Fast Test Error Rates for Gradient-Based Algorithms on Separable Data
ICASSP 2024 - 2024 IEEE International Conference on Acoustics, Speech and Signal Processing (ICASSP)(2024)
摘要
In recent research aimed at understanding the strong generalization performance of simple gradient-based methods on overparameterized models, it has been demonstrated that when training a linear predictor on separable data with an exponentially-tailed loss function, the predictor converges towards the max-margin classifier direction, explaining its resistance to overfitting asymptotically. Moreover, recent findings have shown that overfitting is not a concern even in finite-time scenarios (non-asymptotically), as finite-time generalization bounds have been derived for gradient flow, gradient descent (GD), and stochastic GD. In this work, we extend this line of research and obtain new finite-time generalization bounds for other popular first-order methods, namely normalized GD and Nesterov’s accelerated GD. Our results reveal that these methods, as they converge more rapidly in terms of training loss, also exhibit enhanced generalization performance in terms of test error.
更多查看译文
关键词
Max-margin,gradient descent,generalization bounds,implicit bias
AI 理解论文
溯源树
样例
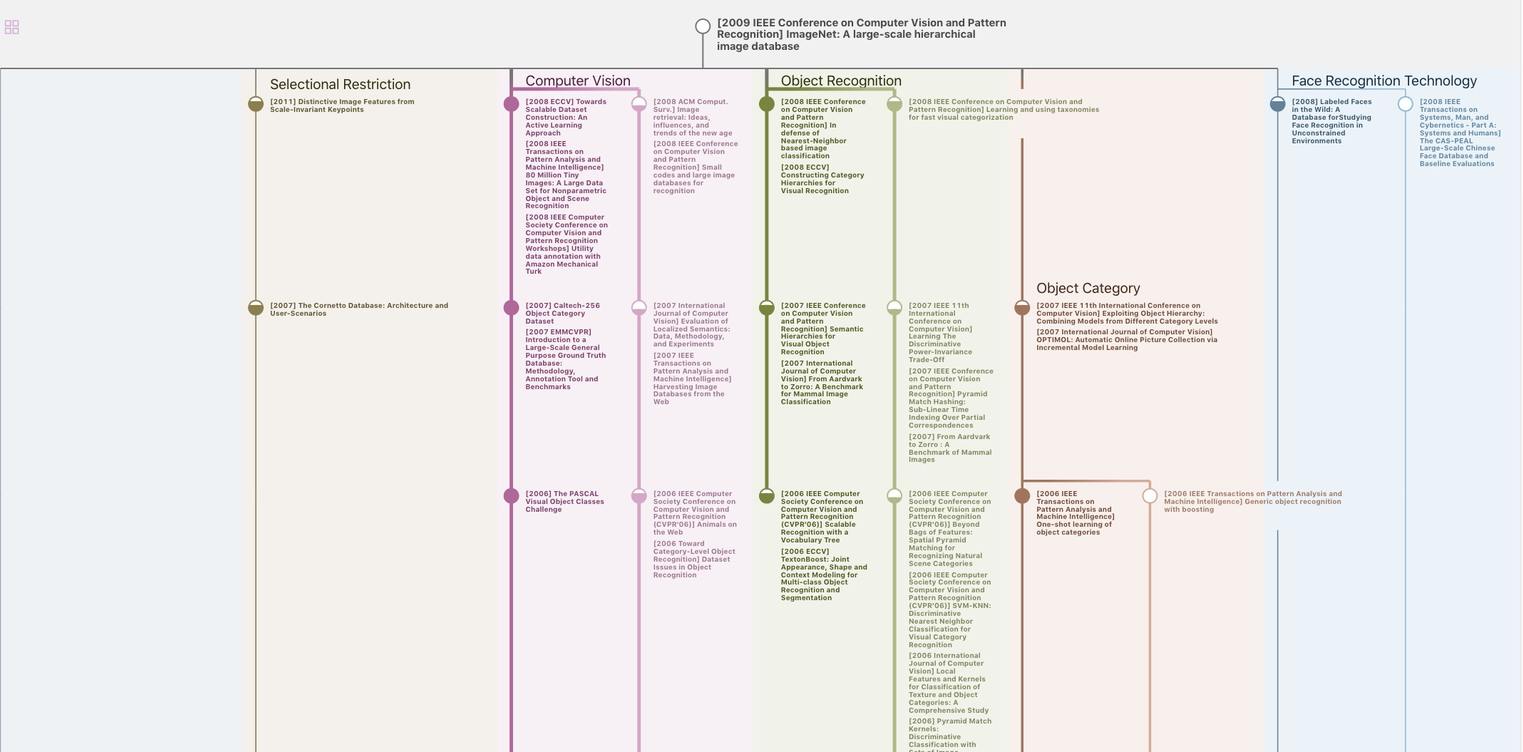
生成溯源树,研究论文发展脉络
Chat Paper
正在生成论文摘要