AdaFL: Adaptive Client Selection and Dynamic Contribution Evaluation for Efficient Federated Learning.
IEEE International Conference on Acoustics, Speech, and Signal Processing(2024)
摘要
Federated learning is a collaborative machine learning framework where multiple clients jointly train a global model. To mitigate communication overhead, it is common to select a subset of clients for participation in each training round. However, existing client selection strategies often rely on a fixed number of clients throughout all rounds, which may not be the optimal choice for balancing training efficiency and model performance. Moreover, these approaches typically evaluate clients solely based on their performances in one single round, neglecting the effects of historical records and potentially introducing randomness into the global model. In our work, we introduce AdaFL, a novel approach to client selection and contribution evaluation for efficient federated learning. AdaFL dynamically adjusts the number of clients to be selected using a piecewise function. It initiates with a small selection size to reduce communication overhead and progressively increases it to enhance model generalization. Furthermore, AdaFL evaluates clients’ contributions by combining their performance metrics from both current and historical rounds through a weighted average function, with a weight parameter fine-tuning the trade-off between current and historical data. Experimental results show that the proposed AdaFL outperforms prior works in terms of improving test accuracy and reducing training runtime.
更多查看译文
关键词
Federated Learning,Client Selection,Contribution Evaluation,Adaptive Strategy
AI 理解论文
溯源树
样例
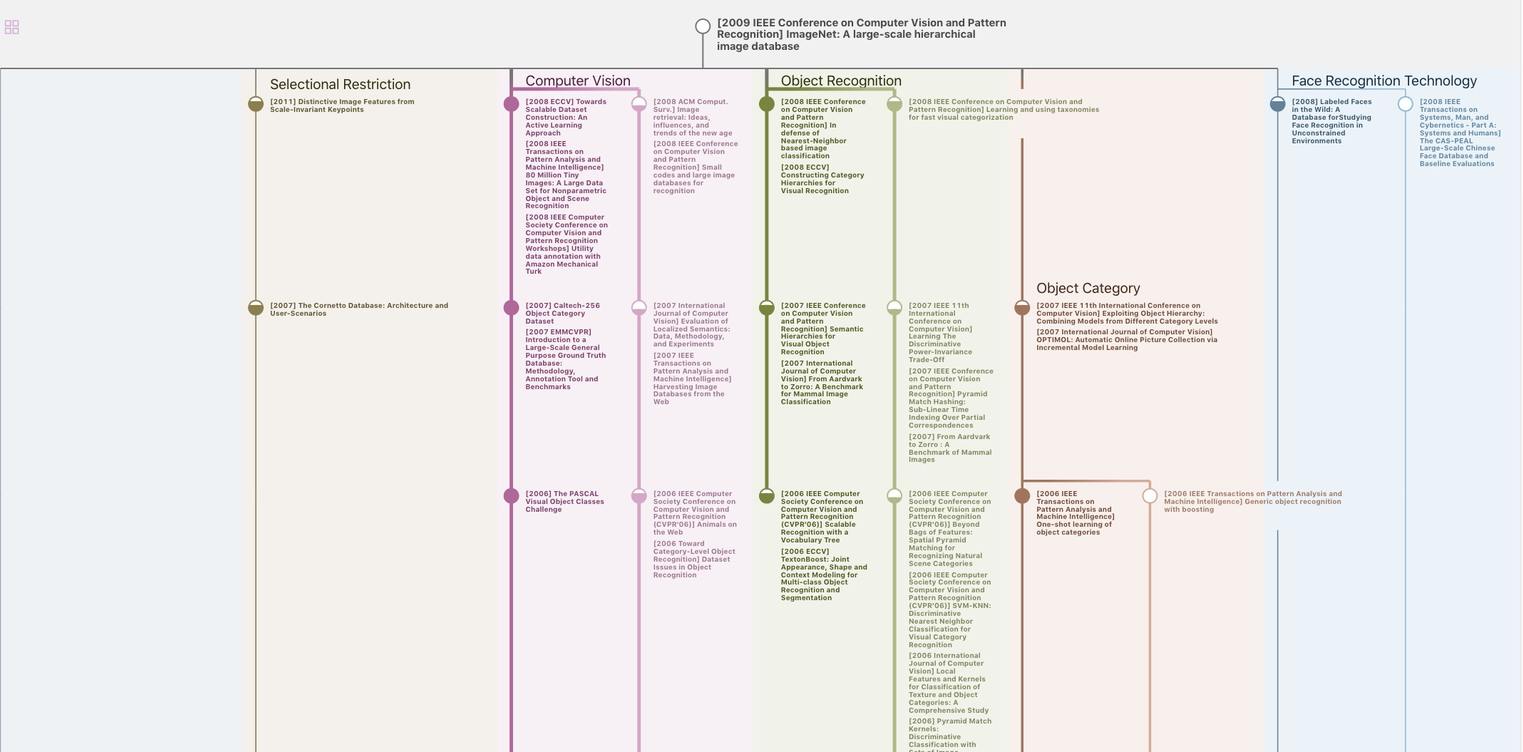
生成溯源树,研究论文发展脉络
Chat Paper
正在生成论文摘要