Embedded Coding of Point Cloud Attributes
IEEE signal processing letters(2024)
摘要
Point cloud compression (PCC) has been rapidly evolving in the context of international standards. Despite the inherent scalability of octree-based geometry descriptions, current attribute compression techniques prevent full scalability of compressed point clouds. We propose an improvement on an embedded attribute encoding method for point clouds based on set partitioning in hierarchical trees (SPIHT). We propose to use a multi-layer perceptron (MLP) to model contexts in order to further compress the final bit-stream. The encoder is used along with the region-adaptive hierarchical transform, which has been a popular transform for point cloud coding and is included in the standard geometry-based point cloud coder (G-PCC). The result is an encoder that is efficient, scalable, and, best of all, embedded. That is, higher compression is achieved by further trimming the single bit-stream. Experimental results show approximately 13% BD-Rate reduction using MLP-based context modeling.
更多查看译文
关键词
Embedded coding,multi-layer perceptron,point cloud compression,SPIHT
AI 理解论文
溯源树
样例
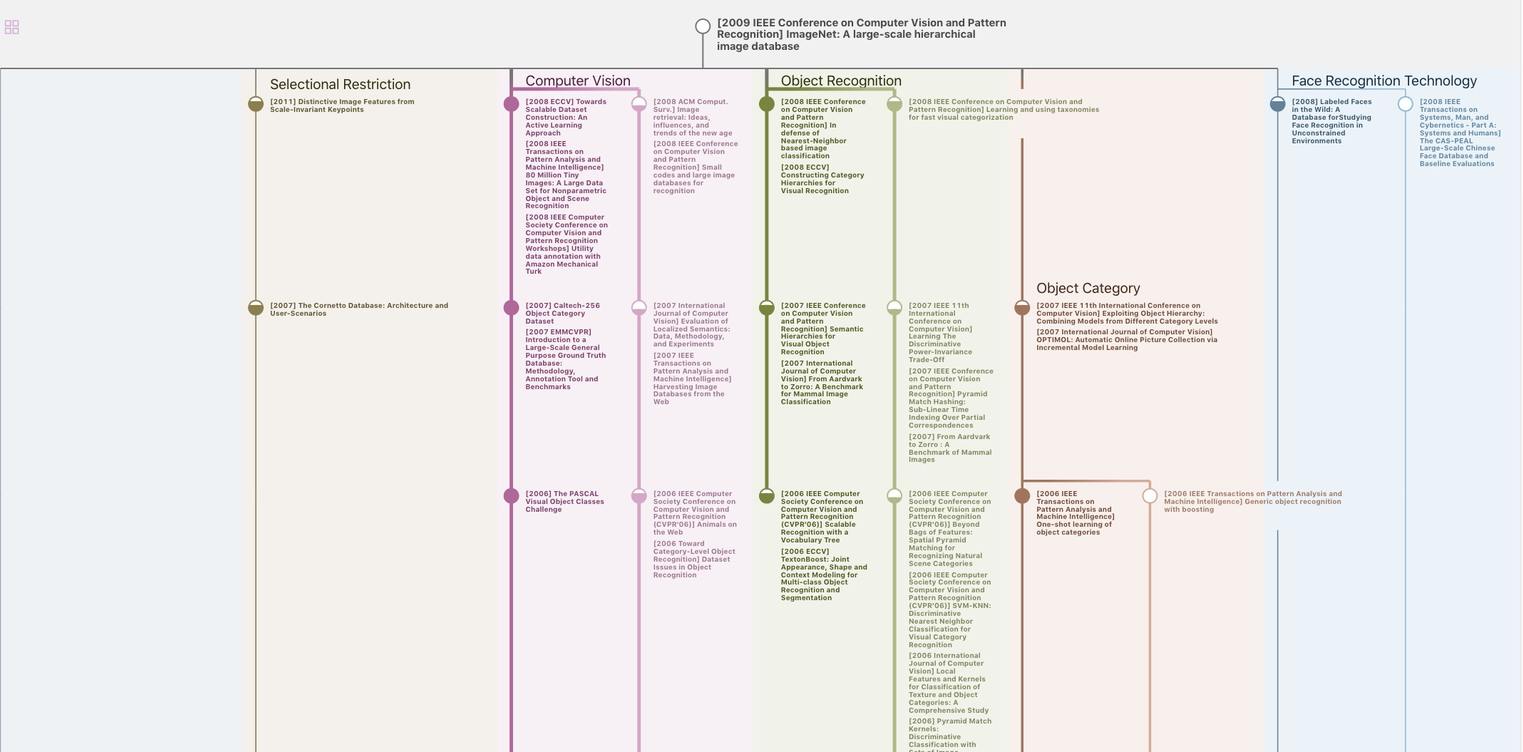
生成溯源树,研究论文发展脉络
Chat Paper
正在生成论文摘要