Stealthy Backdoor Attack Towards Federated Automatic Speaker Verification
ICASSP 2024 - 2024 IEEE International Conference on Acoustics, Speech and Signal Processing (ICASSP)(2024)
摘要
Automatic speech verification (ASV) authenticates individuals based on distinct vocal patterns, playing a pivotal role in many applications such as voice-based unlocking systems for devices. The ASV system comprises three stages: training, registration, and validation. The model refines using voice data in training, extracts vocal features in registration, and contrasts these with speech patterns in validation. Modern ASV models, primarily grounded in DNN architectures, require extensive data for training. Federated learning (FL) fosters model-sharing across multiple clients while ensuring data privacy. Due to its open architecture, FL is vulnerable to backdoor attacks. However, training a stealthy backdoor attack in FL presents challenges, including diminished attack generalization owing to data heterogeneity, and conspicuous triggers that render them easily detectable. In this paper, we propose a Federated Stealthy Backdoor Attack method ($FedSBA$). FedSBA aims to improve the attack model’s generalization, enhance its persistence, and elude anomaly detection under the heterogeneous data distribution. FedSBA constructs an attack model based on a personalized transformer and encompasses a stealthy trigger. Moreover, we also propose a defensive strategy that utilizes an adaptive weight aggregation scheme. The stealthiness and effectiveness of FedSBA are demonstrated by exhibiting superior performance in comparison to previous works.
更多查看译文
关键词
Speaker Verification,Backdoor Attacks,Federated Learning
AI 理解论文
溯源树
样例
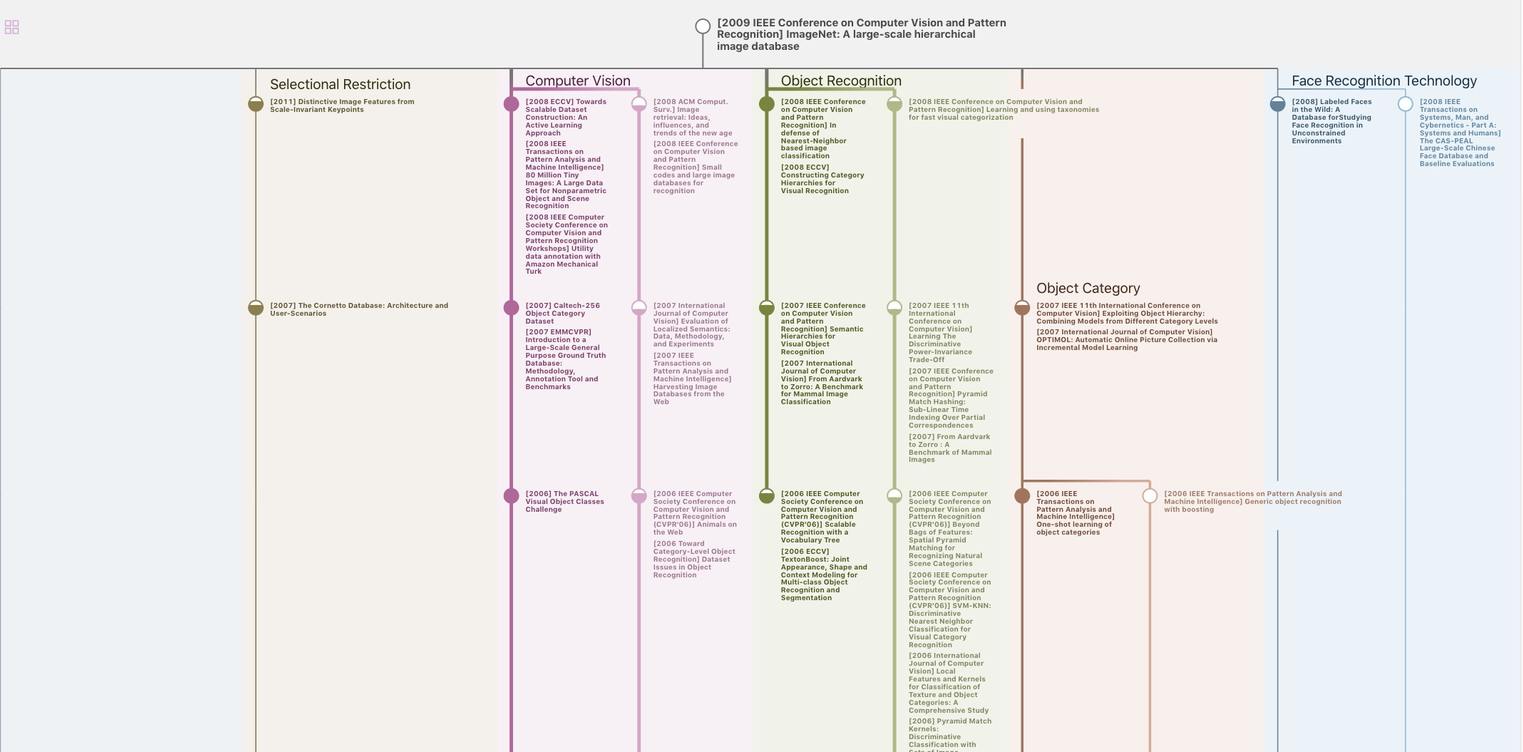
生成溯源树,研究论文发展脉络
Chat Paper
正在生成论文摘要