Robust Decoding of the Auditory Attention from EEG Recordings Through Graph Convolutional Networks
ICASSP 2024 - 2024 IEEE International Conference on Acoustics, Speech and Signal Processing (ICASSP)(2024)
摘要
Auditory attention decoding (AAD) with electroencephalography (EEG) holds great promise in brain-computer interface (BCI). Despite much progress, it remains a research topic on how to effectively evaluate the performance of EEG-based AAD algorithms under an appropriate setting that reflects the use scenarios. It is desired that systems are evaluated under cross-subject and cross-trial settings. However, systems are often reported under same-subject, same-trial settings, where test data are not truly separated from the training data, thus potentially leading to model overfitting due to data leakage. In this paper, we study the robustness of graph convolutional network (GCN), a novel approach to learning the intricate spatial patterns in multi-channel EEG signals, by comparing GCN across cross-subject, cross-trial, and same-subject, same-trial settings. On two publicly available AAD datasets, it is found that GCN exhibits remarkable robustness, outperforming previous conventional convolutional neural network (CNN) solutions. We confirm the superiority of our GCN-based AAD model in terms of generalization and robustness.
更多查看译文
关键词
Auditory attention,convolutional neural network,graph convolutional network,robustness decoding
AI 理解论文
溯源树
样例
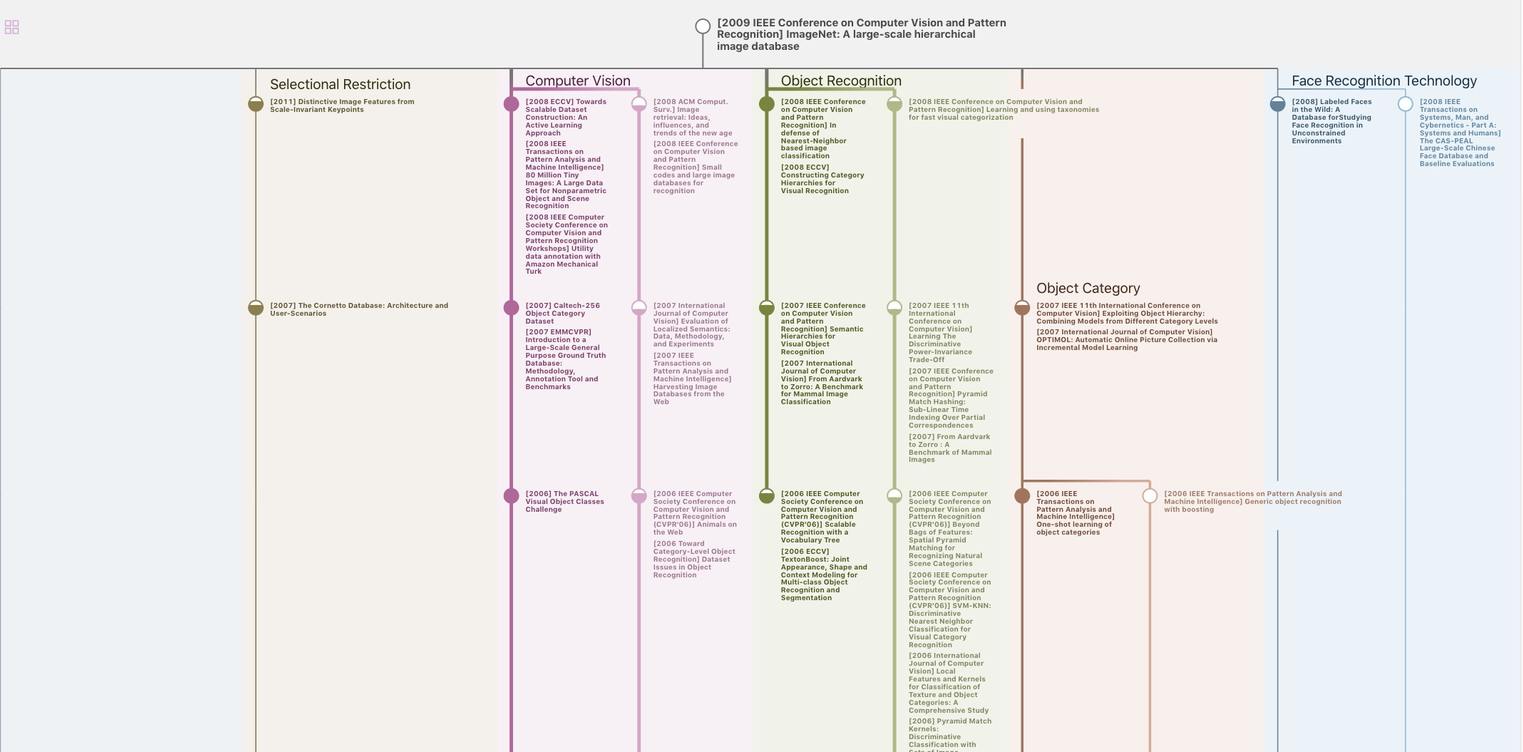
生成溯源树,研究论文发展脉络
Chat Paper
正在生成论文摘要