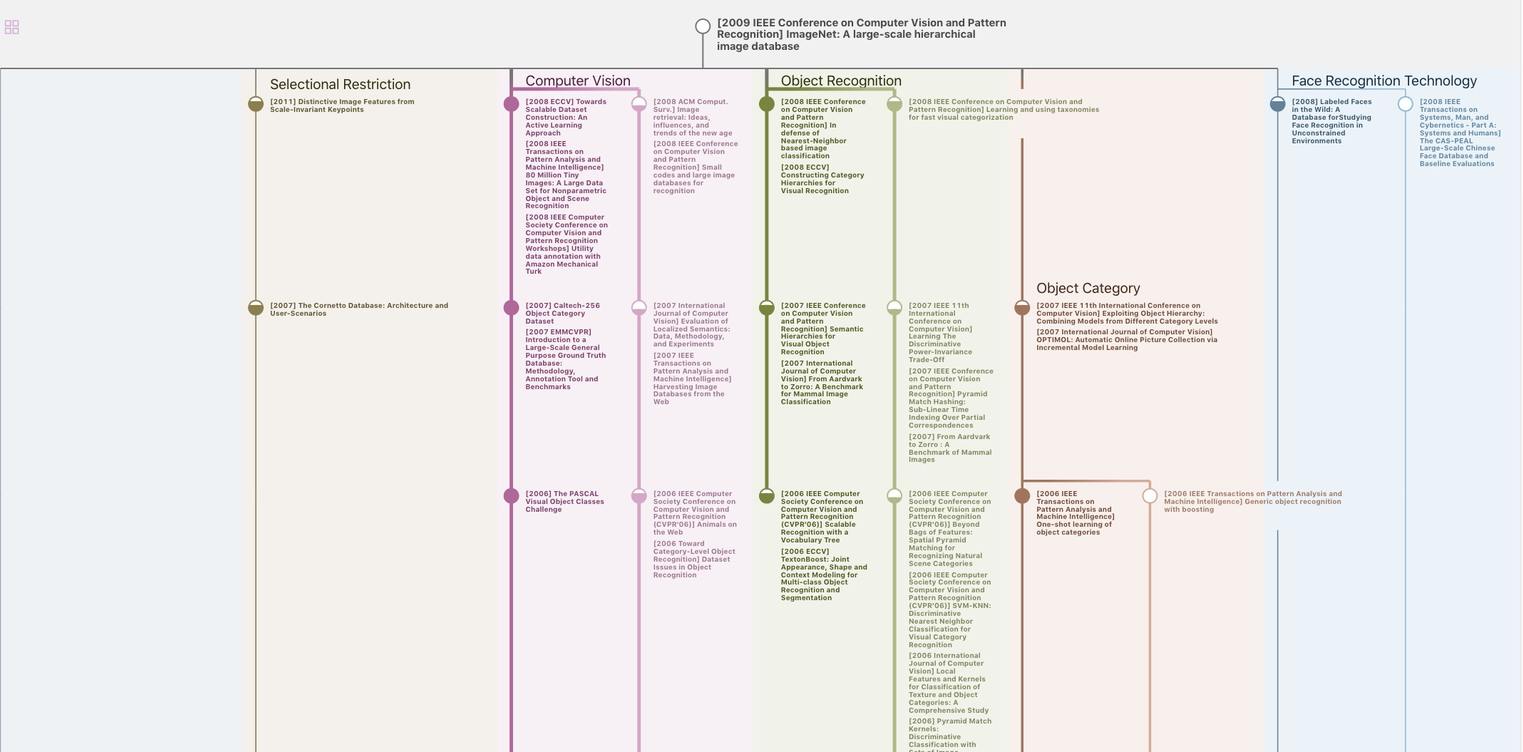
生成溯源树,研究论文发展脉络
Chat Paper
正在生成论文摘要