Gradient and Brightness Guided Low-Light Enhancement with Attention-Based Self-Paced Learning
ICASSP 2024 - 2024 IEEE International Conference on Acoustics, Speech and Signal Processing (ICASSP)(2024)
摘要
Low-light image enhancement aims to reconstruct images with insufficient illumination into visually appealing representations with natural brightness. While most existing methods tend to focus on enhancing illumination, they often overlook the restoration of finer details in the enhanced image. Moreover, these methods do not adequately address the varying degradation levels observed in different regions of the image. In this study, we present a gradient and brightness guided low-light image enhancement framework, which can simultaneously augment the detail and illumination during the enhancement process. Our approach involves extracting gradient information from gamma-corrected images, which offers a remarkable advantage in preserving edge details compared to direct extraction from degraded images. To further refine the enhancement process and adaptively adjust the difficulty of samples, thereby boosting learning efficiency, we introduce an attention-based self-paced learning strategy. This strategy assigns different gradient and brightness weights based on the degradation levels within different image regions. Extensive experiments demonstrate the superiority of our proposed method over state-of-the-art approaches. The code is available at https://github.com/MSL502/GBASPL.
更多查看译文
关键词
Low-Light Image Enhancement,Gradient Enhancement,Brightness Enhancement,Attention-based Self-paced Learning Strategy
AI 理解论文
溯源树
样例
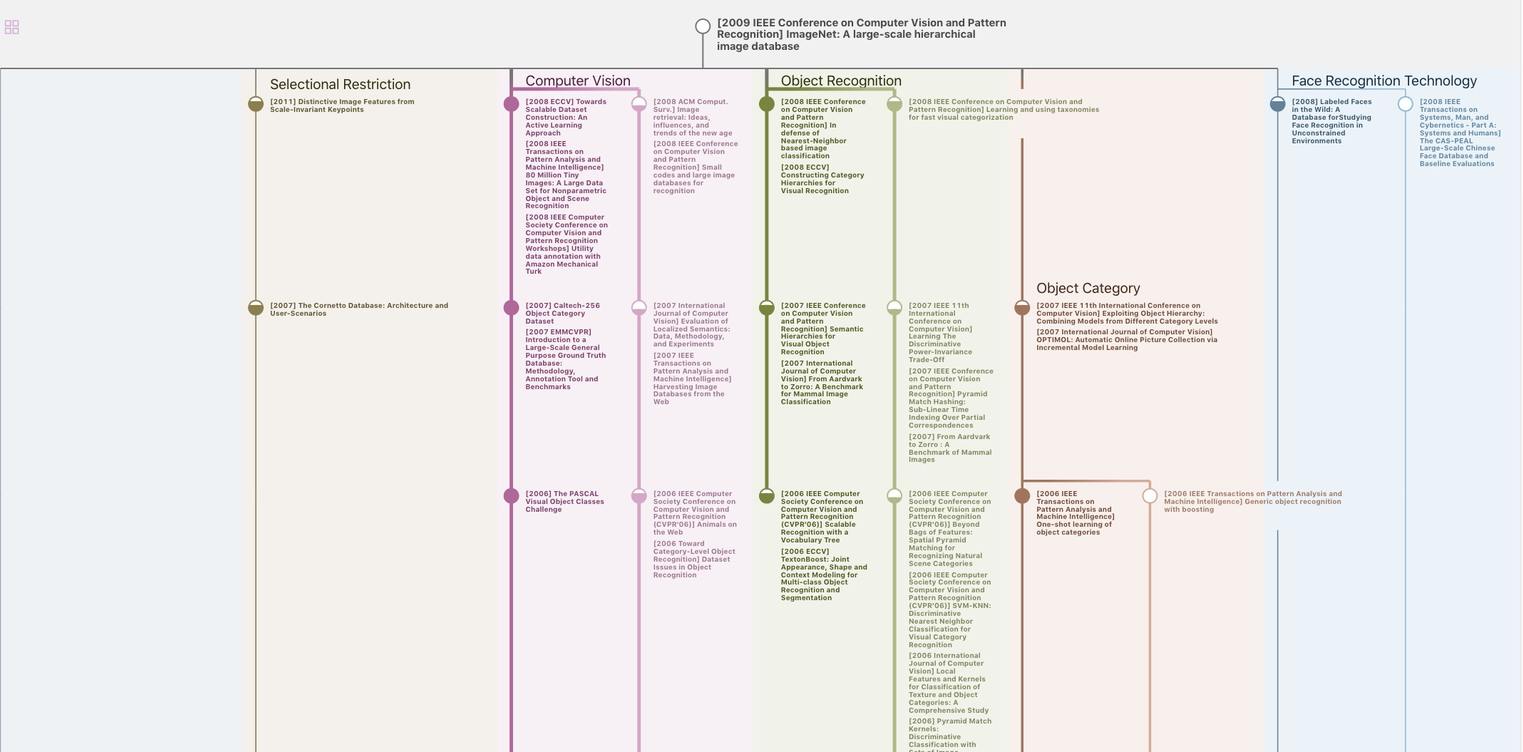
生成溯源树,研究论文发展脉络
Chat Paper
正在生成论文摘要