Robust Lightweight Depth Estimation Model Via Data-Free Distillation
IEEE International Conference on Acoustics, Speech, and Signal Processing(2024)
摘要
Existing Monocular Depth Estimation (MDE) methods often use large and complex neural networks. Despite the advanced performance of these methods, we consider the efficiency and generalization for practical applications with limited resources. In our paper, we present an efficient transformer-based monocular relative depth estimation network and train it with a diverse depth dataset to obtain good generalization performance. Knowledge distillation (KD) is employed to transfer the general knowledge from a pre-trained teacher network to the compact student network, demonstrating that KD can improve the generalization ability as well as the accuracy. Moreover, we propose a geometric label-free distillation method to improve the lightweight model in specific domains utilizing 3D geometric cues with unlabeled data. We show that our method outperforms other KD methods with or without ground truth supervision. Finally, we propose an application of the lightweight network to a two-stage depth completion task. Our method shows on par or even superior cross-domain generalization ability compared to large networks.
更多查看译文
关键词
Depth Estimation,Generalization,Knowledge Distillation,Depth Completion
AI 理解论文
溯源树
样例
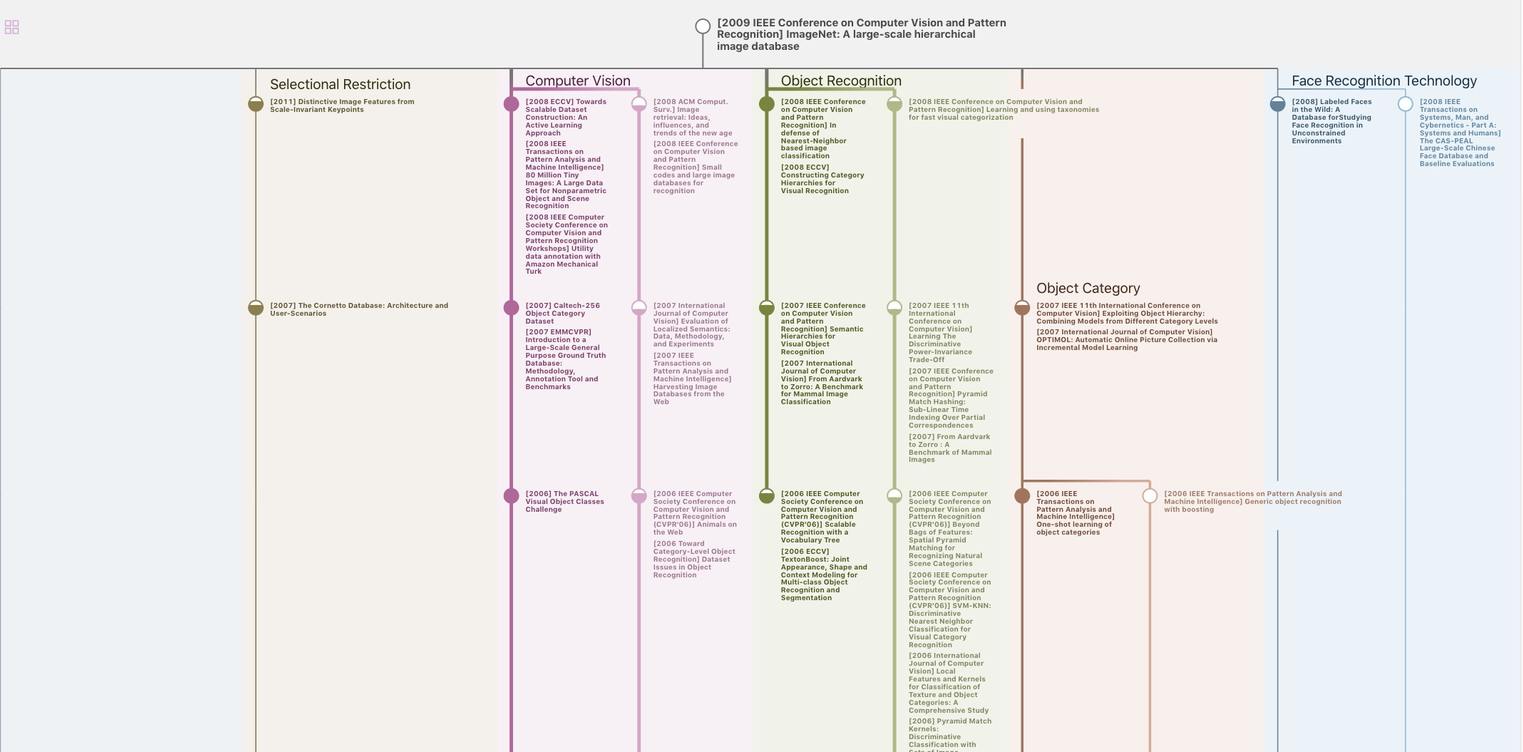
生成溯源树,研究论文发展脉络
Chat Paper
正在生成论文摘要