Inter-individual and inter-site neural code conversion without shared stimuli
CoRR(2024)
摘要
Inter-individual variability in fine-grained functional brain organization
poses challenges for scalable data analysis and modeling. Functional alignment
techniques can help mitigate these individual differences but typically require
paired brain data with the same stimuli between individuals, which is often
unavailable. We present a neural code conversion method that overcomes this
constraint by optimizing conversion parameters based on the discrepancy between
the stimulus contents represented by original and converted brain activity
patterns. This approach, combined with hierarchical features of deep neural
networks (DNNs) as latent content representations, achieves conversion accuracy
comparable to methods using shared stimuli. The converted brain activity from a
source subject can be accurately decoded using the target's pre-trained
decoders, producing high-quality visual image reconstructions that rival
within-individual decoding, even with data across different sites and limited
training samples. Our approach offers a promising framework for scalable neural
data analysis and modeling and a foundation for brain-to-brain communication.
更多查看译文
AI 理解论文
溯源树
样例
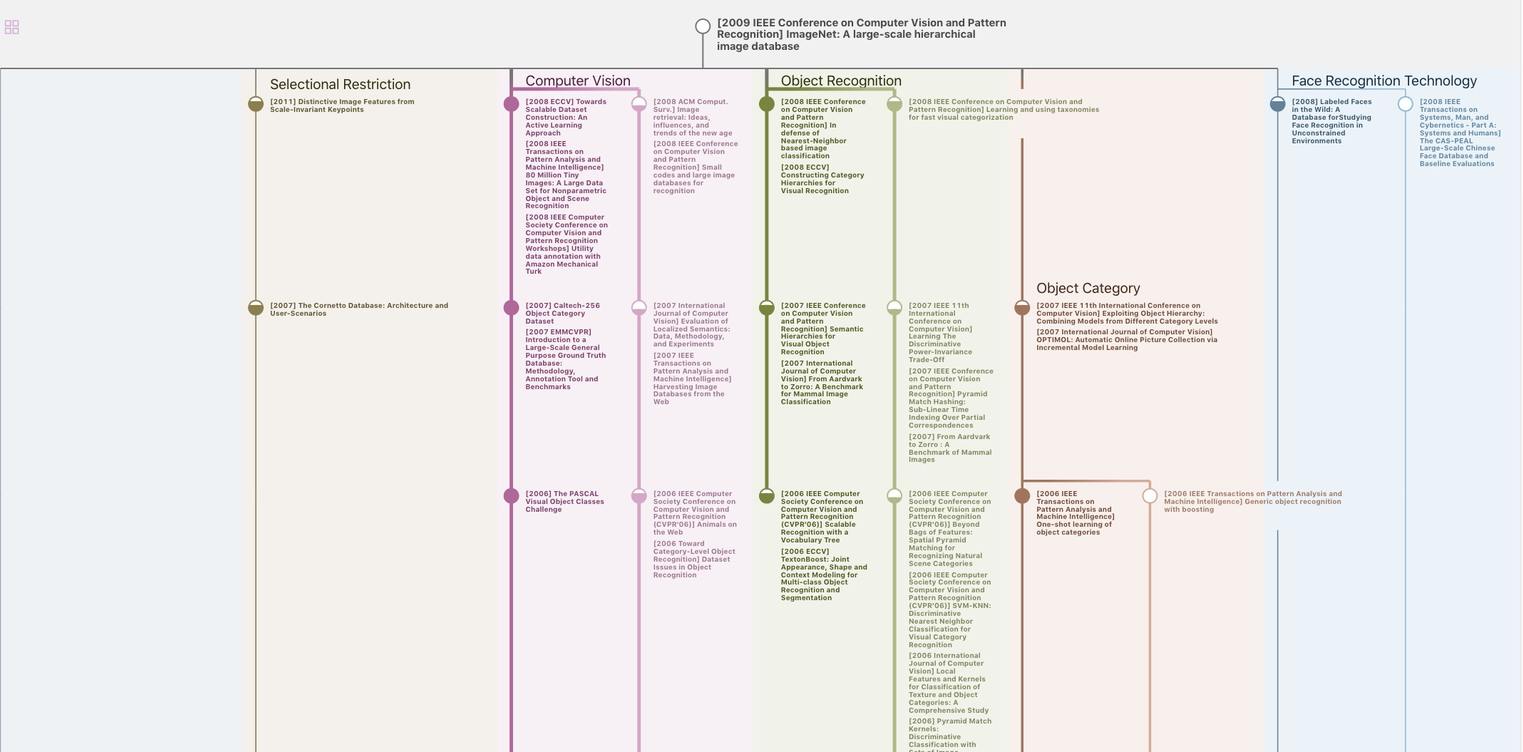
生成溯源树,研究论文发展脉络
Chat Paper
正在生成论文摘要