On the Low-Shot Transferability of [V]-Mamba
arXiv (Cornell University)(2024)
摘要
The strength of modern large-scale neural networks lies in their ability toefficiently adapt to new tasks with few examples. Although extensive researchhas investigated the transferability of Vision Transformers (ViTs) to variousdownstream tasks under diverse constraints, this study shifts focus to explorethe transfer learning potential of [V]-Mamba. We compare its performance withViTs across different few-shot data budgets and efficient transfer methods. Ouranalysis yields three key insights into [V]-Mamba's few-shot transferperformance: (a) [V]-Mamba demonstrates superior or equivalent few-shotlearning capabilities compared to ViTs when utilizing linear probing (LP) fortransfer, (b) Conversely, [V]-Mamba exhibits weaker or similar few-shotlearning performance compared to ViTs when employing visual prompting (VP) asthe transfer method, and (c) We observe a weak positive correlation between theperformance gap in transfer via LP and VP and the scale of the [V]-Mamba model.This preliminary analysis lays the foundation for more comprehensive studiesaimed at furthering our understanding of the capabilities of [V]-Mamba variantsand their distinctions from ViTs.
更多查看译文
AI 理解论文
溯源树
样例
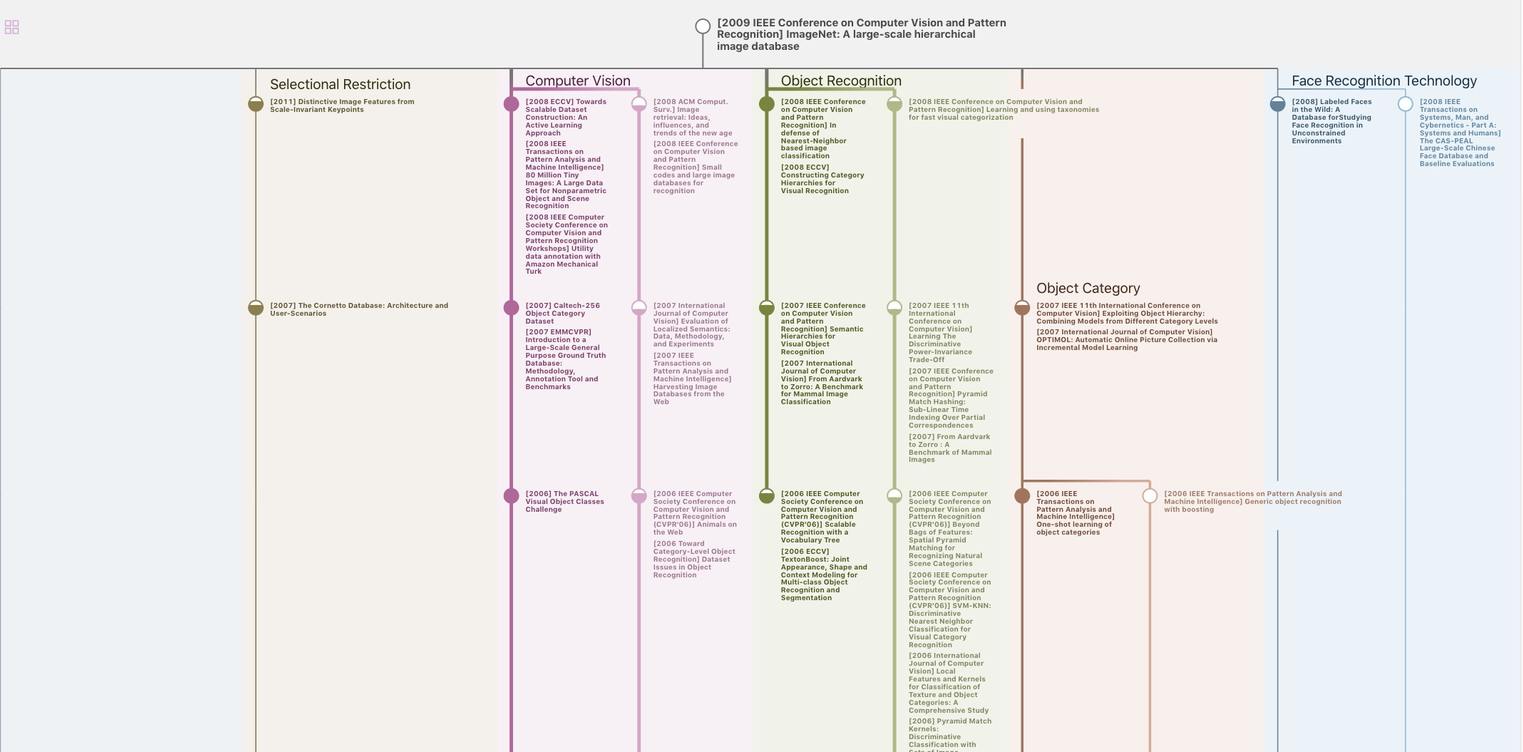
生成溯源树,研究论文发展脉络
Chat Paper
正在生成论文摘要