SF-MMCN: A Low Power Re-configurable Server Flow Convolution Neural Network Accelerator
arXiv (Cornell University)(2024)
摘要
Convolution Neural Network (CNN) accelerators have been developed rapidly inrecent studies. There are lots of CNN accelerators equipped with a variety offunction and algorithm which results in low power and high-speed performances.However, the scale of a PE array in traditional CNN accelerators is too big,which costs the most energy consumption while conducting multiply andaccumulation (MAC) computations. The other issue is that due to the advance ofCNN models, there are enormous models consist of parallel structures such asresidual block in Residual Network (ResNet). The appearance of parallelstructure in CNN models gives a challenge to the design of CNN acceleratorsowing to impacts on both operation and area efficiency. This study proposedSF-MMCN structure. The scale of PE array in proposed designs is reduced bypipeline technique in a PE. Proposed SF structure successfully make proposedSF-MMCN operate in high efficiency when facing parallel structures in CNNmodels. Proposed design is implemented with TSMC 90nm technology on VGG-16 andResNet-18 environments. The performance of proposed design achieves 76saving, 55and 4.92 times respectively.
更多查看译文
关键词
Transfer Learning
AI 理解论文
溯源树
样例
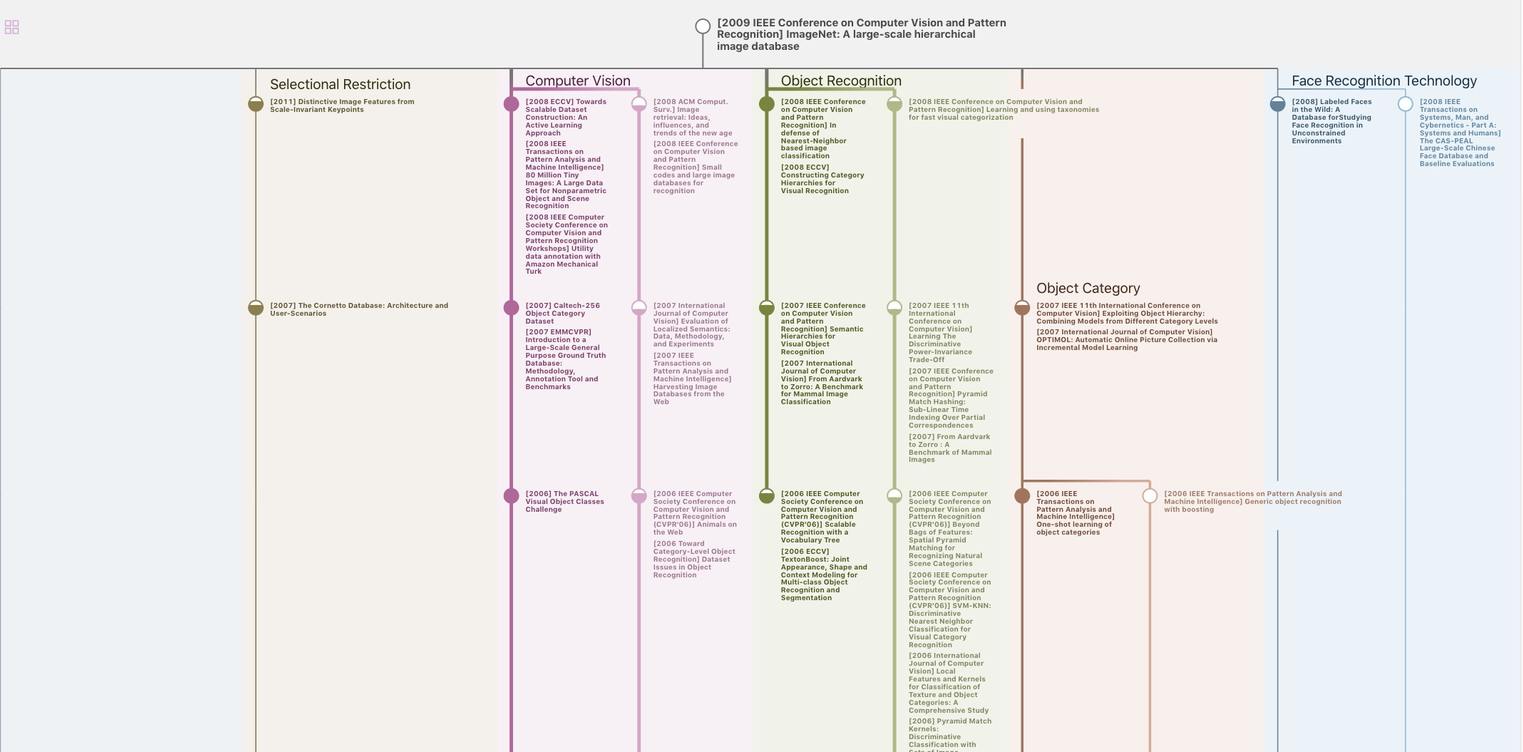
生成溯源树,研究论文发展脉络
Chat Paper
正在生成论文摘要