Improving Acoustic Echo Cancellation for Voice Assistants Using Neural Echo Suppression and Multi-Microphone Noise Reduction
IEEE International Conference on Acoustics, Speech, and Signal Processing(2024)
关键词
aec,speech enhancement,keyword spotting,asr
AI 理解论文
溯源树
样例
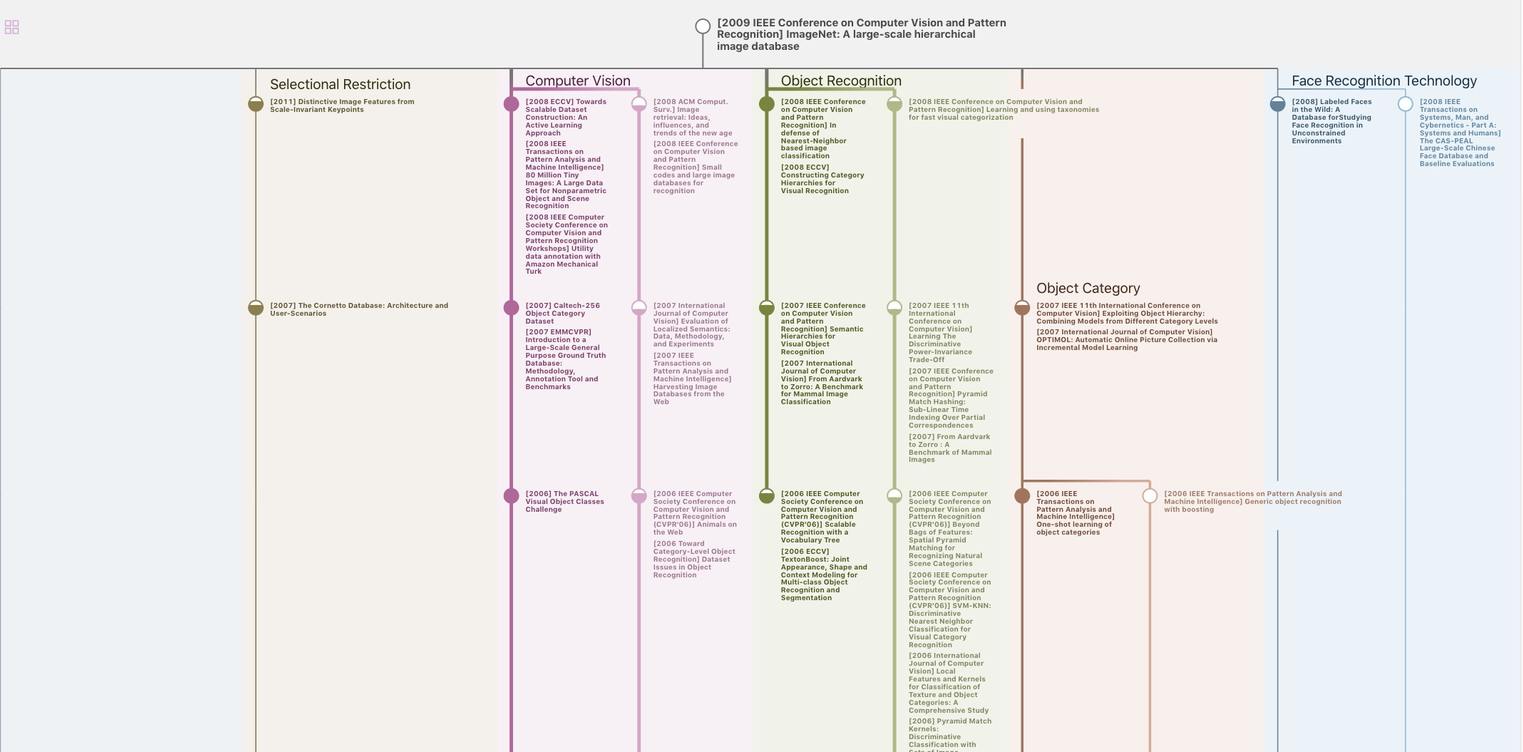
生成溯源树,研究论文发展脉络
Chat Paper
正在生成论文摘要